NavGPT-2: Unleashing Navigational Reasoning Capability for Large Vision-Language Models
Gengze Zhou, Yicong Hong, Zun Wang, Xin Eric Wang, Qi Wu
2024-07-18
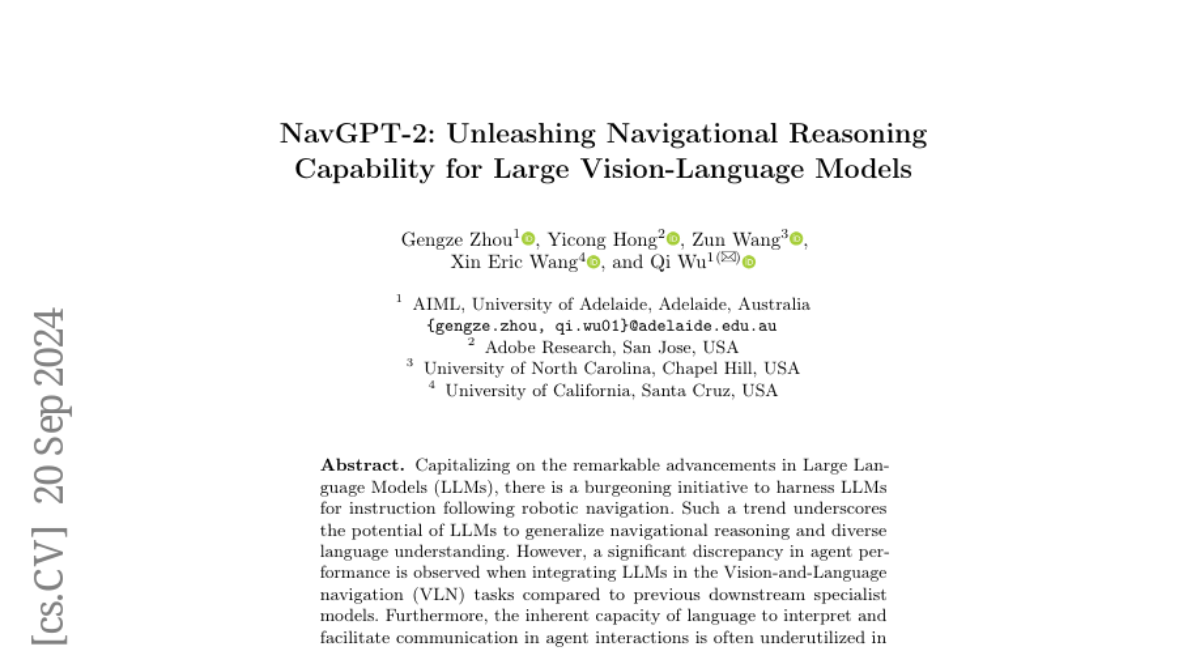
Summary
This paper presents NavGPT-2, a system that enhances the ability of large vision-language models to navigate and understand environments by combining visual information with language instructions.
What's the problem?
While large language models (LLMs) have made great strides in understanding and generating text, they struggle when it comes to navigating real-world environments based on visual cues. Existing models specifically designed for navigation often perform better than LLMs, but they don't take full advantage of the powerful language capabilities of LLMs. This creates a gap in performance when trying to integrate these two types of models for tasks like robotic navigation.
What's the solution?
NavGPT-2 bridges this gap by combining the strengths of vision-language models (VLMs) and navigation-specific models. It uses a frozen LLM to interpret visual information and generate navigational reasoning based on both images and text instructions. The system incorporates advanced techniques to process visual observations and align them with language commands, allowing it to predict effective actions for navigation. This approach improves data efficiency and enhances the model's ability to understand complex environments, making it more effective than previous methods.
Why it matters?
This research is significant because it improves how robots and AI systems can navigate real-world settings by using both visual and language inputs. By enhancing the navigational reasoning capabilities of LLMs, NavGPT-2 can lead to more intelligent and adaptable robotic systems that can better assist in various applications, such as autonomous vehicles, delivery robots, and smart home assistants.
Abstract
Capitalizing on the remarkable advancements in Large Language Models (LLMs), there is a burgeoning initiative to harness LLMs for instruction following robotic navigation. Such a trend underscores the potential of LLMs to generalize navigational reasoning and diverse language understanding. However, a significant discrepancy in agent performance is observed when integrating LLMs in the Vision-and-Language navigation (VLN) tasks compared to previous downstream specialist models. Furthermore, the inherent capacity of language to interpret and facilitate communication in agent interactions is often underutilized in these integrations. In this work, we strive to bridge the divide between VLN-specialized models and LLM-based navigation paradigms, while maintaining the interpretative prowess of LLMs in generating linguistic navigational reasoning. By aligning visual content in a frozen LLM, we encompass visual observation comprehension for LLMs and exploit a way to incorporate LLMs and navigation policy networks for effective action predictions and navigational reasoning. We demonstrate the data efficiency of the proposed methods and eliminate the gap between LM-based agents and state-of-the-art VLN specialists.