MOMAland: A Set of Benchmarks for Multi-Objective Multi-Agent Reinforcement Learning
Florian Felten, Umut Ucak, Hicham Azmani, Gao Peng, Willem Röpke, Hendrik Baier, Patrick Mannion, Diederik M. Roijers, Jordan K. Terry, El-Ghazali Talbi, Grégoire Danoy, Ann Nowé, Roxana Rădulescu
2024-07-25
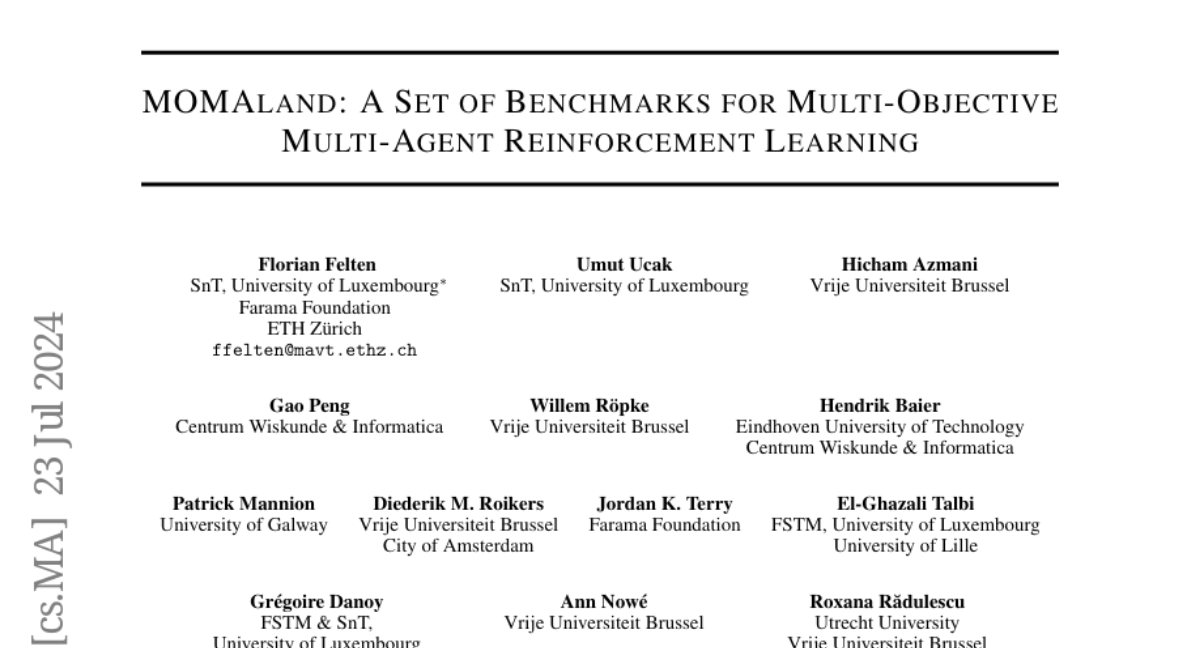
Summary
This paper introduces MOMAland, a new set of benchmarks designed for multi-objective multi-agent reinforcement learning (MOMARL). It provides standardized environments to help researchers develop and test algorithms that can handle complex decision-making tasks involving multiple agents with different goals.
What's the problem?
In many real-world scenarios, such as managing traffic or supply chains, multiple independent decision-makers must work together while balancing conflicting objectives. Current methods for training AI in these situations lack comprehensive benchmarks to evaluate how well they perform across different tasks and environments. This makes it difficult to measure progress and improve algorithms effectively.
What's the solution?
MOMAland addresses this issue by offering a collection of over 10 standardized environments specifically designed for MOMARL. These environments vary in the number of agents, the types of tasks they need to accomplish, and how rewards are structured. By providing these benchmarks, MOMAland allows researchers to systematically evaluate their algorithms and compare their performance against established standards.
Why it matters?
This research is important because it helps advance the field of multi-agent reinforcement learning by creating a solid foundation for testing and improving algorithms. With better benchmarks, researchers can develop more effective AI systems that can handle complex, real-world problems involving multiple agents, leading to improved solutions in areas like traffic management, energy distribution, and logistics.
Abstract
Many challenging tasks such as managing traffic systems, electricity grids, or supply chains involve complex decision-making processes that must balance multiple conflicting objectives and coordinate the actions of various independent decision-makers (DMs). One perspective for formalising and addressing such tasks is multi-objective multi-agent reinforcement learning (MOMARL). MOMARL broadens reinforcement learning (RL) to problems with multiple agents each needing to consider multiple objectives in their learning process. In reinforcement learning research, benchmarks are crucial in facilitating progress, evaluation, and reproducibility. The significance of benchmarks is underscored by the existence of numerous benchmark frameworks developed for various RL paradigms, including single-agent RL (e.g., Gymnasium), multi-agent RL (e.g., PettingZoo), and single-agent multi-objective RL (e.g., MO-Gymnasium). To support the advancement of the MOMARL field, we introduce MOMAland, the first collection of standardised environments for multi-objective multi-agent reinforcement learning. MOMAland addresses the need for comprehensive benchmarking in this emerging field, offering over 10 diverse environments that vary in the number of agents, state representations, reward structures, and utility considerations. To provide strong baselines for future research, MOMAland also includes algorithms capable of learning policies in such settings.