Multimodal Latent Language Modeling with Next-Token Diffusion
Yutao Sun, Hangbo Bao, Wenhui Wang, Zhiliang Peng, Li Dong, Shaohan Huang, Jianyong Wang, Furu Wei
2024-12-13
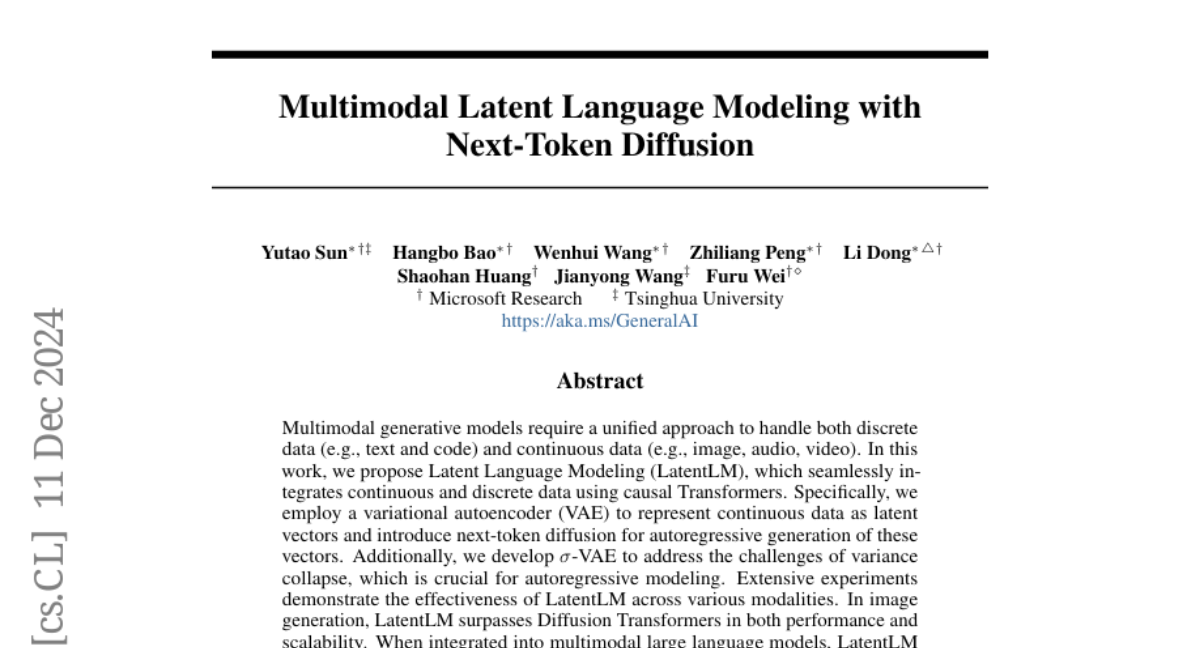
Summary
This paper introduces Latent Language Modeling (LatentLM), a new approach that combines different types of data, like text and images, to improve how AI understands and generates information.
What's the problem?
Many AI models struggle to handle both discrete data (like text) and continuous data (like images or audio) at the same time. This makes it difficult for them to generate coherent responses or understand complex inputs, especially in tasks that require knowledge from multiple sources.
What's the solution?
LatentLM addresses this issue by using a method called next-token diffusion, which helps the model learn how to generate both types of data effectively. It employs a variational autoencoder (VAE) to convert continuous data into a format that the model can work with, and it introduces a new technique to prevent problems during training. By doing extensive experiments, the authors show that LatentLM performs better than existing models in generating images and understanding speech while using fewer resources.
Why it matters?
This research is important because it enhances the capabilities of AI models, making them more versatile and efficient. By successfully integrating different types of data, LatentLM can improve applications in various fields, such as image generation, text-to-speech systems, and more complex AI tasks that require understanding multiple forms of information.
Abstract
Multimodal generative models require a unified approach to handle both discrete data (e.g., text and code) and continuous data (e.g., image, audio, video). In this work, we propose Latent Language Modeling (LatentLM), which seamlessly integrates continuous and discrete data using causal Transformers. Specifically, we employ a variational autoencoder (VAE) to represent continuous data as latent vectors and introduce next-token diffusion for autoregressive generation of these vectors. Additionally, we develop sigma-VAE to address the challenges of variance collapse, which is crucial for autoregressive modeling. Extensive experiments demonstrate the effectiveness of LatentLM across various modalities. In image generation, LatentLM surpasses Diffusion Transformers in both performance and scalability. When integrated into multimodal large language models, LatentLM provides a general-purpose interface that unifies multimodal generation and understanding. Experimental results show that LatentLM achieves favorable performance compared to Transfusion and vector quantized models in the setting of scaling up training tokens. In text-to-speech synthesis, LatentLM outperforms the state-of-the-art VALL-E 2 model in speaker similarity and robustness, while requiring 10x fewer decoding steps. The results establish LatentLM as a highly effective and scalable approach to advance large multimodal models.