MUSCLE: A Model Update Strategy for Compatible LLM Evolution
Jessica Echterhoff, Fartash Faghri, Raviteja Vemulapalli, Ting-Yao Hu, Chun-Liang Li, Oncel Tuzel, Hadi Pouransari
2024-07-15
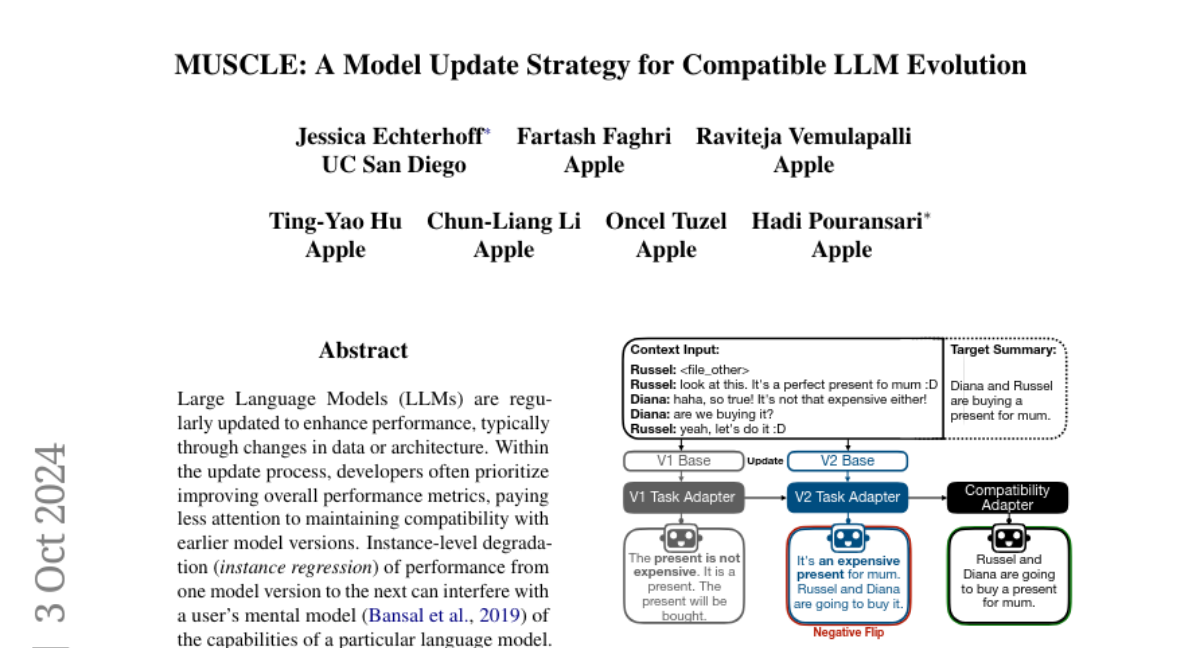
Summary
This paper presents MUSCLE, a new strategy for updating large language models (LLMs) in a way that keeps them compatible with previous versions, making it easier for users to adapt to changes.
What's the problem?
When LLMs are updated to improve their performance, they often change in ways that can confuse users. This is because users build a mental model of how the model works, and every time there's an update, they have to adjust their understanding, which can be frustrating. Sometimes, these updates even cause the model to make mistakes on tasks it previously handled well, known as 'negative flips.'
What's the solution?
MUSCLE addresses this problem by introducing methods to evaluate how compatible the new model is with older versions and by training a compatibility model that helps reduce errors during updates. The researchers created metrics to measure compatibility and developed a training strategy that minimizes the chances of the model making mistakes after an update. They showed that this approach reduced negative flips by up to 40% when moving from one version of the model (Llama 1) to another (Llama 2).
Why it matters?
This research is important because it helps ensure that updates to AI models do not disrupt user experience or lead to confusion. By maintaining compatibility, MUSCLE allows for continuous improvements in LLMs while keeping them reliable and user-friendly. This is especially crucial as LLMs become more integrated into everyday applications like chatbots and digital assistants.
Abstract
Large Language Models (LLMs) are frequently updated due to data or architecture changes to improve their performance. When updating models, developers often focus on increasing overall performance metrics with less emphasis on being compatible with previous model versions. However, users often build a mental model of the functionality and capabilities of a particular machine learning model they are interacting with. They have to adapt their mental model with every update -- a draining task that can lead to user dissatisfaction. In practice, fine-tuned downstream task adapters rely on pretrained LLM base models. When these base models are updated, these user-facing downstream task models experience instance regression or negative flips -- previously correct instances are now predicted incorrectly. This happens even when the downstream task training procedures remain identical. Our work aims to provide seamless model updates to a user in two ways. First, we provide evaluation metrics for a notion of compatibility to prior model versions, specifically for generative tasks but also applicable for discriminative tasks. We observe regression and inconsistencies between different model versions on a diverse set of tasks and model updates. Second, we propose a training strategy to minimize the number of inconsistencies in model updates, involving training of a compatibility model that can enhance task fine-tuned language models. We reduce negative flips -- instances where a prior model version was correct, but a new model incorrect -- by up to 40% from Llama 1 to Llama 2.