MMIU: Multimodal Multi-image Understanding for Evaluating Large Vision-Language Models
Fanqing Meng, Jin Wang, Chuanhao Li, Quanfeng Lu, Hao Tian, Jiaqi Liao, Xizhou Zhu, Jifeng Dai, Yu Qiao, Ping Luo, Kaipeng Zhang, Wenqi Shao
2024-08-07
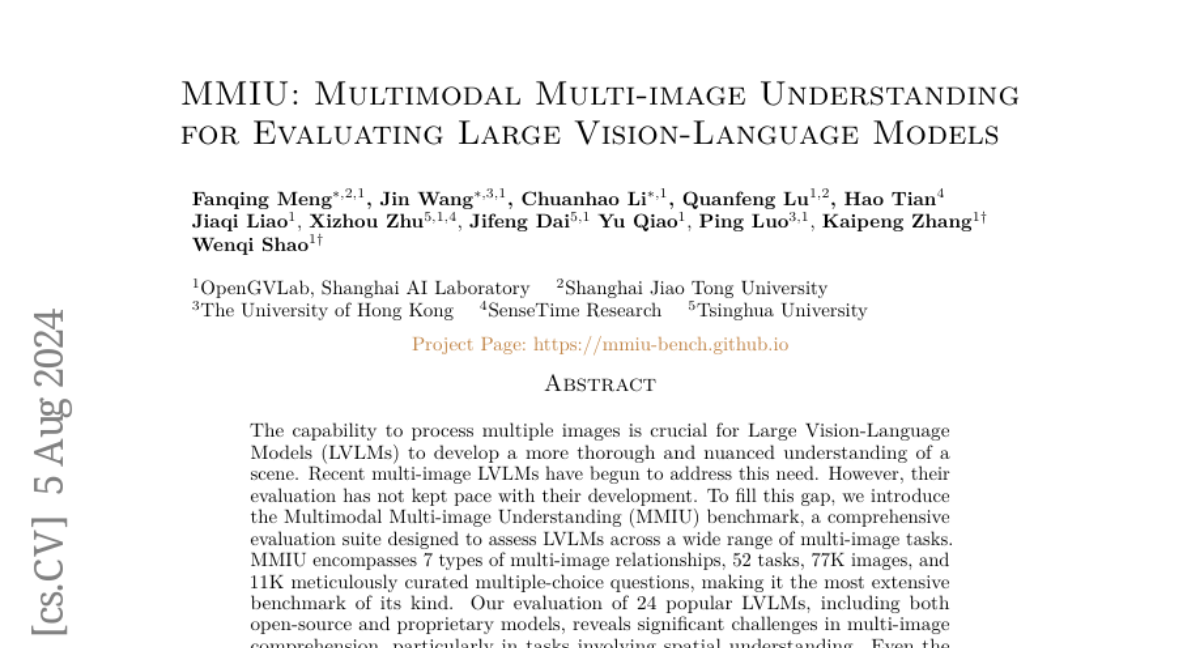
Summary
This paper presents MMIU, a new benchmark designed to evaluate how well large vision-language models (LVLMs) understand and process multiple images in various tasks.
What's the problem?
As large vision-language models become more advanced, they need to be able to analyze and understand multiple images at once to provide better insights. However, there hasn't been a proper way to evaluate these models on their ability to handle multi-image tasks, which limits our understanding of their performance.
What's the solution?
The authors introduced the Multimodal Multi-image Understanding (MMIU) benchmark, which includes 7 types of relationships between images, 52 different tasks, and a total of 77,000 images along with 11,000 carefully crafted multiple-choice questions. This extensive evaluation suite allows researchers to assess LVLMs across a wide range of multi-image tasks effectively. The study tested 24 popular LVLMs and found that even the best models struggled with understanding spatial relationships in images.
Why it matters?
MMIU is important because it provides a comprehensive way to evaluate the capabilities of LVLMs in understanding complex visual information. By identifying performance gaps in these models, this benchmark can help guide future improvements in AI technology, making it more effective for applications that require detailed visual comprehension.
Abstract
The capability to process multiple images is crucial for Large Vision-Language Models (LVLMs) to develop a more thorough and nuanced understanding of a scene. Recent multi-image LVLMs have begun to address this need. However, their evaluation has not kept pace with their development. To fill this gap, we introduce the Multimodal Multi-image Understanding (MMIU) benchmark, a comprehensive evaluation suite designed to assess LVLMs across a wide range of multi-image tasks. MMIU encompasses 7 types of multi-image relationships, 52 tasks, 77K images, and 11K meticulously curated multiple-choice questions, making it the most extensive benchmark of its kind. Our evaluation of 24 popular LVLMs, including both open-source and proprietary models, reveals significant challenges in multi-image comprehension, particularly in tasks involving spatial understanding. Even the most advanced models, such as GPT-4o, achieve only 55.7% accuracy on MMIU. Through multi-faceted analytical experiments, we identify key performance gaps and limitations, providing valuable insights for future model and data improvements. We aim for MMIU to advance the frontier of LVLM research and development, moving us toward achieving sophisticated multimodal multi-image user interactions.