MiRAGeNews: Multimodal Realistic AI-Generated News Detection
Runsheng Huang, Liam Dugan, Yue Yang, Chris Callison-Burch
2024-10-14
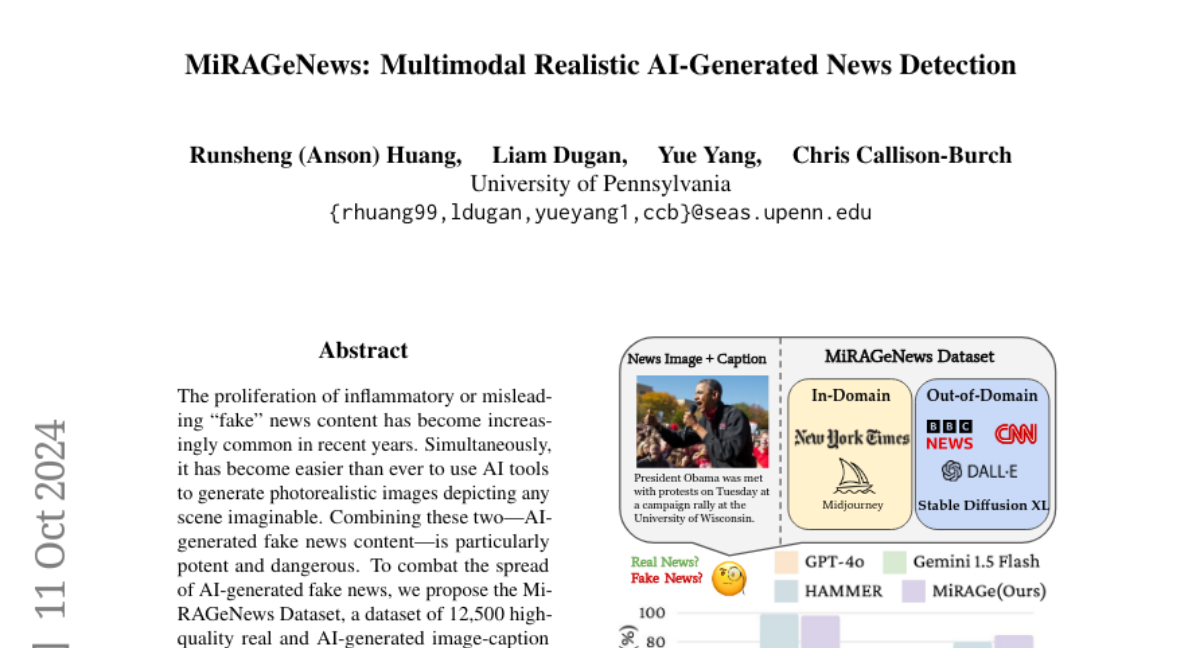
Summary
This paper presents MiRAGeNews, a dataset and framework designed to detect AI-generated fake news by analyzing both images and captions to identify misleading content.
What's the problem?
The rise of fake news, especially AI-generated content that looks very realistic, poses a significant threat to accurate information online. As AI tools become more sophisticated at creating convincing news articles and images, it becomes harder for people to tell what is real and what is not. This makes it crucial to develop effective methods for detecting such misleading content.
What's the solution?
To tackle this issue, the researchers created the MiRAGeNews Dataset, which includes 12,500 pairs of real and AI-generated image-caption combinations. They then trained a multi-modal detector called MiRAGe that uses both text and images to identify whether news content is genuine or generated by AI. Their experiments showed that MiRAGe outperformed existing methods in detecting fake news, improving accuracy significantly.
Why it matters?
This research is important because it helps combat the spread of misinformation by providing tools and datasets that can improve the detection of AI-generated fake news. As misinformation continues to grow online, having reliable detection systems is essential for maintaining trust in media and information sources.
Abstract
The proliferation of inflammatory or misleading "fake" news content has become increasingly common in recent years. Simultaneously, it has become easier than ever to use AI tools to generate photorealistic images depicting any scene imaginable. Combining these two -- AI-generated fake news content -- is particularly potent and dangerous. To combat the spread of AI-generated fake news, we propose the MiRAGeNews Dataset, a dataset of 12,500 high-quality real and AI-generated image-caption pairs from state-of-the-art generators. We find that our dataset poses a significant challenge to humans (60% F-1) and state-of-the-art multi-modal LLMs (< 24% F-1). Using our dataset we train a multi-modal detector (MiRAGe) that improves by +5.1% F-1 over state-of-the-art baselines on image-caption pairs from out-of-domain image generators and news publishers. We release our code and data to aid future work on detecting AI-generated content.