NaturalBench: Evaluating Vision-Language Models on Natural Adversarial Samples
Baiqi Li, Zhiqiu Lin, Wenxuan Peng, Jean de Dieu Nyandwi, Daniel Jiang, Zixian Ma, Simran Khanuja, Ranjay Krishna, Graham Neubig, Deva Ramanan
2024-10-21
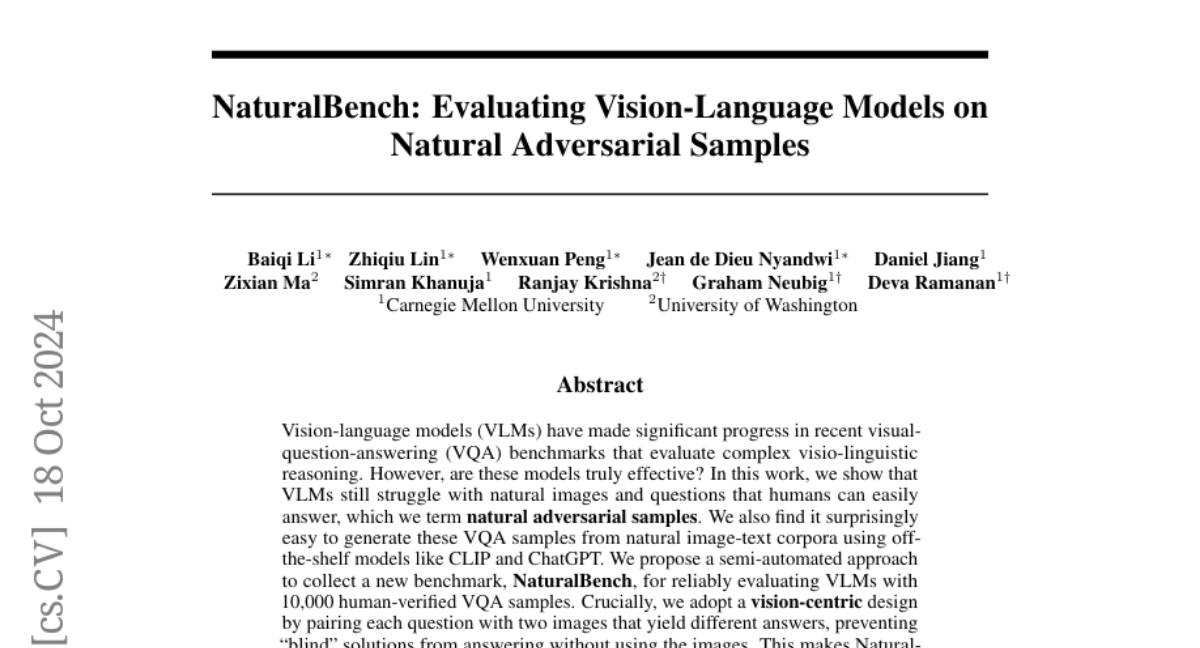
Summary
This paper introduces NaturalBench, a new benchmark designed to evaluate how well vision-language models (VLMs) can answer questions about images using natural adversarial samples.
What's the problem?
Even though vision-language models like CLIP have improved in answering questions about images, they still struggle with natural images and questions that humans find easy. This is a problem because it shows that these models don't fully understand the content they are analyzing. The authors identify these challenging scenarios as 'natural adversarial samples' and note that it's surprisingly easy to create these samples using existing models.
What's the solution?
To address this issue, the authors developed NaturalBench, a benchmark that includes 10,000 human-verified question-and-answer pairs based on natural images. They designed this benchmark to be more challenging by pairing each question with two different images that lead to different answers, ensuring that the models can't just rely on common sense to answer correctly. They tested 53 state-of-the-art VLMs on NaturalBench and found that many of them performed significantly worse than humans, highlighting the limitations of current models. The authors also analyzed why NaturalBench is difficult by looking at the need for diverse skills and exposing biases in the models.
Why it matters?
This research is important because it provides a more rigorous way to evaluate vision-language models, ensuring they can handle real-world scenarios effectively. By highlighting the weaknesses of these models through NaturalBench, researchers can work on improving them, which could lead to better AI systems for applications like image recognition, automated customer service, and more.
Abstract
Vision-language models (VLMs) have made significant progress in recent visual-question-answering (VQA) benchmarks that evaluate complex visio-linguistic reasoning. However, are these models truly effective? In this work, we show that VLMs still struggle with natural images and questions that humans can easily answer, which we term natural adversarial samples. We also find it surprisingly easy to generate these VQA samples from natural image-text corpora using off-the-shelf models like CLIP and ChatGPT. We propose a semi-automated approach to collect a new benchmark, NaturalBench, for reliably evaluating VLMs with 10,000 human-verified VQA samples. Crucially, we adopt a vision-centric design by pairing each question with two images that yield different answers, preventing blind solutions from answering without using the images. This makes NaturalBench more challenging than previous benchmarks that can be solved with commonsense priors. We evaluate 53 state-of-the-art VLMs on NaturalBench, showing that models like LLaVA-OneVision, Cambrian-1, Llama3.2-Vision, Molmo, Qwen2-VL, and even GPT-4o lag 50%-70% behind human performance (over 90%). We analyze why NaturalBench is hard from two angles: (1) Compositionality: Solving NaturalBench requires diverse visio-linguistic skills, including understanding attribute bindings, object relationships, and advanced reasoning like logic and counting. To this end, unlike prior work that uses a single tag per sample, we tag each NaturalBench sample with 1 to 8 skill tags for fine-grained evaluation. (2) Biases: NaturalBench exposes severe biases in VLMs, as models often choose the same answer regardless of the image. Lastly, we apply our benchmark curation method to diverse data sources, including long captions (over 100 words) and non-English languages like Chinese and Hindi, highlighting its potential for dynamic evaluations of VLMs.