Mitigating Object Hallucination via Concentric Causal Attention
Yun Xing, Yiheng Li, Ivan Laptev, Shijian Lu
2024-10-23
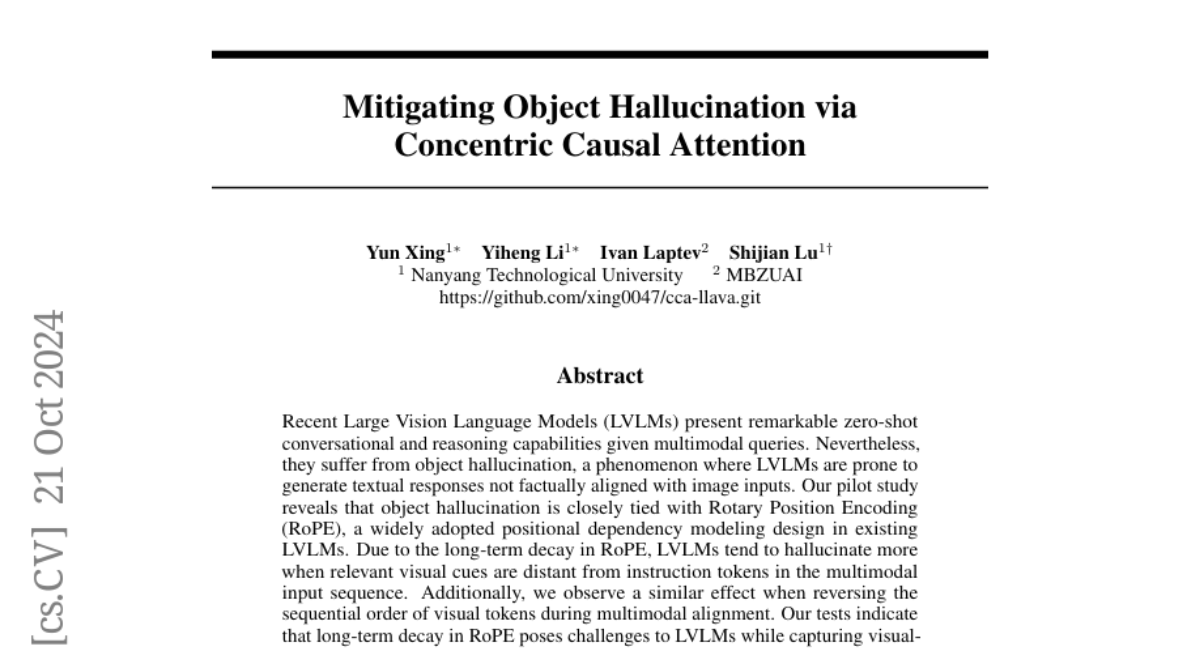
Summary
This paper discusses a new method called Concentric Causal Attention (CCA) that helps improve how large vision-language models (LVLMs) understand and respond to images and text by reducing a problem known as object hallucination.
What's the problem?
Object hallucination occurs when LVLMs create responses that don't match the images they are analyzing. This happens because the models struggle to connect visual information with text instructions, especially when the relevant parts of the image are far away from the text in the input sequence.
What's the solution?
The researchers found that a technique called Rotary Position Encoding (RoPE) contributes to this problem because it causes important visual information to lose its relevance over distance. To fix this, they introduced CCA, which adjusts how visual and text tokens interact, making it easier for the model to understand their relationships. This reduces the distance between relevant tokens, improving the model's ability to accurately respond to multimodal queries.
Why it matters?
Improving how LVLMs handle object hallucination is crucial because it enhances their reliability and accuracy in real-world applications, such as image captioning and visual question answering. A better understanding of images leads to more trustworthy AI systems that can be used in various fields like education, healthcare, and entertainment.
Abstract
Recent Large Vision Language Models (LVLMs) present remarkable zero-shot conversational and reasoning capabilities given multimodal queries. Nevertheless, they suffer from object hallucination, a phenomenon where LVLMs are prone to generate textual responses not factually aligned with image inputs. Our pilot study reveals that object hallucination is closely tied with Rotary Position Encoding (RoPE), a widely adopted positional dependency modeling design in existing LVLMs. Due to the long-term decay in RoPE, LVLMs tend to hallucinate more when relevant visual cues are distant from instruction tokens in the multimodal input sequence. Additionally, we observe a similar effect when reversing the sequential order of visual tokens during multimodal alignment. Our tests indicate that long-term decay in RoPE poses challenges to LVLMs while capturing visual-instruction interactions across long distances. We propose Concentric Causal Attention (CCA), a simple yet effective positional alignment strategy that mitigates the impact of RoPE long-term decay in LVLMs by naturally reducing relative distance between visual and instruction tokens. With CCA, visual tokens can better interact with instruction tokens, thereby enhancing model's perception capability and alleviating object hallucination. Without bells and whistles, our positional alignment method surpasses existing hallucination mitigation strategies by large margins on multiple object hallucination benchmarks.