MIRAI: Evaluating LLM Agents for Event Forecasting
Chenchen Ye, Ziniu Hu, Yihe Deng, Zijie Huang, Mingyu Derek Ma, Yanqiao Zhu, Wei Wang
2024-07-02
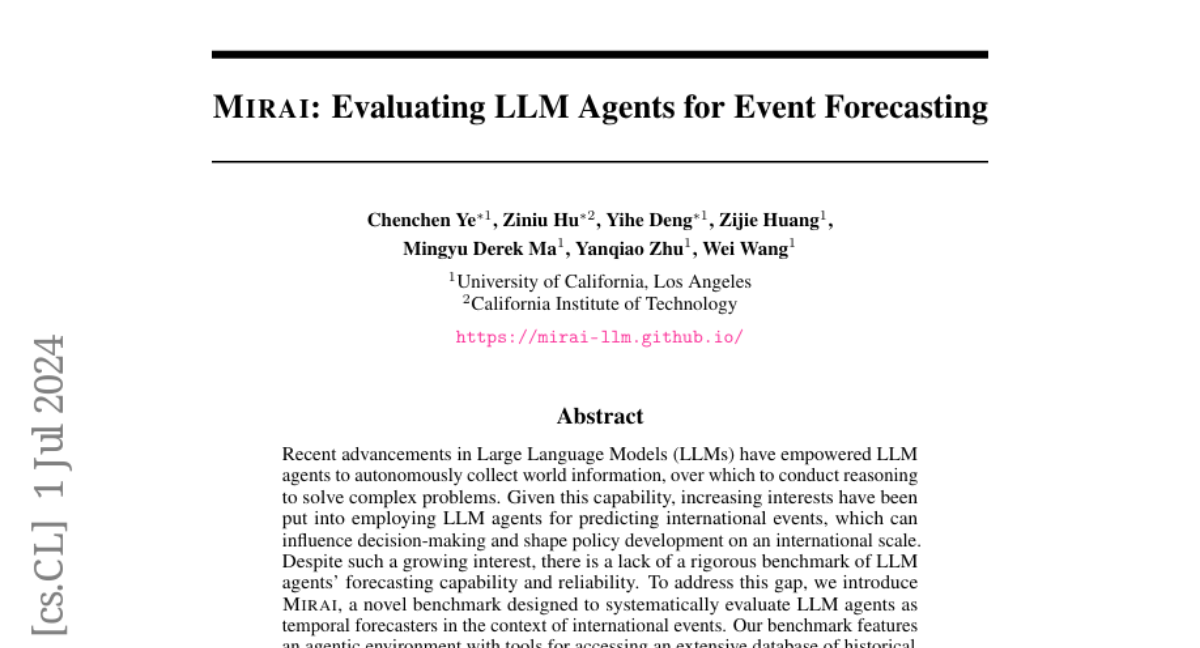
Summary
This paper talks about MIRAI, a new benchmark designed to evaluate how well large language model (LLM) agents can predict international events. It focuses on creating a system that tests these models' abilities to gather information and make accurate forecasts.
What's the problem?
As LLMs have advanced, there has been a growing interest in using them to predict future international events, which can impact important decisions and policies. However, there hasn't been a proper way to measure how good these models are at forecasting, making it difficult to know which models perform best and under what conditions.
What's the solution?
To address this issue, the authors developed MIRAI, which provides a structured environment for testing LLM agents. This benchmark allows the models to access a large database of historical events and news articles. The authors refined an existing database (GDELT) and created various tasks that require the models to make predictions over different time frames. They also included tools that let the agents write code to gather and analyze data effectively. This comprehensive approach helps evaluate how well the agents can source information, use tools, and reason based on historical data to predict future events.
Why it matters?
This research is important because it establishes a reliable way to assess the forecasting abilities of LLM agents. By creating MIRAI, the authors contribute to improving the accuracy and reliability of models used in international relations analysis. This can help policymakers and researchers make better-informed decisions based on accurate predictions of future events.
Abstract
Recent advancements in Large Language Models (LLMs) have empowered LLM agents to autonomously collect world information, over which to conduct reasoning to solve complex problems. Given this capability, increasing interests have been put into employing LLM agents for predicting international events, which can influence decision-making and shape policy development on an international scale. Despite such a growing interest, there is a lack of a rigorous benchmark of LLM agents' forecasting capability and reliability. To address this gap, we introduce MIRAI, a novel benchmark designed to systematically evaluate LLM agents as temporal forecasters in the context of international events. Our benchmark features an agentic environment with tools for accessing an extensive database of historical, structured events and textual news articles. We refine the GDELT event database with careful cleaning and parsing to curate a series of relational prediction tasks with varying forecasting horizons, assessing LLM agents' abilities from short-term to long-term forecasting. We further implement APIs to enable LLM agents to utilize different tools via a code-based interface. In summary, MIRAI comprehensively evaluates the agents' capabilities in three dimensions: 1) autonomously source and integrate critical information from large global databases; 2) write codes using domain-specific APIs and libraries for tool-use; and 3) jointly reason over historical knowledge from diverse formats and time to accurately predict future events. Through comprehensive benchmarking, we aim to establish a reliable framework for assessing the capabilities of LLM agents in forecasting international events, thereby contributing to the development of more accurate and trustworthy models for international relation analysis.