Multi-Draft Speculative Sampling: Canonical Architectures and Theoretical Limits
Ashish Khisti, M. Reza Ebrahimi, Hassan Dbouk, Arash Behboodi, Roland Memisevic, Christos Louizos
2024-10-25
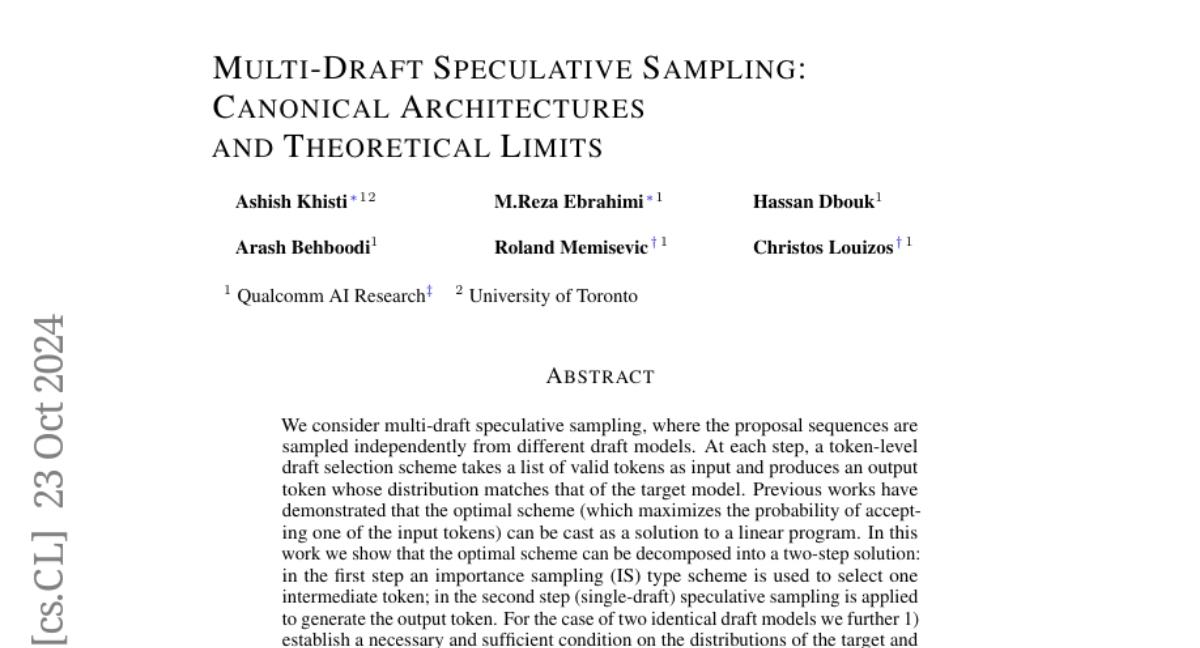
Summary
This paper discusses a new method called Multi-Draft Speculative Sampling, which improves how language models generate text by using multiple draft models to select the best tokens for output.
What's the problem?
When language models generate text, they often need to choose from many possible words or tokens at each step. Traditional methods can be inefficient and may not always produce the best results, especially when it comes to selecting the most relevant tokens from different drafts or versions of the model.
What's the solution?
The authors propose a two-step approach to enhance token selection. First, they use an importance sampling method to pick one intermediate token from various draft models. Then, they apply speculative sampling to generate the final output token. This method helps ensure that the selected tokens align closely with what the target model would produce, improving overall performance. They also establish conditions under which this method works best and introduce a new selection scheme based on weighted importance sampling.
Why it matters?
This research is important because it offers a more efficient way for language models to generate text, potentially leading to better quality outputs in applications like chatbots, content creation, and other AI-driven tasks. By optimizing how models select tokens, this method can help improve the speed and accuracy of language generation.
Abstract
We consider multi-draft speculative sampling, where the proposal sequences are sampled independently from different draft models. At each step, a token-level draft selection scheme takes a list of valid tokens as input and produces an output token whose distribution matches that of the target model. Previous works have demonstrated that the optimal scheme (which maximizes the probability of accepting one of the input tokens) can be cast as a solution to a linear program. In this work we show that the optimal scheme can be decomposed into a two-step solution: in the first step an importance sampling (IS) type scheme is used to select one intermediate token; in the second step (single-draft) speculative sampling is applied to generate the output token. For the case of two identical draft models we further 1) establish a necessary and sufficient condition on the distributions of the target and draft models for the acceptance probability to equal one and 2) provide an explicit expression for the optimal acceptance probability. Our theoretical analysis also motives a new class of token-level selection scheme based on weighted importance sampling. Our experimental results demonstrate consistent improvements in the achievable block efficiency and token rates over baseline schemes in a number of scenarios.