NaRCan: Natural Refined Canonical Image with Integration of Diffusion Prior for Video Editing
Ting-Hsuan Chen, Jiewen Chan, Hau-Shiang Shiu, Shih-Han Yen, Chang-Han Yeh, Yu-Lun Liu
2024-06-13
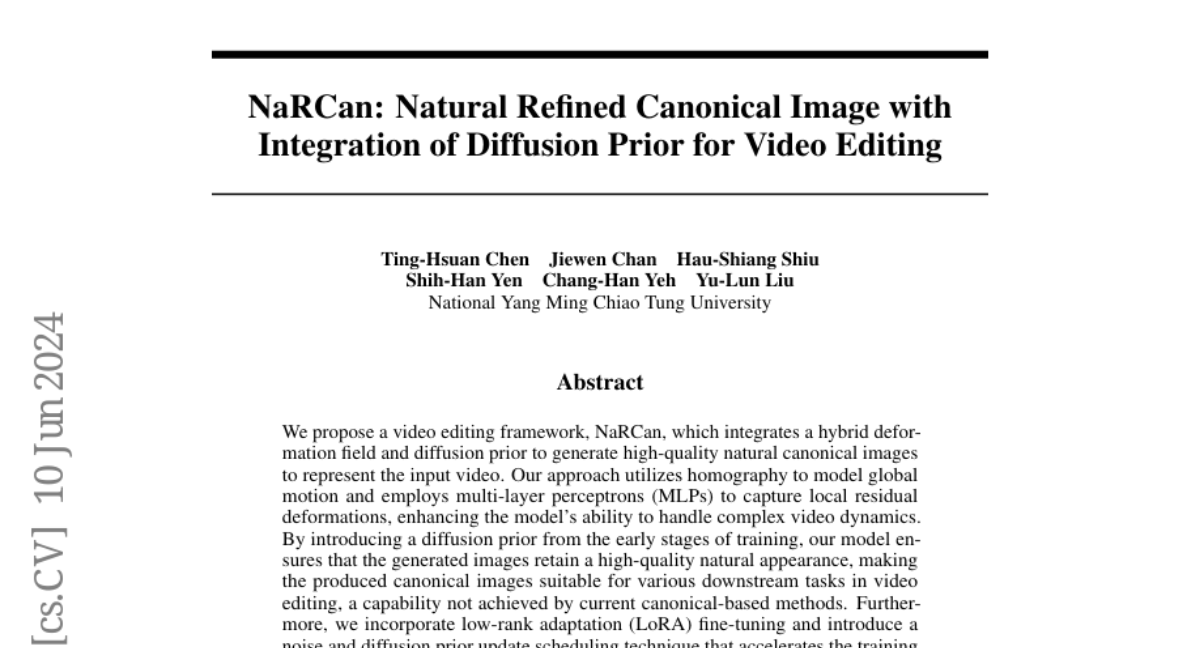
Summary
This paper presents NaRCan, a new video editing framework that uses advanced techniques to create high-quality images from videos. By combining different methods, NaRCan improves how videos are edited, ensuring the final results look natural and consistent.
What's the problem?
Video editing often struggles with maintaining the quality and consistency of images, especially when dealing with complex scenes. Traditional methods can produce poor results due to issues like motion blur or inaccuracies in capturing details. Additionally, existing techniques for creating canonical images (which summarize video content) do not always guarantee that these images will be of high quality.
What's the solution?
The authors developed NaRCan, which integrates a hybrid deformation field and diffusion prior to generate better canonical images. They use homography to track overall motion in the video and multi-layer perceptrons (MLPs) to manage smaller, detailed movements. By starting with a diffusion prior early in the training process, NaRCan ensures that the images produced look natural. They also implemented a technique called low-rank adaptation (LoRA) to speed up training by 14 times and tested their method extensively to confirm its effectiveness.
Why it matters?
NaRCan is important because it addresses major challenges in video editing by producing high-quality images that maintain temporal consistency. This means that when edits are made, the changes appear smooth and realistic across the entire video. The advancements made by NaRCan can significantly enhance various video editing tasks, making it a valuable tool for creators in film, animation, and other visual media.
Abstract
We propose a video editing framework, NaRCan, which integrates a hybrid deformation field and diffusion prior to generate high-quality natural canonical images to represent the input video. Our approach utilizes homography to model global motion and employs multi-layer perceptrons (MLPs) to capture local residual deformations, enhancing the model's ability to handle complex video dynamics. By introducing a diffusion prior from the early stages of training, our model ensures that the generated images retain a high-quality natural appearance, making the produced canonical images suitable for various downstream tasks in video editing, a capability not achieved by current canonical-based methods. Furthermore, we incorporate low-rank adaptation (LoRA) fine-tuning and introduce a noise and diffusion prior update scheduling technique that accelerates the training process by 14 times. Extensive experimental results show that our method outperforms existing approaches in various video editing tasks and produces coherent and high-quality edited video sequences. See our project page for video results at https://koi953215.github.io/NaRCan_page/.