MIO: A Foundation Model on Multimodal Tokens
Zekun Wang, King Zhu, Chunpu Xu, Wangchunshu Zhou, Jiaheng Liu, Yibo Zhang, Jiashuo Wang, Ning Shi, Siyu Li, Yizhi Li, Haoran Que, Zhaoxiang Zhang, Yuanxing Zhang, Ge Zhang, Ke Xu, Jie Fu, Wenhao Huang
2024-09-30
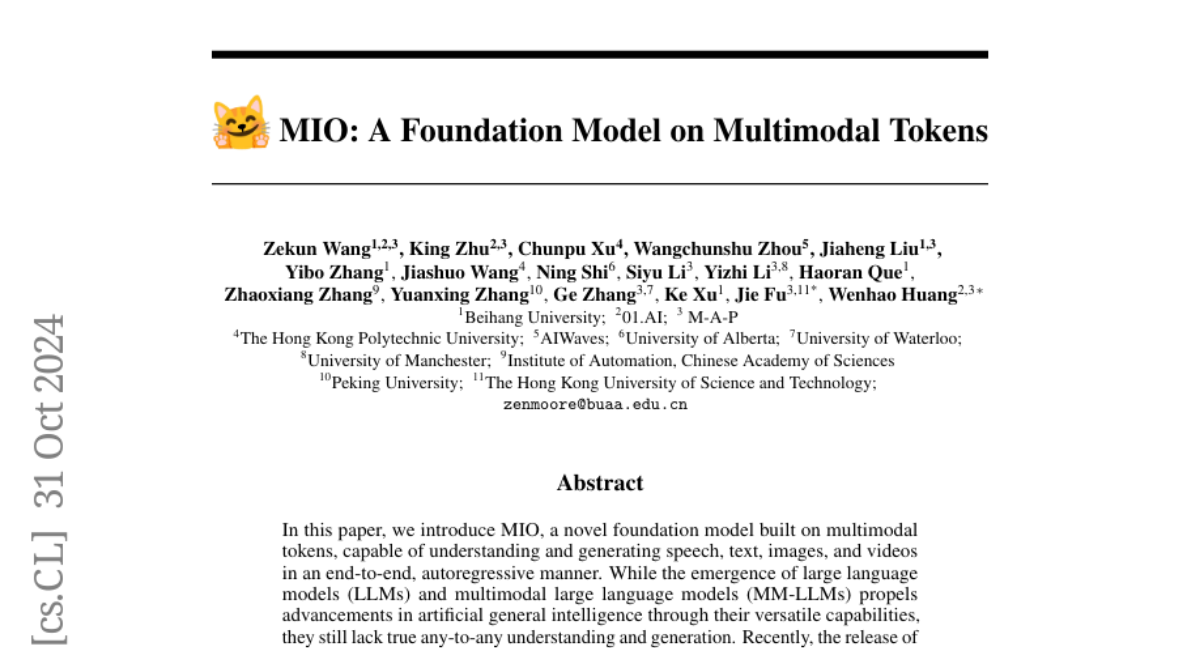
Summary
This paper talks about MIO, a new foundation model designed to understand and generate different types of data, including speech, text, images, and videos. It aims to improve how machines interact with various forms of information in a seamless way.
What's the problem?
While existing models like large language models (LLMs) can handle multiple types of data, they often struggle with truly understanding and generating content across all these formats at the same time. Many current systems are either closed-source or limited in their capabilities, making it difficult to create complex outputs that involve mixing different types of media, like combining text with images or videos.
What's the solution?
MIO addresses these challenges by using a method called causal multimodal modeling, which allows it to learn from a wide range of data types simultaneously. The model undergoes a four-stage training process that includes aligning different types of data, interleaving them for better integration, enhancing speech capabilities, and fine-tuning on various tasks. This approach enables MIO to generate interleaved sequences of text and video and perform complex reasoning tasks effectively.
Why it matters?
This research is important because it represents a significant advancement in creating AI systems that can understand and generate multiple forms of media simultaneously. By improving the ability to mix and match different types of content, MIO could enhance applications in areas like content creation, education, and entertainment, making interactions with technology more intuitive and versatile.
Abstract
In this paper, we introduce MIO, a novel foundation model built on multimodal tokens, capable of understanding and generating speech, text, images, and videos in an end-to-end, autoregressive manner. While the emergence of large language models (LLMs) and multimodal large language models (MM-LLMs) propels advancements in artificial general intelligence through their versatile capabilities, they still lack true any-to-any understanding and generation. Recently, the release of GPT-4o has showcased the remarkable potential of any-to-any LLMs for complex real-world tasks, enabling omnidirectional input and output across images, speech, and text. However, it is closed-source and does not support the generation of multimodal interleaved sequences. To address this gap, we present MIO, which is trained on a mixture of discrete tokens across four modalities using causal multimodal modeling. MIO undergoes a four-stage training process: (1) alignment pre-training, (2) interleaved pre-training, (3) speech-enhanced pre-training, and (4) comprehensive supervised fine-tuning on diverse textual, visual, and speech tasks. Our experimental results indicate that MIO exhibits competitive, and in some cases superior, performance compared to previous dual-modal baselines, any-to-any model baselines, and even modality-specific baselines. Moreover, MIO demonstrates advanced capabilities inherent to its any-to-any feature, such as interleaved video-text generation, chain-of-visual-thought reasoning, visual guideline generation, instructional image editing, etc.