MV-Adapter: Multi-view Consistent Image Generation Made Easy
Zehuan Huang, Yuan-Chen Guo, Haoran Wang, Ran Yi, Lizhuang Ma, Yan-Pei Cao, Lu Sheng
2024-12-06
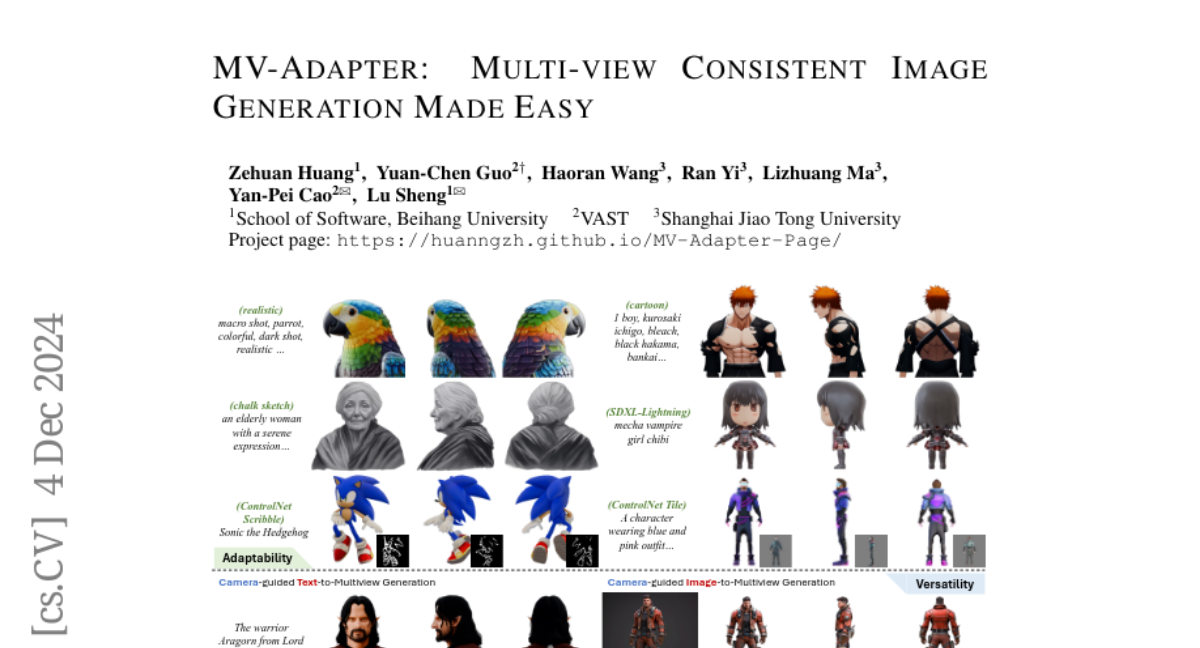
Summary
This paper introduces MV-Adapter, a new method that makes it easier and more efficient to generate images from multiple viewpoints using existing text-to-image models without needing major changes.
What's the problem?
Current methods for generating multi-view images often require significant modifications to pre-trained text-to-image (T2I) models and involve full fine-tuning. This can lead to high computational costs, especially when working with large models and high-resolution images, and can also reduce the quality of the generated images due to optimization challenges and limited high-quality 3D data.
What's the solution?
MV-Adapter addresses these issues by providing a plug-and-play adapter that enhances T2I models without altering their original structure. It updates fewer parameters, which allows for efficient training while preserving the knowledge already embedded in pre-trained models. The adapter uses innovative designs, such as duplicated self-attention layers, to effectively incorporate 3D geometric knowledge. Additionally, it includes a unified condition encoder that integrates camera settings and geometric information, making it versatile for various applications like text- and image-based 3D generation.
Why it matters?
This research is important because it sets a new standard for generating multi-view images efficiently and with high quality. By improving the adaptability and versatility of existing models, MV-Adapter opens up new possibilities for applications in fields like virtual reality, gaming, and content creation, allowing users to create detailed images from multiple perspectives more easily.
Abstract
Existing multi-view image generation methods often make invasive modifications to pre-trained text-to-image (T2I) models and require full fine-tuning, leading to (1) high computational costs, especially with large base models and high-resolution images, and (2) degradation in image quality due to optimization difficulties and scarce high-quality 3D data. In this paper, we propose the first adapter-based solution for multi-view image generation, and introduce MV-Adapter, a versatile plug-and-play adapter that enhances T2I models and their derivatives without altering the original network structure or feature space. By updating fewer parameters, MV-Adapter enables efficient training and preserves the prior knowledge embedded in pre-trained models, mitigating overfitting risks. To efficiently model the 3D geometric knowledge within the adapter, we introduce innovative designs that include duplicated self-attention layers and parallel attention architecture, enabling the adapter to inherit the powerful priors of the pre-trained models to model the novel 3D knowledge. Moreover, we present a unified condition encoder that seamlessly integrates camera parameters and geometric information, facilitating applications such as text- and image-based 3D generation and texturing. MV-Adapter achieves multi-view generation at 768 resolution on Stable Diffusion XL (SDXL), and demonstrates adaptability and versatility. It can also be extended to arbitrary view generation, enabling broader applications. We demonstrate that MV-Adapter sets a new quality standard for multi-view image generation, and opens up new possibilities due to its efficiency, adaptability and versatility.