MooER: LLM-based Speech Recognition and Translation Models from Moore Threads
Junhao Xu, Zhenlin Liang, Yi Liu, Yichao Hu, Jian Li, Yajun Zheng, Meng Cai, Hua Wang
2024-08-12
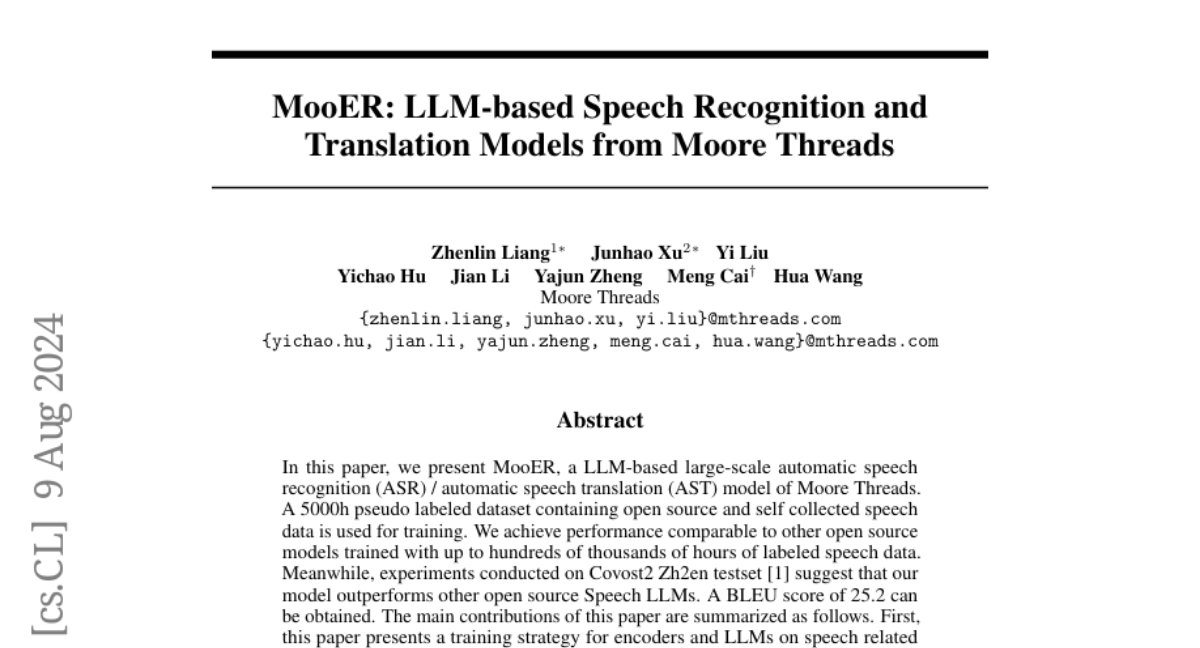
Summary
This paper introduces MooER, a new model developed by Moore Threads for automatic speech recognition (ASR) and automatic speech translation (AST) that uses a large dataset of speech data to perform well without needing extensive manual labeling.
What's the problem?
Creating effective models for recognizing and translating speech is challenging, especially when there isn't enough labeled data available. Most existing models rely on hundreds of thousands of hours of labeled speech data, which can be expensive and time-consuming to collect. This limits the ability to develop robust speech recognition systems, particularly for less common languages or dialects.
What's the solution?
MooER addresses this issue by using a dataset of 5,000 hours of pseudo-labeled speech data, which includes both open-source and self-collected recordings. The model is trained using a new strategy that allows it to learn from this smaller dataset without requiring extensive manual annotations. Experiments show that MooER performs comparably to other models trained on much larger datasets, achieving a BLEU score of 25.2 on translation tasks, indicating strong performance in translating spoken language.
Why it matters?
This research is important because it demonstrates that high-quality speech recognition and translation can be achieved with less labeled data, making these technologies more accessible. By releasing MooER and its training methods as open-source, the authors aim to encourage further development in the field, allowing more researchers and developers to create effective speech technologies without the need for massive datasets.
Abstract
In this paper, we present MooER, a LLM-based large-scale automatic speech recognition (ASR) / automatic speech translation (AST) model of Moore Threads. A 5000h pseudo labeled dataset containing open source and self collected speech data is used for training. We achieve performance comparable to other open source models trained with up to hundreds of thousands of hours of labeled speech data. Meanwhile, experiments conducted on Covost2 Zh2en testset suggest that our model outperforms other open source Speech LLMs. A BLEU score of 25.2 can be obtained. The main contributions of this paper are summarized as follows. First, this paper presents a training strategy for encoders and LLMs on speech related tasks (including ASR and AST) using a small size of pseudo labeled data without any extra manual annotation and selection. Second, we release our ASR and AST models and plan to open-source our training code and strategy in the near future. Moreover, a model trained on 8wh scale training data is planned to be released later on.