MusiConGen: Rhythm and Chord Control for Transformer-Based Text-to-Music Generation
Yun-Han Lan, Wen-Yi Hsiao, Hao-Chung Cheng, Yi-Hsuan Yang
2024-07-23
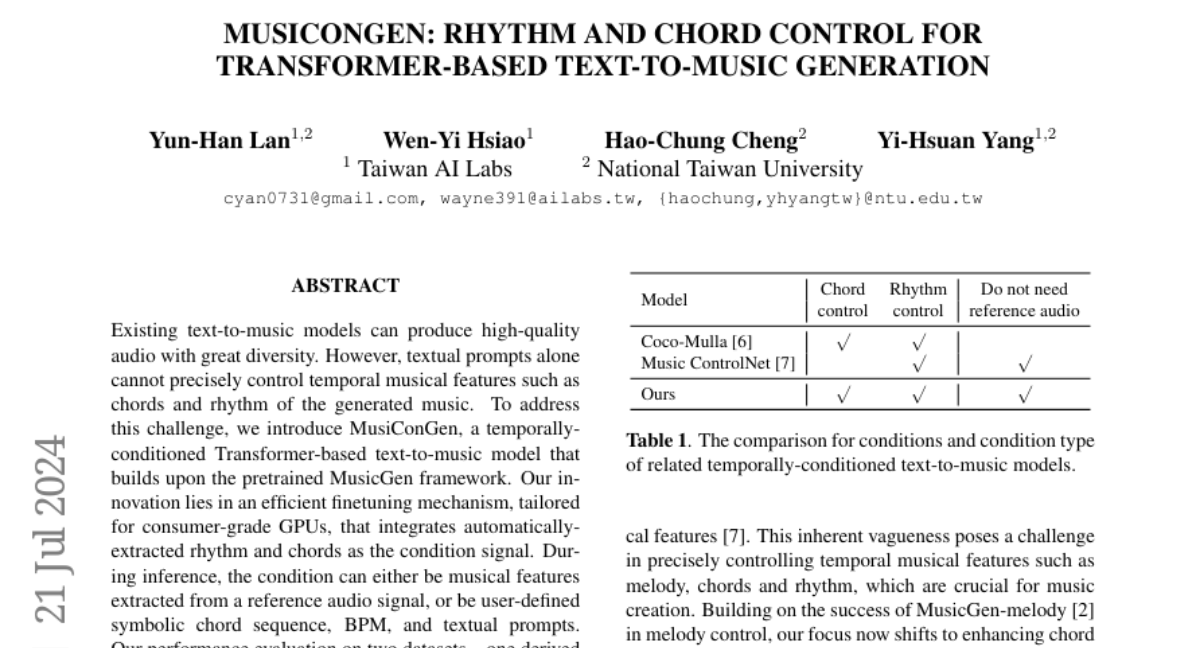
Summary
This paper introduces MusiConGen, a new model that generates music from text descriptions while allowing users to control specific elements like rhythm and chords. It builds on previous models to create more precise and customizable music outputs.
What's the problem?
Many existing models that generate music from text can create a variety of audio, but they often lack the ability to control important musical features such as rhythm and chord progressions. This makes it hard for users to get the exact sound they want based on their descriptions, limiting creativity and customization in music generation.
What's the solution?
MusiConGen addresses this issue by using a Transformer-based approach that incorporates rhythm and chord information directly into the music generation process. It uses a special training method that allows the model to understand and apply these musical features based on user input. Users can provide not only text prompts but also specific details like chord sequences and beats per minute (BPM), which helps the model generate music that aligns closely with their vision. The model has been tested on two datasets, showing improved performance in creating realistic backing tracks that match the specified conditions.
Why it matters?
This research is important because it enhances the capabilities of AI in music generation, making it easier for musicians, composers, and hobbyists to create music that fits their ideas perfectly. By allowing for more control over musical elements, MusiConGen can help foster creativity and innovation in music production, potentially transforming how we approach music composition with technology.
Abstract
Existing text-to-music models can produce high-quality audio with great diversity. However, textual prompts alone cannot precisely control temporal musical features such as chords and rhythm of the generated music. To address this challenge, we introduce MusiConGen, a temporally-conditioned Transformer-based text-to-music model that builds upon the pretrained MusicGen framework. Our innovation lies in an efficient finetuning mechanism, tailored for consumer-grade GPUs, that integrates automatically-extracted rhythm and chords as the condition signal. During inference, the condition can either be musical features extracted from a reference audio signal, or be user-defined symbolic chord sequence, BPM, and textual prompts. Our performance evaluation on two datasets -- one derived from extracted features and the other from user-created inputs -- demonstrates that MusiConGen can generate realistic backing track music that aligns well with the specified conditions. We open-source the code and model checkpoints, and provide audio examples online, https://musicongen.github.io/musicongen_demo/.