MindSearch: Mimicking Human Minds Elicits Deep AI Searcher
Zehui Chen, Kuikun Liu, Qiuchen Wang, Jiangning Liu, Wenwei Zhang, Kai Chen, Feng Zhao
2024-07-30
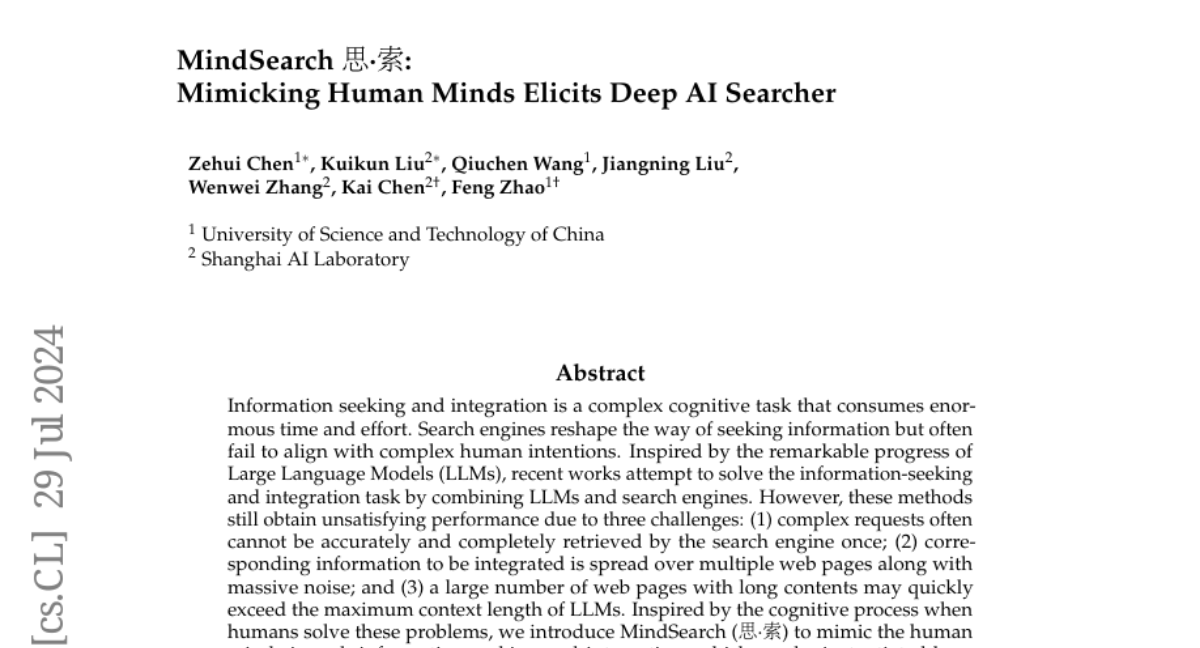
Summary
This paper discusses MindSearch, a new system designed to improve how AI searches for and integrates information from the web. It mimics the way humans think and solve problems when looking for information.
What's the problem?
Finding accurate information online can be difficult, especially when requests are complex. Current methods often struggle because they can’t retrieve all the needed information at once, and relevant data is usually scattered across many web pages, making it hard to piece everything together. Additionally, long web pages can exceed the limits of what AI models can process at one time.
What's the solution?
To tackle these challenges, MindSearch uses a multi-agent framework that simulates human thinking. It breaks down a user's question into smaller parts (sub-questions) and organizes them like a graph. The system has two main components: the WebPlanner, which decides how to approach the search by creating this graph, and the WebSearcher, which collects information from various web pages for each sub-question. This allows MindSearch to efficiently gather and integrate information from over 300 web pages in just three minutes, which would take a human about three hours.
Why it matters?
This research is important because it enhances the ability of AI systems to search for and understand complex information more effectively. By mimicking human thought processes, MindSearch can provide deeper and broader answers to user queries, making it a valuable tool for anyone looking for reliable information online. This could lead to better AI search engines that help users find what they need more quickly and accurately.
Abstract
Information seeking and integration is a complex cognitive task that consumes enormous time and effort. Inspired by the remarkable progress of Large Language Models, recent works attempt to solve this task by combining LLMs and search engines. However, these methods still obtain unsatisfying performance due to three challenges: (1) complex requests often cannot be accurately and completely retrieved by the search engine once (2) corresponding information to be integrated is spread over multiple web pages along with massive noise, and (3) a large number of web pages with long contents may quickly exceed the maximum context length of LLMs. Inspired by the cognitive process when humans solve these problems, we introduce MindSearch to mimic the human minds in web information seeking and integration, which can be instantiated by a simple yet effective LLM-based multi-agent framework. The WebPlanner models the human mind of multi-step information seeking as a dynamic graph construction process: it decomposes the user query into atomic sub-questions as nodes in the graph and progressively extends the graph based on the search result from WebSearcher. Tasked with each sub-question, WebSearcher performs hierarchical information retrieval with search engines and collects valuable information for WebPlanner. The multi-agent design of MindSearch enables the whole framework to seek and integrate information parallelly from larger-scale (e.g., more than 300) web pages in 3 minutes, which is worth 3 hours of human effort. MindSearch demonstrates significant improvement in the response quality in terms of depth and breadth, on both close-set and open-set QA problems. Besides, responses from MindSearch based on InternLM2.5-7B are preferable by humans to ChatGPT-Web and Perplexity.ai applications, which implies that MindSearch can already deliver a competitive solution to the proprietary AI search engine.