MMSearch: Benchmarking the Potential of Large Models as Multi-modal Search Engines
Dongzhi Jiang, Renrui Zhang, Ziyu Guo, Yanmin Wu, Jiayi Lei, Pengshuo Qiu, Pan Lu, Zehui Chen, Guanglu Song, Peng Gao, Yu Liu, Chunyuan Li, Hongsheng Li
2024-09-20
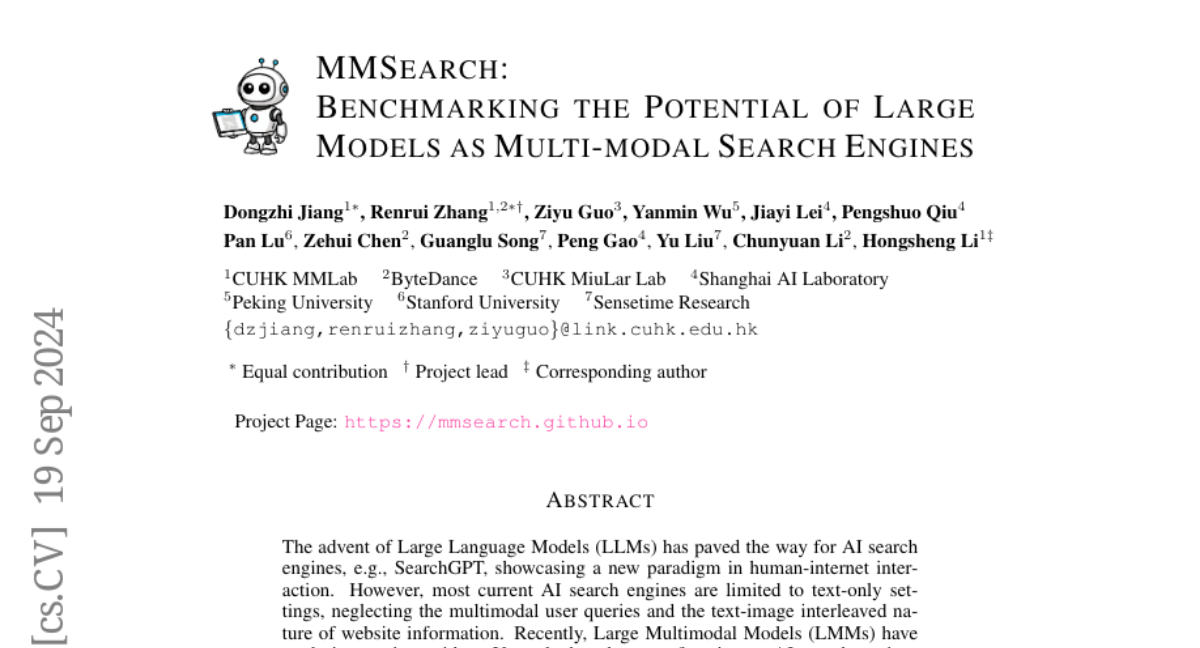
Summary
This paper introduces MMSearch, a new benchmark designed to evaluate how well large multimodal models (LMMs) can function as search engines that understand both text and images.
What's the problem?
Most current AI search engines only work with text, which limits their ability to handle complex queries that include images or mixed content. As LMMs have advanced, it's unclear if they can effectively serve as multimodal search engines that combine text and visual information.
What's the solution?
To tackle this issue, the authors created a system called MMSearch-Engine, which allows LMMs to perform multimodal searches. They also developed the MMSearch benchmark, which includes 300 carefully selected tasks across different subjects. This benchmark tests LMMs on various tasks like requerying (rephrasing a question for better search results), reranking (organizing search results by relevance), and summarization (condensing information from multiple sources). The authors conducted experiments with several models, including GPT-4o, and found that their system outperformed existing commercial search engines in certain tasks.
Why it matters?
This research is significant because it opens up new possibilities for how AI can assist users in finding information more effectively by understanding both text and images. By improving multimodal search capabilities, it can enhance user experiences in areas like online research, education, and content creation.
Abstract
The advent of Large Language Models (LLMs) has paved the way for AI search engines, e.g., SearchGPT, showcasing a new paradigm in human-internet interaction. However, most current AI search engines are limited to text-only settings, neglecting the multimodal user queries and the text-image interleaved nature of website information. Recently, Large Multimodal Models (LMMs) have made impressive strides. Yet, whether they can function as AI search engines remains under-explored, leaving the potential of LMMs in multimodal search an open question. To this end, we first design a delicate pipeline, MMSearch-Engine, to empower any LMMs with multimodal search capabilities. On top of this, we introduce MMSearch, a comprehensive evaluation benchmark to assess the multimodal search performance of LMMs. The curated dataset contains 300 manually collected instances spanning 14 subfields, which involves no overlap with the current LMMs' training data, ensuring the correct answer can only be obtained within searching. By using MMSearch-Engine, the LMMs are evaluated by performing three individual tasks (requery, rerank, and summarization), and one challenging end-to-end task with a complete searching process. We conduct extensive experiments on closed-source and open-source LMMs. Among all tested models, GPT-4o with MMSearch-Engine achieves the best results, which surpasses the commercial product, Perplexity Pro, in the end-to-end task, demonstrating the effectiveness of our proposed pipeline. We further present error analysis to unveil current LMMs still struggle to fully grasp the multimodal search tasks, and conduct ablation study to indicate the potential of scaling test-time computation for AI search engine. We hope MMSearch may provide unique insights to guide the future development of multimodal AI search engine. Project Page: https://mmsearch.github.io