MMEvol: Empowering Multimodal Large Language Models with Evol-Instruct
Run Luo, Haonan Zhang, Longze Chen, Ting-En Lin, Xiong Liu, Yuchuan Wu, Min Yang, Minzheng Wang, Pengpeng Zeng, Lianli Gao, Heng Tao Shen, Yunshui Li, Xiaobo Xia, Fei Huang, Jingkuan Song, Yongbin Li
2024-09-10
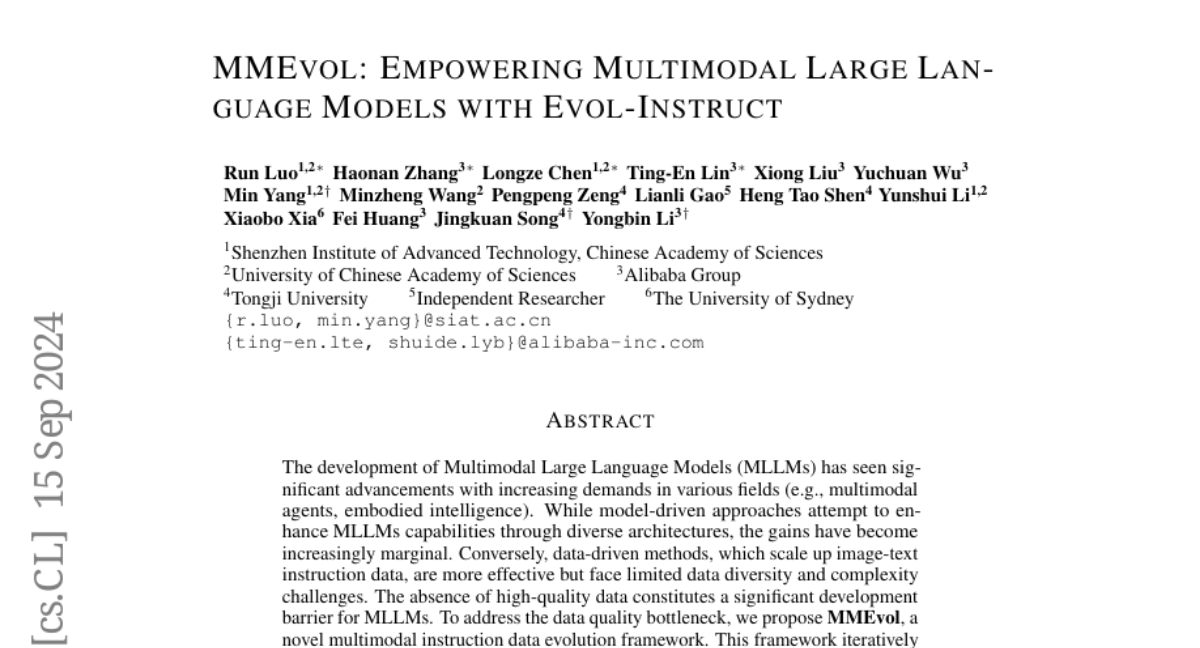
Summary
This paper talks about MMEvol, a new framework designed to improve the quality and diversity of instruction data for Multimodal Large Language Models (MLLMs), which can handle both text and images.
What's the problem?
One of the main challenges in developing MLLMs is the lack of high-quality and diverse instruction data. Creating this data manually takes a lot of time and effort, and using existing models often leads to simple instructions that don't help the models perform well. This limits the models' abilities to understand complex tasks involving images and text.
What's the solution?
To solve this problem, the authors propose MMEvol, which evolves instruction data through three main processes: fine-grained perception evolution, cognitive reasoning evolution, and interaction evolution. Starting with an initial set of instructions (SEED-163K), MMEvol systematically enhances the variety of instruction types, adds reasoning steps for better understanding, and improves visual comprehension by extracting detailed information from images. They tested their approach by training a model called LLaVA-NeXT with this evolved data and found that it significantly improved performance on various tasks.
Why it matters?
This research is important because it helps create better training data for MLLMs, leading to more capable AI systems that can understand and generate complex content involving both text and images. By improving how these models learn from diverse instructions, we can enhance their applications in areas like creative writing, education, and human-computer interaction.
Abstract
The development of Multimodal Large Language Models (MLLMs) has seen significant advancements. However, the quantity and quality of multimodal instruction data have emerged as significant bottlenecks in their progress. Manually creating multimodal instruction data is both time-consuming and inefficient, posing challenges in producing instructions of high complexity. Moreover, distilling instruction data from black-box commercial models (e.g., GPT-4o, GPT-4V) often results in simplistic instruction data, which constrains performance to that of these models. The challenge of curating diverse and complex instruction data remains substantial. We propose MMEvol, a novel multimodal instruction data evolution framework that combines fine-grained perception evolution, cognitive reasoning evolution, and interaction evolution. This iterative approach breaks through data quality bottlenecks to generate a complex and diverse image-text instruction dataset, thereby empowering MLLMs with enhanced capabilities. Beginning with an initial set of instructions, SEED-163K, we utilize MMEvol to systematically broadens the diversity of instruction types, integrates reasoning steps to enhance cognitive capabilities, and extracts detailed information from images to improve visual understanding and robustness. To comprehensively evaluate the effectiveness of our data, we train LLaVA-NeXT using the evolved data and conduct experiments across 13 vision-language tasks. Compared to the baseline trained with seed data, our approach achieves an average accuracy improvement of 3.1 points and reaches state-of-the-art (SOTA) performance on 9 of these tasks.