Multi-Object Hallucination in Vision-Language Models
Xuweiyi Chen, Ziqiao Ma, Xuejun Zhang, Sihan Xu, Shengyi Qian, Jianing Yang, David F. Fouhey, Joyce Chai
2024-07-09
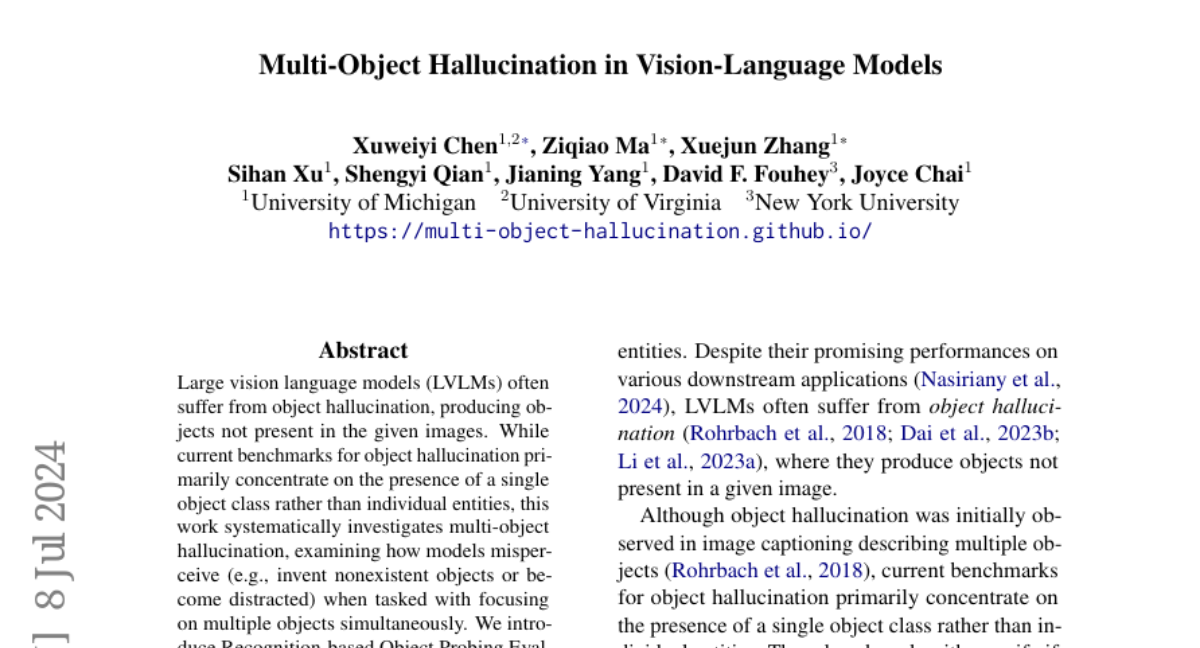
Summary
This paper talks about the problem of multi-object hallucination in large vision language models (LVLMs), where these models incorrectly generate or identify objects that aren't actually present in images. It introduces a new evaluation method called ROPE to better understand and measure this issue.
What's the problem?
The main problem is that LVLMs often make mistakes when trying to recognize multiple objects in an image. Instead of accurately identifying what is there, they may invent objects that don't exist or get confused by the presence of many items. Current tests mainly focus on single objects, which doesn't fully capture how these models perform in more complex situations with multiple objects.
What's the solution?
To address this, the authors developed Recognition-based Object Probing Evaluation (ROPE), a new way to evaluate how well LVLMs handle images with multiple objects. ROPE looks at how different types of objects are distributed within an image and uses specific visual prompts to clarify what the model should focus on. Through experiments, they found that LVLMs tend to hallucinate more when trying to understand multiple objects at once, and that factors like how often certain objects appear and their importance can influence these hallucinations.
Why it matters?
This research is important because it helps improve the accuracy of AI systems that interact with visual data. By understanding and addressing the issue of multi-object hallucination, we can enhance how models recognize and reason about real-world scenes, leading to better performance in applications like image captioning, visual search, and assisting visually impaired individuals.
Abstract
Large vision language models (LVLMs) often suffer from object hallucination, producing objects not present in the given images. While current benchmarks for object hallucination primarily concentrate on the presence of a single object class rather than individual entities, this work systematically investigates multi-object hallucination, examining how models misperceive (e.g., invent nonexistent objects or become distracted) when tasked with focusing on multiple objects simultaneously. We introduce Recognition-based Object Probing Evaluation (ROPE), an automated evaluation protocol that considers the distribution of object classes within a single image during testing and uses visual referring prompts to eliminate ambiguity. With comprehensive empirical studies and analysis of potential factors leading to multi-object hallucination, we found that (1) LVLMs suffer more hallucinations when focusing on multiple objects compared to a single object. (2) The tested object class distribution affects hallucination behaviors, indicating that LVLMs may follow shortcuts and spurious correlations.(3) Hallucinatory behaviors are influenced by data-specific factors, salience and frequency, and model intrinsic behaviors. We hope to enable LVLMs to recognize and reason about multiple objects that often occur in realistic visual scenes, provide insights, and quantify our progress towards mitigating the issues.