MIVE: New Design and Benchmark for Multi-Instance Video Editing
Samuel Teodoro, Agus Gunawan, Soo Ye Kim, Jihyong Oh, Munchurl Kim
2024-12-18
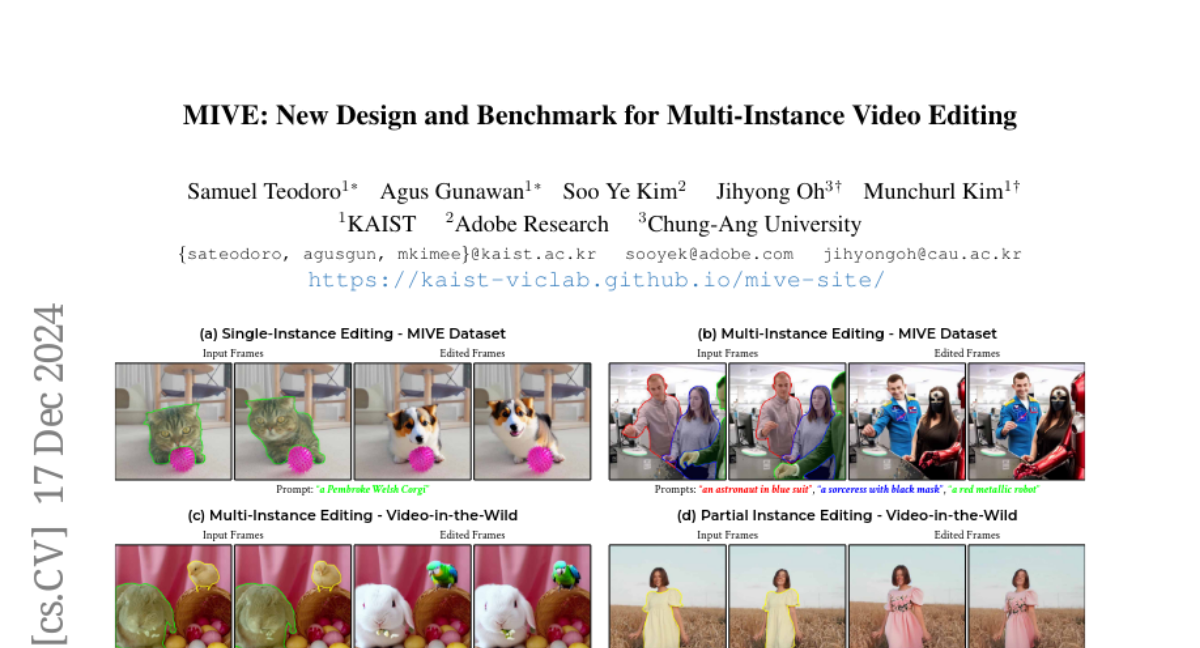
Summary
This paper talks about MIVE, a new framework for multi-instance video editing that allows users to edit multiple objects in a video accurately and efficiently using simple text prompts.
What's the problem?
Current AI video editing methods often struggle when users want to make localized edits to multiple objects in a video. These methods can lead to problems like unintentional changes in other parts of the video, inaccurate edits, and lack of proper evaluation tools to measure how well the edits are done. This makes it hard for users to achieve the desired results without affecting other elements in the video.
What's the solution?
MIVE introduces a zero-shot approach to multi-instance video editing, meaning it can edit videos without needing prior training on specific objects. It uses two main techniques: Disentangled Multi-instance Sampling (DMS) to prevent unwanted changes during editing and Instance-centric Probability Redistribution (IPR) to ensure accurate and faithful edits. Additionally, MIVE comes with a new dataset and a unique evaluation metric called the Cross-Instance Accuracy (CIA) Score to help assess how well the edits are made across different instances in a video.
Why it matters?
This research is important because it sets a new standard for how AI can be used in video editing, particularly when dealing with multiple objects. By improving the accuracy and reliability of edits, MIVE enables creators to produce high-quality videos more easily, which can benefit fields like filmmaking, marketing, and content creation.
Abstract
Recent AI-based video editing has enabled users to edit videos through simple text prompts, significantly simplifying the editing process. However, recent zero-shot video editing techniques primarily focus on global or single-object edits, which can lead to unintended changes in other parts of the video. When multiple objects require localized edits, existing methods face challenges, such as unfaithful editing, editing leakage, and lack of suitable evaluation datasets and metrics. To overcome these limitations, we propose a zero-shot Multi-Instance Video Editing framework, called MIVE. MIVE is a general-purpose mask-based framework, not dedicated to specific objects (e.g., people). MIVE introduces two key modules: (i) Disentangled Multi-instance Sampling (DMS) to prevent editing leakage and (ii) Instance-centric Probability Redistribution (IPR) to ensure precise localization and faithful editing. Additionally, we present our new MIVE Dataset featuring diverse video scenarios and introduce the Cross-Instance Accuracy (CIA) Score to evaluate editing leakage in multi-instance video editing tasks. Our extensive qualitative, quantitative, and user study evaluations demonstrate that MIVE significantly outperforms recent state-of-the-art methods in terms of editing faithfulness, accuracy, and leakage prevention, setting a new benchmark for multi-instance video editing. The project page is available at https://kaist-viclab.github.io/mive-site/