MURI: High-Quality Instruction Tuning Datasets for Low-Resource Languages via Reverse Instructions
Abdullatif Köksal, Marion Thaler, Ayyoob Imani, Ahmet Üstün, Anna Korhonen, Hinrich Schütze
2024-09-20
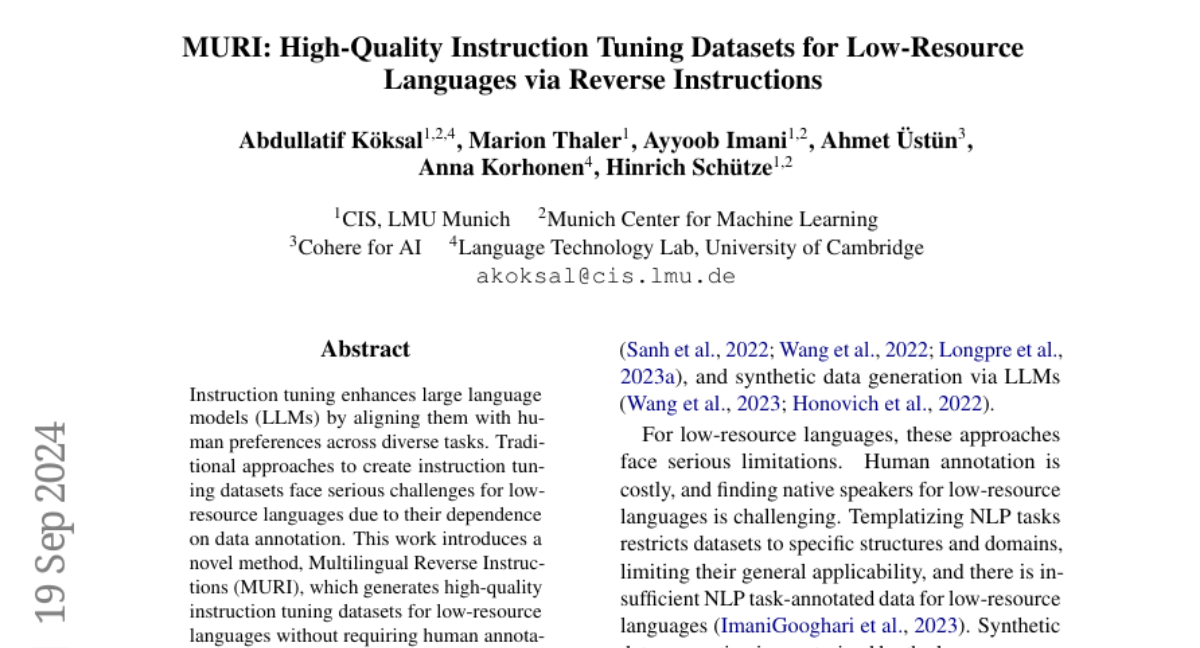
Summary
This paper introduces a new method called Multilingual Reverse Instructions (MURI) to create high-quality instruction tuning datasets for languages that don't have a lot of resources. This method helps improve language models by generating instructions from existing texts without needing human annotators.
What's the problem?
Creating instruction tuning datasets for low-resource languages is challenging because it usually requires a lot of human effort to annotate data. Many languages lack sufficient data, making it hard to train effective language models that can understand and generate instructions accurately.
What's the solution?
MURI solves this problem by using a technique called reverse instructions, which generates instruction-output pairs from existing human-written texts in low-resource languages. It combines this with a translation process to ensure the generated instructions are culturally relevant and appropriate. The resulting dataset, MURI-IT, includes over 2 million instruction-output pairs across 200 languages, allowing for better training of language models without the need for extensive human input.
Why it matters?
This research is important because it makes advanced language technology more accessible to speakers of low-resource languages. By providing high-quality datasets, MURI can help improve the performance of language models in diverse languages, ensuring that more people can benefit from AI technologies in their native languages.
Abstract
Instruction tuning enhances large language models (LLMs) by aligning them with human preferences across diverse tasks. Traditional approaches to create instruction tuning datasets face serious challenges for low-resource languages due to their dependence on data annotation. This work introduces a novel method, Multilingual Reverse Instructions (MURI), which generates high-quality instruction tuning datasets for low-resource languages without requiring human annotators or pre-existing multilingual models. Utilizing reverse instructions and a translation pipeline, MURI produces instruction-output pairs from existing human-written texts in low-resource languages. This method ensures cultural relevance and diversity by sourcing texts from different native domains and applying filters to eliminate inappropriate content. Our dataset, MURI-IT, includes more than 2 million instruction-output pairs across 200 languages. Evaluation by native speakers and fine-tuning experiments with mT5 models demonstrate the approach's effectiveness for both NLU and open-ended generation. We publicly release datasets and models at https://github.com/akoksal/muri.