MultiVENT 2.0: A Massive Multilingual Benchmark for Event-Centric Video Retrieval
Reno Kriz, Kate Sanders, David Etter, Kenton Murray, Cameron Carpenter, Kelly Van Ochten, Hannah Recknor, Jimena Guallar-Blasco, Alexander Martin, Ronald Colaianni, Nolan King, Eugene Yang, Benjamin Van Durme
2024-10-16
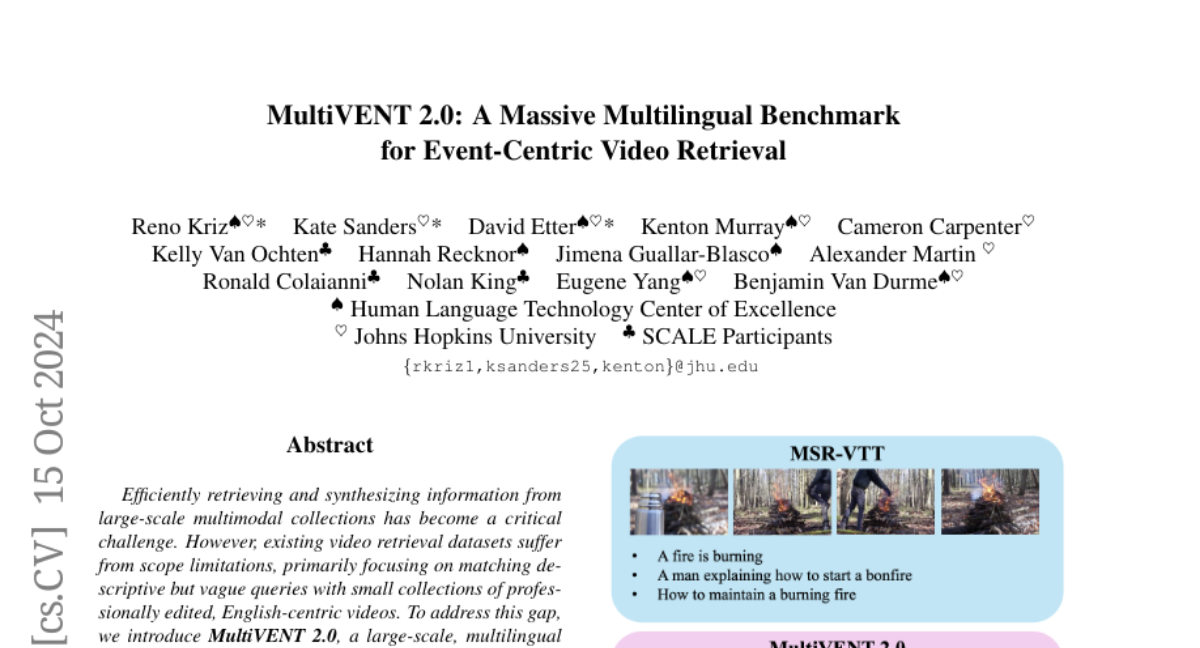
Summary
This paper introduces MultiVENT 2.0, a large-scale benchmark designed to improve how we search for and retrieve videos based on specific events, using a wide range of queries.
What's the problem?
Many existing video retrieval systems focus on matching vague descriptions with small collections of videos, often only in English. This limits their effectiveness in finding relevant information in diverse and complex video datasets, especially when dealing with real-world events.
What's the solution?
To tackle this issue, the authors created MultiVENT 2.0, which includes over 218,000 news videos and nearly 4,000 specific queries about various world events. These queries require the retrieval system to analyze not just the spoken words but also visual content, audio, and text within the videos. This comprehensive approach helps improve the accuracy of video retrieval.
Why it matters?
This research is important because it provides a more robust tool for retrieving information from videos, which is essential for understanding current events and accessing relevant media. By improving video retrieval systems, MultiVENT 2.0 can enhance applications in journalism, education, and content creation, making it easier for users to find the information they need.
Abstract
Efficiently retrieving and synthesizing information from large-scale multimodal collections has become a critical challenge. However, existing video retrieval datasets suffer from scope limitations, primarily focusing on matching descriptive but vague queries with small collections of professionally edited, English-centric videos. To address this gap, we introduce MultiVENT 2.0, a large-scale, multilingual event-centric video retrieval benchmark featuring a collection of more than 218,000 news videos and 3,906 queries targeting specific world events. These queries specifically target information found in the visual content, audio, embedded text, and text metadata of the videos, requiring systems leverage all these sources to succeed at the task. Preliminary results show that state-of-the-art vision-language models struggle significantly with this task, and while alternative approaches show promise, they are still insufficient to adequately address this problem. These findings underscore the need for more robust multimodal retrieval systems, as effective video retrieval is a crucial step towards multimodal content understanding and generation tasks.