MVInpainter: Learning Multi-View Consistent Inpainting to Bridge 2D and 3D Editing
Chenjie Cao, Chaohui Yu, Yanwei Fu, Fan Wang, Xiangyang Xue
2024-08-16
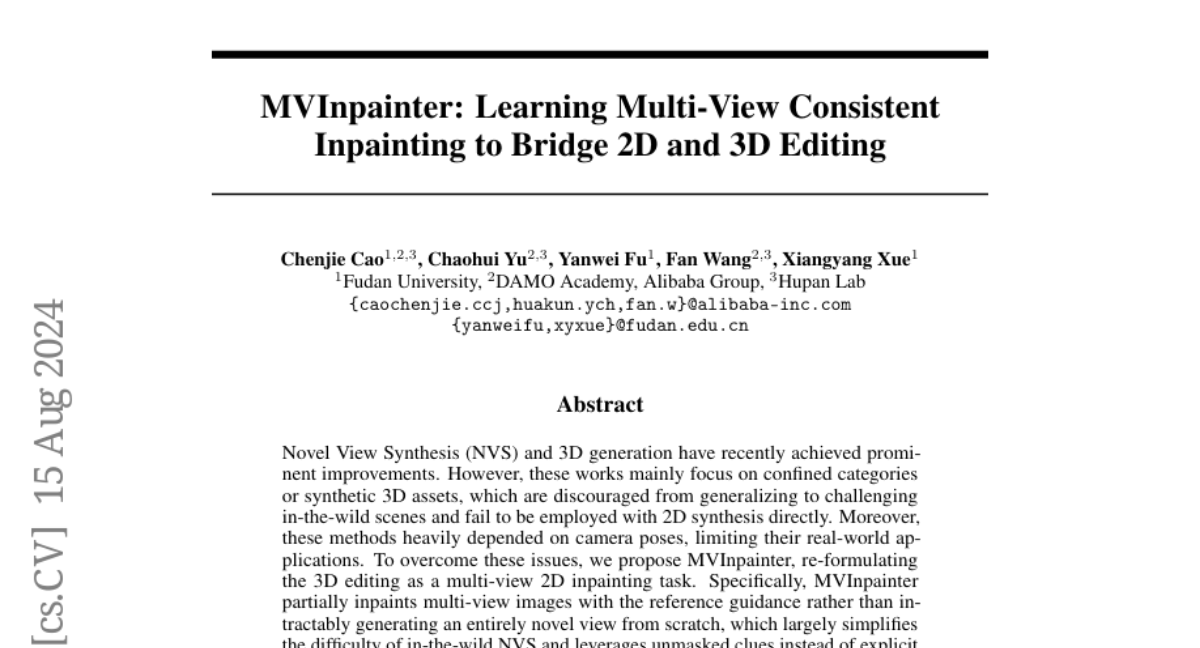
Summary
This paper introduces MVInpainter, a new method for improving the editing of images and scenes by using information from multiple views to fill in missing parts.
What's the problem?
Current methods for generating 3D views from 2D images often struggle with real-world scenes because they rely heavily on specific camera positions and can only handle limited categories. This makes it hard to edit images effectively when the scene is complex or when different views are needed.
What's the solution?
MVInpainter solves this problem by treating the task of editing as a process of filling in missing parts of images taken from different angles. Instead of creating a completely new view from scratch, it uses existing images to guide the editing process. It also incorporates techniques that help maintain consistency across different views and allows for more flexible editing without needing precise camera information.
Why it matters?
This research is important because it makes it easier to edit complex scenes in a way that looks natural and realistic. By bridging the gap between 2D and 3D editing, MVInpainter can be useful in various applications like video games, movies, and virtual reality, where high-quality visuals are essential.
Abstract
Novel View Synthesis (NVS) and 3D generation have recently achieved prominent improvements. However, these works mainly focus on confined categories or synthetic 3D assets, which are discouraged from generalizing to challenging in-the-wild scenes and fail to be employed with 2D synthesis directly. Moreover, these methods heavily depended on camera poses, limiting their real-world applications. To overcome these issues, we propose MVInpainter, re-formulating the 3D editing as a multi-view 2D inpainting task. Specifically, MVInpainter partially inpaints multi-view images with the reference guidance rather than intractably generating an entirely novel view from scratch, which largely simplifies the difficulty of in-the-wild NVS and leverages unmasked clues instead of explicit pose conditions. To ensure cross-view consistency, MVInpainter is enhanced by video priors from motion components and appearance guidance from concatenated reference key&value attention. Furthermore, MVInpainter incorporates slot attention to aggregate high-level optical flow features from unmasked regions to control the camera movement with pose-free training and inference. Sufficient scene-level experiments on both object-centric and forward-facing datasets verify the effectiveness of MVInpainter, including diverse tasks, such as multi-view object removal, synthesis, insertion, and replacement. The project page is https://ewrfcas.github.io/MVInpainter/.