MIA-DPO: Multi-Image Augmented Direct Preference Optimization For Large Vision-Language Models
Ziyu Liu, Yuhang Zang, Xiaoyi Dong, Pan Zhang, Yuhang Cao, Haodong Duan, Conghui He, Yuanjun Xiong, Dahua Lin, Jiaqi Wang
2024-10-24
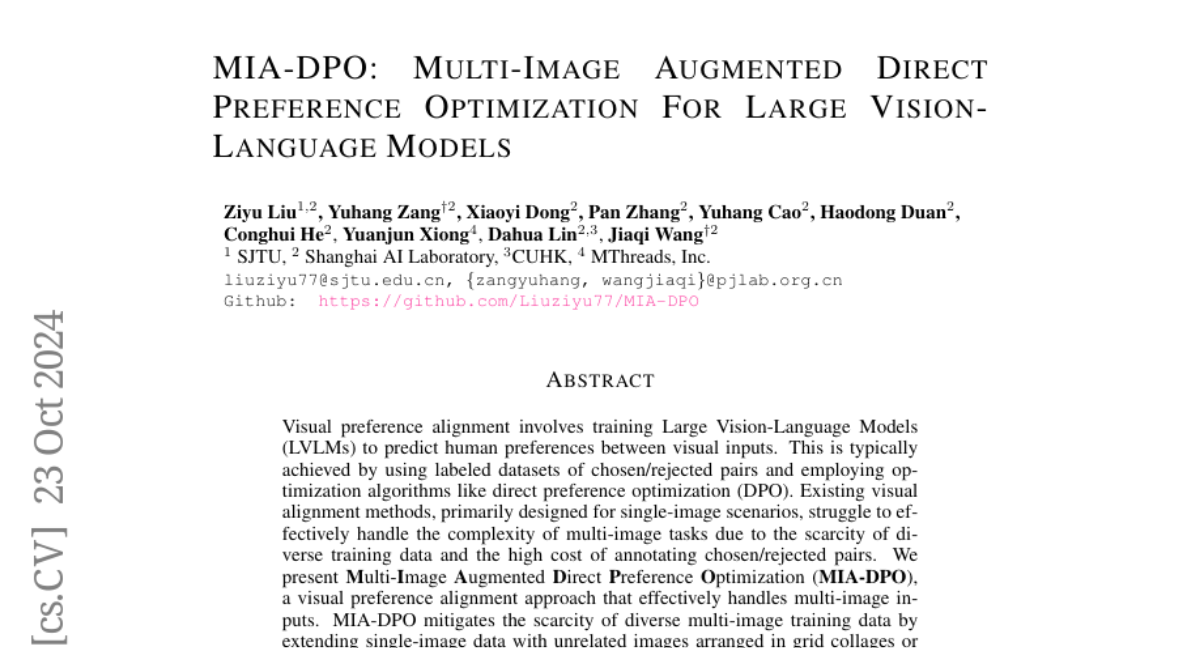
Summary
This paper introduces MIA-DPO, a new method for training Large Vision-Language Models (LVLMs) to better understand and predict human preferences when looking at multiple images.
What's the problem?
Current methods for aligning visual preferences in LVLMs often focus on single images and struggle with multi-image tasks. This is mainly because there isn't enough diverse training data available, and collecting data for multi-image scenarios can be expensive and time-consuming.
What's the solution?
MIA-DPO addresses these challenges by using a clever technique that combines single-image data with unrelated images arranged in creative formats, like grid collages. This approach reduces the need for extensive human annotations while still allowing the model to learn effectively. The method also uses attention values from the model to filter out incorrect responses, ensuring that the model learns from the right examples without needing extra data or human input.
Why it matters?
This research is important because it improves how LVLMs can analyze and respond to multiple images, making them more effective for real-world applications like image recognition and understanding complex visual information. By enhancing these models' performance, we can create AI systems that better align with human preferences and needs.
Abstract
Visual preference alignment involves training Large Vision-Language Models (LVLMs) to predict human preferences between visual inputs. This is typically achieved by using labeled datasets of chosen/rejected pairs and employing optimization algorithms like direct preference optimization (DPO). Existing visual alignment methods, primarily designed for single-image scenarios, struggle to effectively handle the complexity of multi-image tasks due to the scarcity of diverse training data and the high cost of annotating chosen/rejected pairs. We present Multi-Image Augmented Direct Preference Optimization (MIA-DPO), a visual preference alignment approach that effectively handles multi-image inputs. MIA-DPO mitigates the scarcity of diverse multi-image training data by extending single-image data with unrelated images arranged in grid collages or pic-in-pic formats, significantly reducing the costs associated with multi-image data annotations. Our observation reveals that attention values of LVLMs vary considerably across different images. We use attention values to identify and filter out rejected responses the model may have mistakenly focused on. Our attention-aware selection for constructing the chosen/rejected pairs without relying on (i) human annotation, (ii) extra data, and (iii) external models or APIs. MIA-DPO is compatible with various architectures and outperforms existing methods on five multi-image benchmarks, achieving an average performance boost of 3.0% on LLaVA-v1.5 and 4.3% on the recent InternLM-XC2.5. Moreover, MIA-DPO has a minimal effect on the model's ability to understand single images.