MovieDreamer: Hierarchical Generation for Coherent Long Visual Sequence
Canyu Zhao, Mingyu Liu, Wen Wang, Jianlong Yuan, Hao Chen, Bo Zhang, Chunhua Shen
2024-07-24
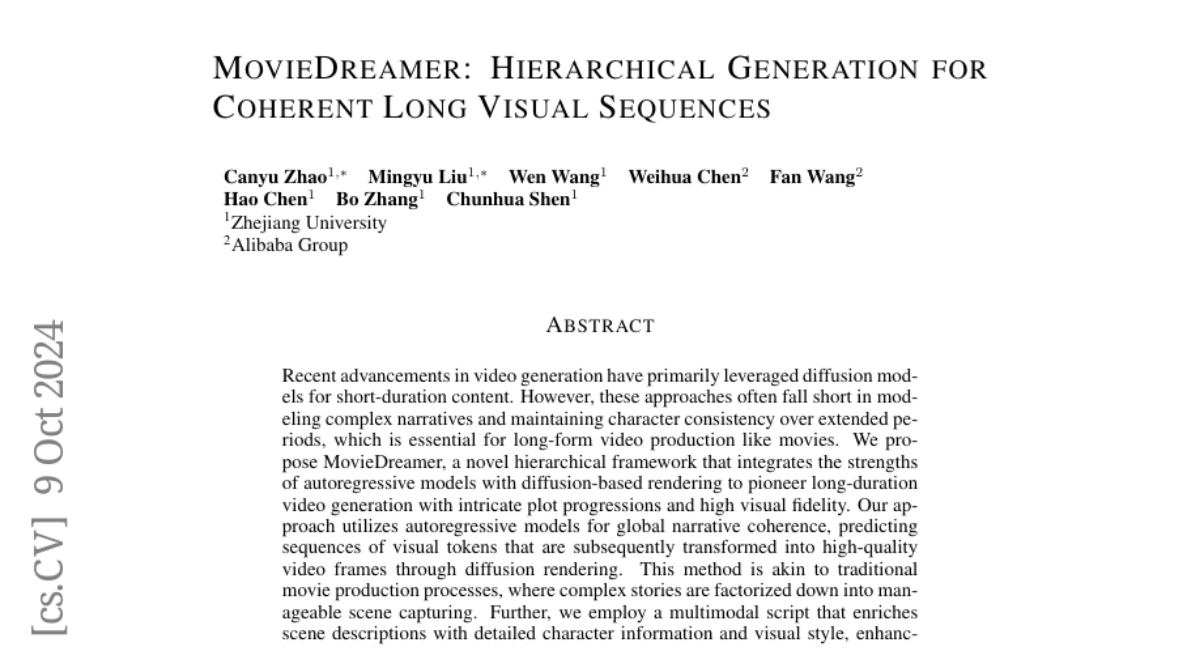
Summary
This paper introduces MovieDreamer, a new method for generating long videos that tells coherent stories. It combines different techniques to ensure that the videos maintain character consistency and narrative flow over time, similar to how movies are traditionally made.
What's the problem?
Current video generation methods often struggle with creating long videos that have complex plots and consistent characters. Most existing models are good at making short clips but fail to keep the story coherent and the characters recognizable when the video is longer. This makes it difficult to produce high-quality, engaging content for things like movies or series.
What's the solution?
MovieDreamer addresses these issues by using a hierarchical framework that blends autoregressive models (which predict sequences step by step) with diffusion-based rendering (which creates high-quality images). The model first generates a rough outline of the story, then fills in details to create high-quality video frames. Additionally, it uses a multimodal script that adds rich descriptions of scenes and characters, helping to maintain continuity and character identity throughout the video. This approach allows for better storytelling and visual quality over longer durations.
Why it matters?
This research is important because it pushes the boundaries of what AI can do in video generation. By enabling the creation of longer, more complex videos with high visual fidelity and coherent narratives, MovieDreamer opens up new possibilities for filmmakers, game developers, and content creators. It can significantly enhance how stories are told through visual media, making it easier to produce engaging content.
Abstract
Recent advancements in video generation have primarily leveraged diffusion models for short-duration content. However, these approaches often fall short in modeling complex narratives and maintaining character consistency over extended periods, which is essential for long-form video production like movies. We propose MovieDreamer, a novel hierarchical framework that integrates the strengths of autoregressive models with diffusion-based rendering to pioneer long-duration video generation with intricate plot progressions and high visual fidelity. Our approach utilizes autoregressive models for global narrative coherence, predicting sequences of visual tokens that are subsequently transformed into high-quality video frames through diffusion rendering. This method is akin to traditional movie production processes, where complex stories are factorized down into manageable scene capturing. Further, we employ a multimodal script that enriches scene descriptions with detailed character information and visual style, enhancing continuity and character identity across scenes. We present extensive experiments across various movie genres, demonstrating that our approach not only achieves superior visual and narrative quality but also effectively extends the duration of generated content significantly beyond current capabilities. Homepage: https://aim-uofa.github.io/MovieDreamer/.