MIT-10M: A Large Scale Parallel Corpus of Multilingual Image Translation
Bo Li, Shaolin Zhu, Lijie Wen
2024-12-12
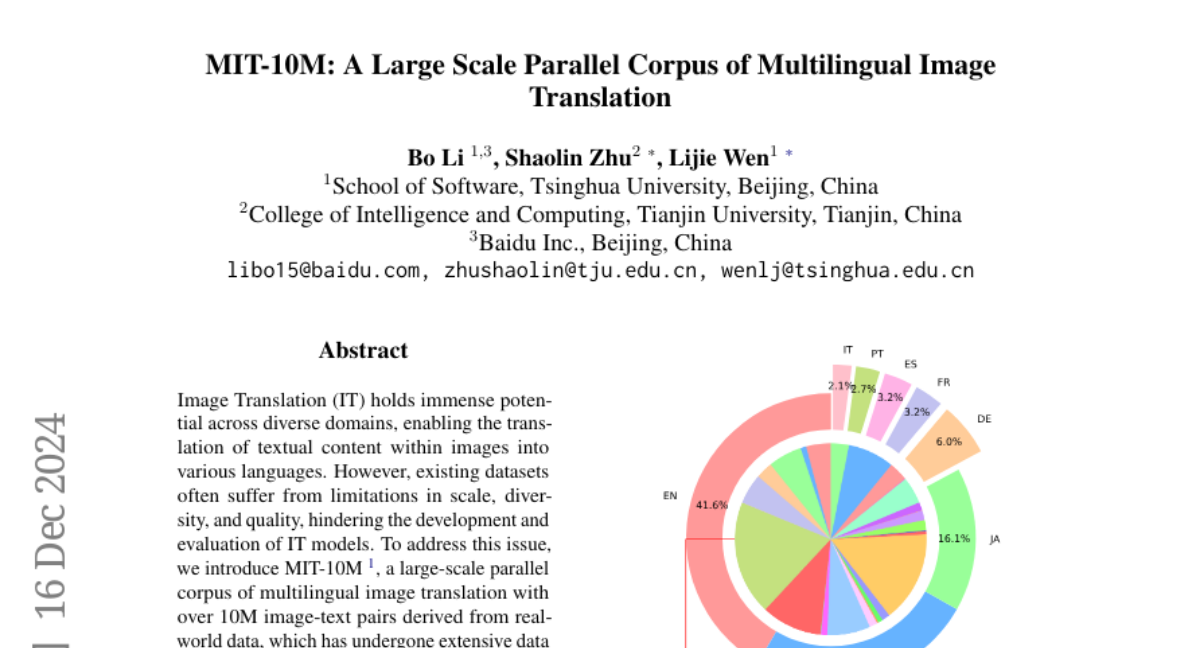
Summary
This paper introduces MIT-10M, a massive dataset designed for translating text in images into multiple languages. It contains over 10 million image-text pairs that help improve the training of AI models for image translation.
What's the problem?
Existing datasets for image translation often lack enough size, variety, and quality, which makes it hard to train AI models effectively. These limitations prevent the development of robust models that can handle real-world tasks where images contain text that needs to be translated into different languages.
What's the solution?
MIT-10M addresses these issues by providing a large-scale collection of over 10 million image-text pairs, derived from real-world sources. The dataset includes 840,000 images across 14 languages and various categories and difficulty levels. The data has been cleaned and validated to ensure high quality, allowing AI models to learn better and perform well in translating text within images.
Why it matters?
This dataset is significant because it enhances the ability of AI systems to understand and translate text in images accurately. By providing a rich and diverse set of data, MIT-10M helps improve the performance of translation models, making them more effective in real-world applications like translating signs, labels, and other text found in images.
Abstract
Image Translation (IT) holds immense potential across diverse domains, enabling the translation of textual content within images into various languages. However, existing datasets often suffer from limitations in scale, diversity, and quality, hindering the development and evaluation of IT models. To address this issue, we introduce MIT-10M, a large-scale parallel corpus of multilingual image translation with over 10M image-text pairs derived from real-world data, which has undergone extensive data cleaning and multilingual translation validation. It contains 840K images in three sizes, 28 categories, tasks with three levels of difficulty and 14 languages image-text pairs, which is a considerable improvement on existing datasets. We conduct extensive experiments to evaluate and train models on MIT-10M. The experimental results clearly indicate that our dataset has higher adaptability when it comes to evaluating the performance of the models in tackling challenging and complex image translation tasks in the real world. Moreover, the performance of the model fine-tuned with MIT-10M has tripled compared to the baseline model, further confirming its superiority.