mOSCAR: A Large-scale Multilingual and Multimodal Document-level Corpus
Matthieu Futeral, Armel Zebaze, Pedro Ortiz Suarez, Julien Abadji, Rémi Lacroix, Cordelia Schmid, Rachel Bawden, Benoît Sagot
2024-06-14
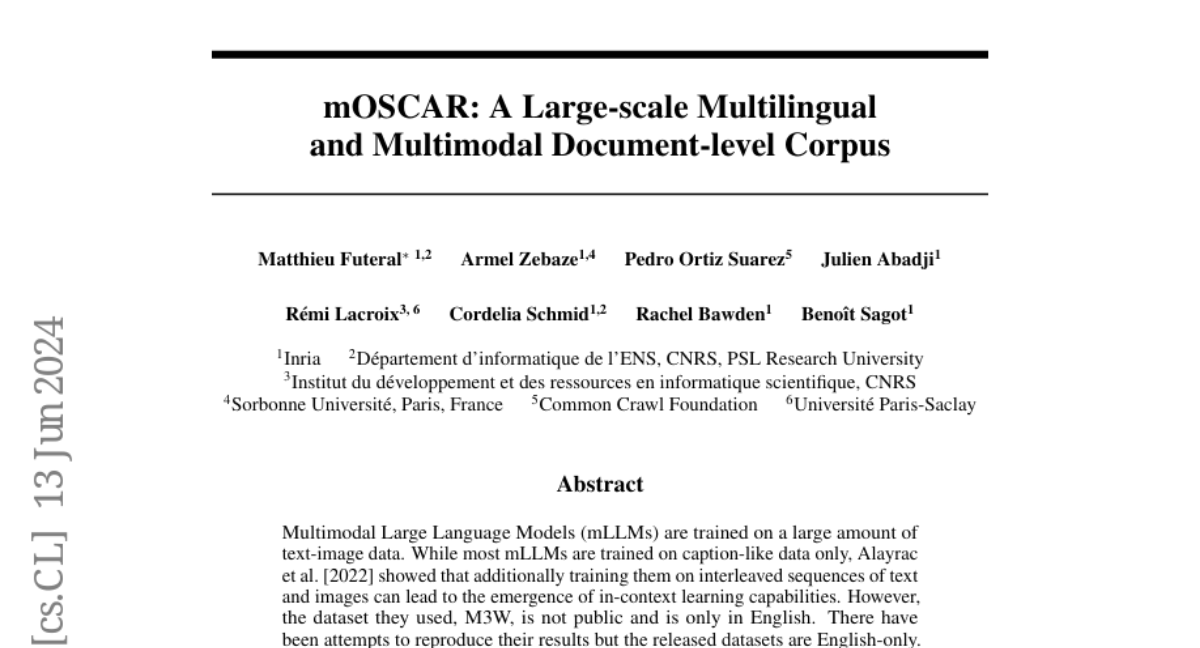
Summary
This paper introduces mOSCAR, a large-scale multilingual and multimodal document corpus designed to improve the training of multimodal large language models (mLLMs) that work with both text and images. It aims to support research across many languages and enhance the models' ability to learn from diverse data.
What's the problem?
Most existing datasets for training mLLMs are either limited to English or consist only of caption-like data, which restricts their effectiveness in understanding and generating content in other languages. This is a problem because there are over 7,000 languages spoken worldwide, and researchers need data that reflects this diversity to train more capable models.
What's the solution?
To address this issue, the authors created mOSCAR, which includes a massive collection of 315 million documents covering 163 languages, along with 214 billion tokens and 1.2 billion images. They implemented careful filtering and evaluation processes to ensure the quality and safety of the dataset. Additionally, they trained two types of multilingual models: one using a subset of mOSCAR combined with captioning data, and another using only captioning data. The results showed that the model trained with mOSCAR performed significantly better in various tasks involving text and images.
Why it matters?
This research is important because it provides a valuable resource for developing more effective AI models that can understand and generate content in multiple languages. By making mOSCAR publicly available, it encourages further advancements in multilingual AI research and helps bridge the gap in language representation, ultimately leading to better tools for communication and information access worldwide.
Abstract
Multimodal Large Language Models (mLLMs) are trained on a large amount of text-image data. While most mLLMs are trained on caption-like data only, Alayrac et al. [2022] showed that additionally training them on interleaved sequences of text and images can lead to the emergence of in-context learning capabilities. However, the dataset they used, M3W, is not public and is only in English. There have been attempts to reproduce their results but the released datasets are English-only. In contrast, current multilingual and multimodal datasets are either composed of caption-like only or medium-scale or fully private data. This limits mLLM research for the 7,000 other languages spoken in the world. We therefore introduce mOSCAR, to the best of our knowledge the first large-scale multilingual and multimodal document corpus crawled from the web. It covers 163 languages, 315M documents, 214B tokens and 1.2B images. We carefully conduct a set of filtering and evaluation steps to make sure mOSCAR is sufficiently safe, diverse and of good quality. We additionally train two types of multilingual model to prove the benefits of mOSCAR: (1) a model trained on a subset of mOSCAR and captioning data and (2) a model train on captioning data only. The model additionally trained on mOSCAR shows a strong boost in few-shot learning performance across various multilingual image-text tasks and benchmarks, confirming previous findings for English-only mLLMs.