Model Merging and Safety Alignment: One Bad Model Spoils the Bunch
Hasan Abed Al Kader Hammoud, Umberto Michieli, Fabio Pizzati, Philip Torr, Adel Bibi, Bernard Ghanem, Mete Ozay
2024-06-21
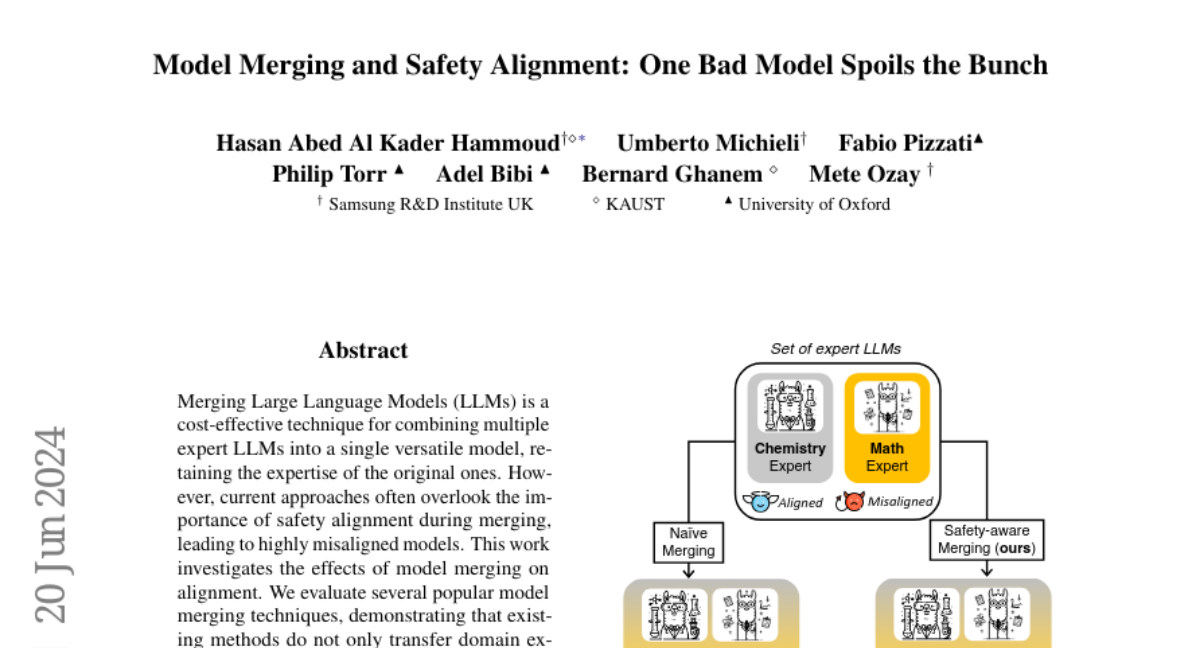
Summary
This paper discusses the challenges and solutions related to merging large language models (LLMs) while ensuring they remain safe and aligned in their responses.
What's the problem?
Merging multiple LLMs into one powerful model can save time and resources, but it often leads to problems with safety and alignment. When different models are combined, they may not work well together, which can result in the new model producing unsafe or incorrect outputs. This is a significant concern, especially in applications where safety is critical.
What's the solution?
The researchers propose a two-step approach to improve the merging process. First, they suggest creating synthetic data that focuses on safety and specific domains. Then, they recommend using this data to enhance the merging techniques already in use. By doing this, they treat alignment as a skill that can be improved, ensuring that the merged model maintains both its expertise in specific areas and its safety standards.
Why it matters?
This research is important because it addresses a crucial issue in AI development: how to safely combine different models without losing their effectiveness. By improving the merging process, developers can create more reliable AI systems that are better suited for real-world applications, ultimately leading to safer technology that users can trust.
Abstract
Merging Large Language Models (LLMs) is a cost-effective technique for combining multiple expert LLMs into a single versatile model, retaining the expertise of the original ones. However, current approaches often overlook the importance of safety alignment during merging, leading to highly misaligned models. This work investigates the effects of model merging on alignment. We evaluate several popular model merging techniques, demonstrating that existing methods do not only transfer domain expertise but also propagate misalignment. We propose a simple two-step approach to address this problem: (i) generating synthetic safety and domain-specific data, and (ii) incorporating these generated data into the optimization process of existing data-aware model merging techniques. This allows us to treat alignment as a skill that can be maximized in the resulting merged LLM. Our experiments illustrate the effectiveness of integrating alignment-related data during merging, resulting in models that excel in both domain expertise and alignment.