Minimum Entropy Coupling with Bottleneck
M. Reza Ebrahimi, Jun Chen, Ashish Khisti
2024-11-01
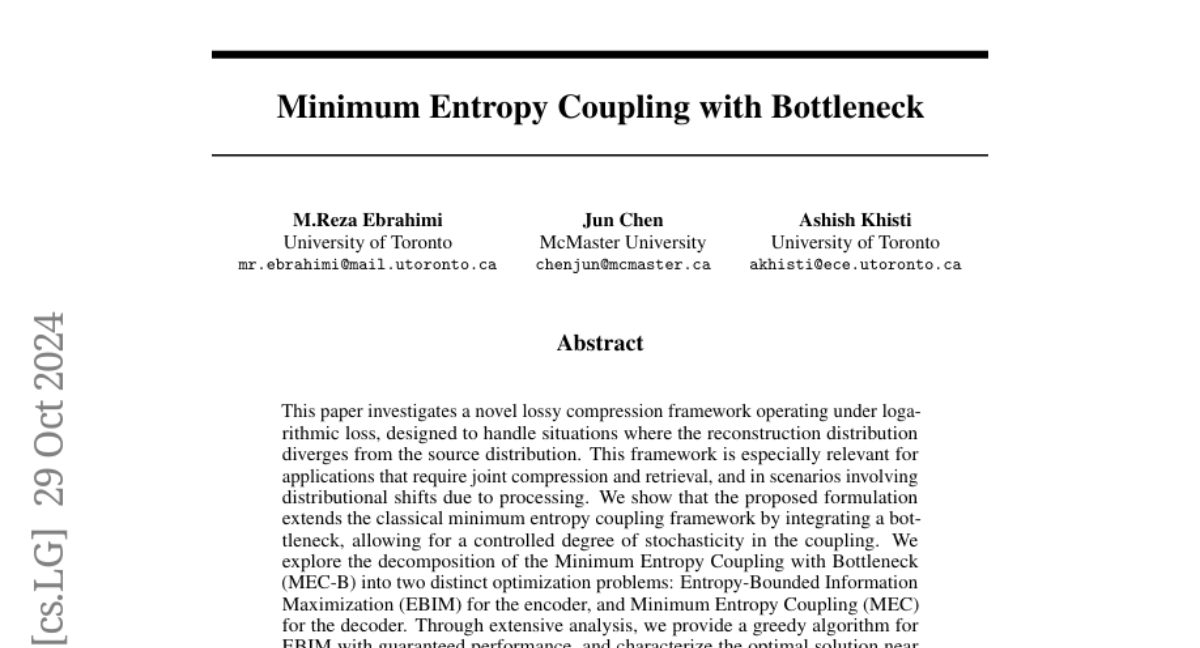
Summary
This paper discusses a new method called Minimum Entropy Coupling with Bottleneck (MEC-B) for compressing data while still allowing for some loss of information. This method is useful for situations where the data being reconstructed is different from the original data, especially in tasks that involve both compressing and retrieving information.
What's the problem?
When compressing data, especially in cases where the original and reconstructed data distributions differ, existing methods struggle to maintain quality and efficiency. This makes it hard to effectively compress and retrieve information, particularly in complex scenarios like communication systems.
What's the solution?
The authors propose MEC-B, which improves on traditional methods by adding a 'bottleneck' that controls how much randomness is allowed during the compression process. They break down this method into two parts: one focuses on maximizing the information captured by the encoder (the part that compresses the data), and the other ensures minimal entropy during decoding (the part that retrieves the data). They also develop a greedy algorithm to optimize this process and test it in practical scenarios using Markov Coding Games.
Why it matters?
This research is significant because it provides a more effective way to handle data compression and retrieval, which is crucial for various applications like communication systems and data storage. By improving how we can compress information while still retrieving it accurately, this method could enhance performance in many real-world technologies.
Abstract
This paper investigates a novel lossy compression framework operating under logarithmic loss, designed to handle situations where the reconstruction distribution diverges from the source distribution. This framework is especially relevant for applications that require joint compression and retrieval, and in scenarios involving distributional shifts due to processing. We show that the proposed formulation extends the classical minimum entropy coupling framework by integrating a bottleneck, allowing for a controlled degree of stochasticity in the coupling. We explore the decomposition of the Minimum Entropy Coupling with Bottleneck (MEC-B) into two distinct optimization problems: Entropy-Bounded Information Maximization (EBIM) for the encoder, and Minimum Entropy Coupling (MEC) for the decoder. Through extensive analysis, we provide a greedy algorithm for EBIM with guaranteed performance, and characterize the optimal solution near functional mappings, yielding significant theoretical insights into the structural complexity of this problem. Furthermore, we illustrate the practical application of MEC-B through experiments in Markov Coding Games (MCGs) under rate limits. These games simulate a communication scenario within a Markov Decision Process, where an agent must transmit a compressed message from a sender to a receiver through its actions. Our experiments highlight the trade-offs between MDP rewards and receiver accuracy across various compression rates, showcasing the efficacy of our method compared to conventional compression baseline.