MotionBench: Benchmarking and Improving Fine-grained Video Motion Understanding for Vision Language Models
Wenyi Hong, Yean Cheng, Zhuoyi Yang, Weihan Wang, Lefan Wang, Xiaotao Gu, Shiyu Huang, Yuxiao Dong, Jie Tang
2025-01-08
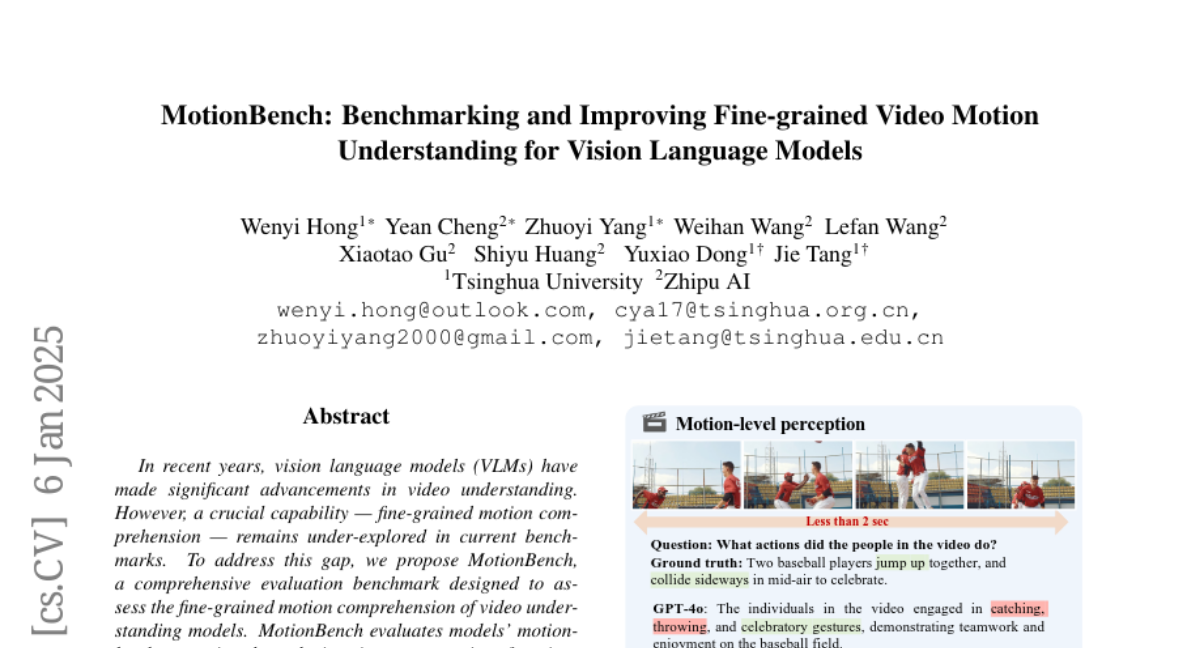
Summary
This paper talks about MotionBench, a new tool to test and improve how well AI models can understand detailed movements in videos.
What's the problem?
Current AI models that work with videos (called Vision Language Models or VLMs) are getting better at understanding videos in general. However, they're not very good at understanding specific, detailed movements within those videos. There hasn't been a good way to test this ability until now.
What's the solution?
The researchers created MotionBench, which is like a big test for AI models. It includes lots of different types of videos and asks the AI models questions about specific movements in these videos. They also came up with a new method called Through-Encoder (TE) Fusion to help AI models better understand movements in videos without needing too much computer power.
Why it matters?
This matters because understanding detailed movements is crucial for AI to truly comprehend what's happening in videos. It could lead to better AI assistants that can describe videos more accurately, improved security cameras that can detect specific actions, or even help in creating more advanced robotics. By providing a way to test and improve this ability, MotionBench could push AI research forward, leading to smarter and more useful video understanding systems.
Abstract
In recent years, vision language models (VLMs) have made significant advancements in video understanding. However, a crucial capability - fine-grained motion comprehension - remains under-explored in current benchmarks. To address this gap, we propose MotionBench, a comprehensive evaluation benchmark designed to assess the fine-grained motion comprehension of video understanding models. MotionBench evaluates models' motion-level perception through six primary categories of motion-oriented question types and includes data collected from diverse sources, ensuring a broad representation of real-world video content. Experimental results reveal that existing VLMs perform poorly in understanding fine-grained motions. To enhance VLM's ability to perceive fine-grained motion within a limited sequence length of LLM, we conduct extensive experiments reviewing VLM architectures optimized for video feature compression and propose a novel and efficient Through-Encoder (TE) Fusion method. Experiments show that higher frame rate inputs and TE Fusion yield improvements in motion understanding, yet there is still substantial room for enhancement. Our benchmark aims to guide and motivate the development of more capable video understanding models, emphasizing the importance of fine-grained motion comprehension. Project page: https://motion-bench.github.io .