Molmo and PixMo: Open Weights and Open Data for State-of-the-Art Multimodal Models
Matt Deitke, Christopher Clark, Sangho Lee, Rohun Tripathi, Yue Yang, Jae Sung Park, Mohammadreza Salehi, Niklas Muennighoff, Kyle Lo, Luca Soldaini, Jiasen Lu, Taira Anderson, Erin Bransom, Kiana Ehsani, Huong Ngo, YenSung Chen, Ajay Patel, Mark Yatskar, Chris Callison-Burch, Andrew Head, Rose Hendrix, Favyen Bastani
2024-09-26
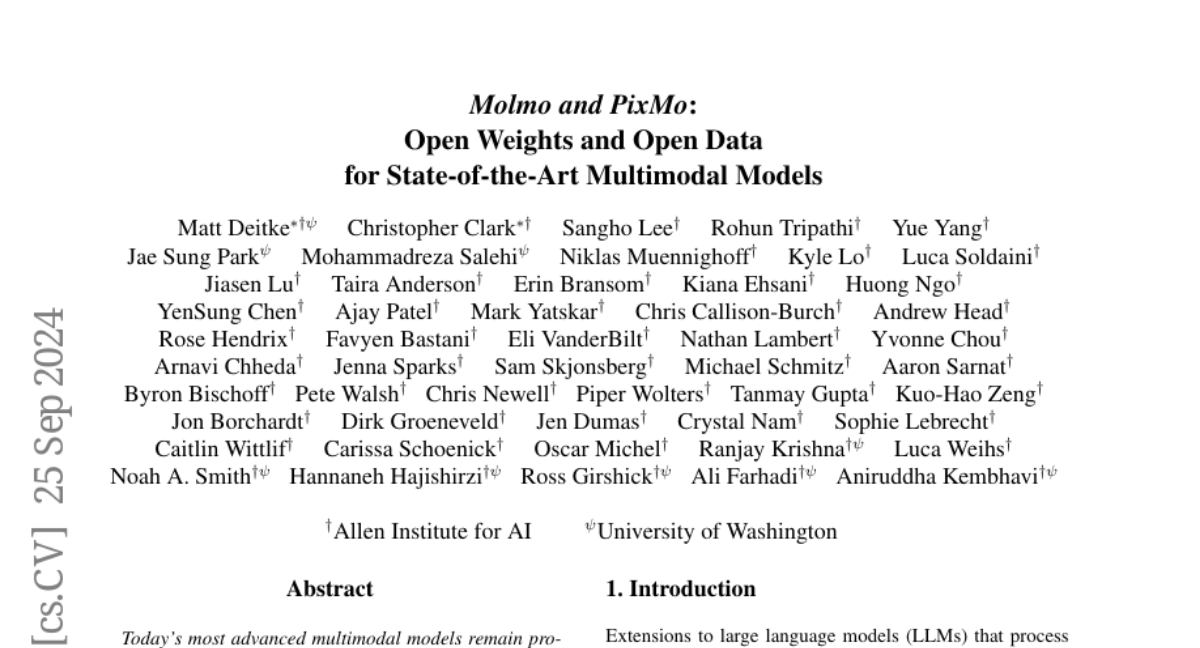
Summary
This paper presents Molmo and PixMo, a new family of open-weight multimodal models that excel in processing both images and text. The authors highlight their innovative approach to creating high-quality datasets and training models that can outperform existing proprietary systems.
What's the problem?
Most advanced multimodal models are proprietary, meaning they are not openly available for others to use or learn from. This limits the community's ability to understand how to build effective models from scratch. Additionally, existing open-weight models often rely on synthetic data from closed systems, which does not provide foundational knowledge for developing new models.
What's the solution?
To address these issues, the researchers introduced Molmo, which is built on a new dataset of image captions created by human annotators using speech descriptions. They also developed PixMo, which includes diverse data for fine-tuning the model, such as question-and-answer interactions and 2D pointing data. Their approach focuses on careful model architecture and training processes, ensuring high performance while being open and accessible to the community.
Why it matters?
This research is significant because it provides valuable resources and knowledge for developing multimodal models that can process both images and text effectively. By releasing their model weights, datasets, and source code, the authors aim to empower other researchers and developers to build upon their work, fostering innovation in the field of artificial intelligence.
Abstract
Today's most advanced multimodal models remain proprietary. The strongest open-weight models rely heavily on synthetic data from proprietary VLMs to achieve good performance, effectively distilling these closed models into open ones. As a result, the community is still missing foundational knowledge about how to build performant VLMs from scratch. We present Molmo, a new family of VLMs that are state-of-the-art in their class of openness. Our key innovation is a novel, highly detailed image caption dataset collected entirely from human annotators using speech-based descriptions. To enable a wide array of user interactions, we also introduce a diverse dataset mixture for fine-tuning that includes in-the-wild Q&A and innovative 2D pointing data. The success of our approach relies on careful choices for the model architecture details, a well-tuned training pipeline, and, most critically, the quality of our newly collected datasets, all of which will be released. The best-in-class 72B model within the Molmo family not only outperforms others in the class of open weight and data models but also compares favorably against proprietary systems like GPT-4o, Claude 3.5, and Gemini 1.5 on both academic benchmarks and human evaluation. We will be releasing all of our model weights, captioning and fine-tuning data, and source code in the near future. Select model weights, inference code, and demo are available at https://molmo.allenai.org.