MM-IFEngine: Towards Multimodal Instruction Following
Shengyuan Ding, Shenxi Wu, Xiangyu Zhao, Yuhang Zang, Haodong Duan, Xiaoyi Dong, Pan Zhang, Yuhang Cao, Dahua Lin, Jiaqi Wang
2025-04-11
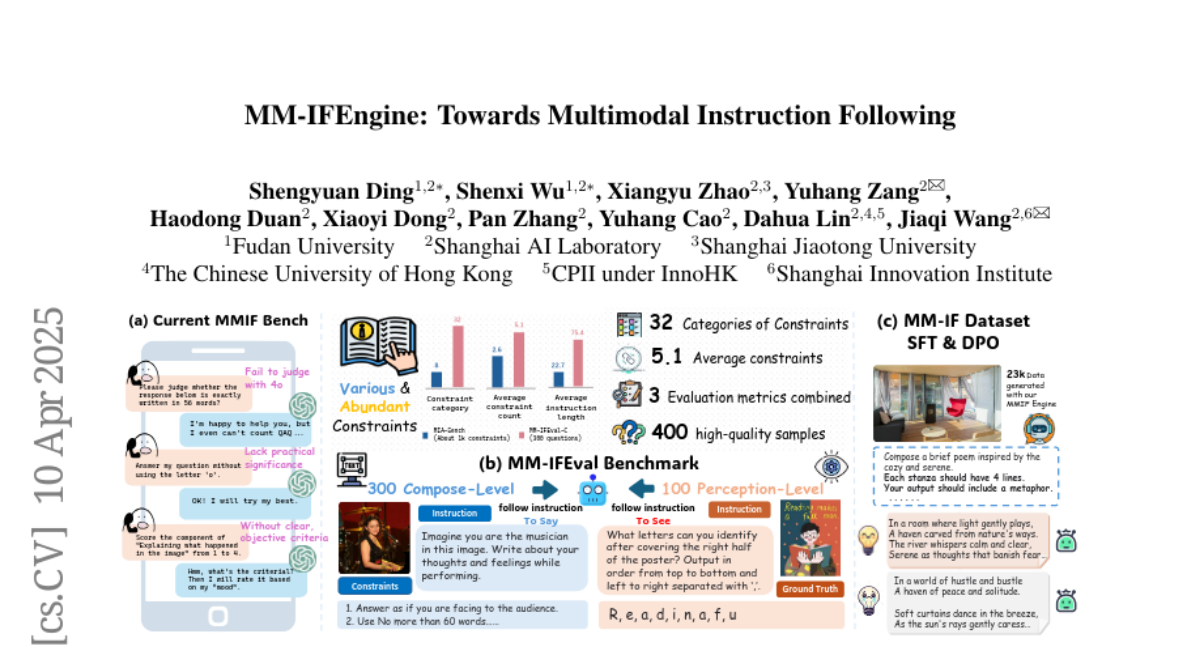
Summary
This paper talks about MM-IFEngine, a tool that helps AI better follow instructions involving both images and text, like making a cake by following a recipe with pictures, by improving how it learns and is tested.
What's the problem?
Current AI models struggle with complex tasks that mix images and text because they lack good training examples, use oversimplified tests, and can’t tell if mistakes come from misreading images or bad reasoning.
What's the solution?
MM-IFEngine creates realistic training examples (like recipe-image pairs), builds tougher tests that check both image understanding and reasoning, and uses feedback loops to teach AI to follow instructions more precisely.
Why it matters?
This improves AI assistants for tasks like cooking tutorials or assembly guides, where clear image-text understanding is crucial, and helps developers build smarter, more reliable models.
Abstract
The Instruction Following (IF) ability measures how well Multi-modal Large Language Models (MLLMs) understand exactly what users are telling them and whether they are doing it right. Existing multimodal instruction following training data is scarce, the benchmarks are simple with atomic instructions, and the evaluation strategies are imprecise for tasks demanding exact output constraints. To address this, we present MM-IFEngine, an effective pipeline to generate high-quality image-instruction pairs. Our MM-IFEngine pipeline yields large-scale, diverse, and high-quality training data MM-IFInstruct-23k, which is suitable for Supervised Fine-Tuning (SFT) and extended as MM-IFDPO-23k for Direct Preference Optimization (DPO). We further introduce MM-IFEval, a challenging and diverse multi-modal instruction-following benchmark that includes (1) both compose-level constraints for output responses and perception-level constraints tied to the input images, and (2) a comprehensive evaluation pipeline incorporating both rule-based assessment and judge model. We conduct SFT and DPO experiments and demonstrate that fine-tuning MLLMs on MM-IFInstruct-23k and MM-IFDPO-23k achieves notable gains on various IF benchmarks, such as MM-IFEval (+10.2%), MIA (+7.6%), and IFEval (+12.3%). The full data and evaluation code will be released on https://github.com/SYuan03/MM-IFEngine.