NeCo: Improving DINOv2's spatial representations in 19 GPU hours with Patch Neighbor Consistency
Valentinos Pariza, Mohammadreza Salehi, Gertjan Burghouts, Francesco Locatello, Yuki M. Asano
2024-08-21
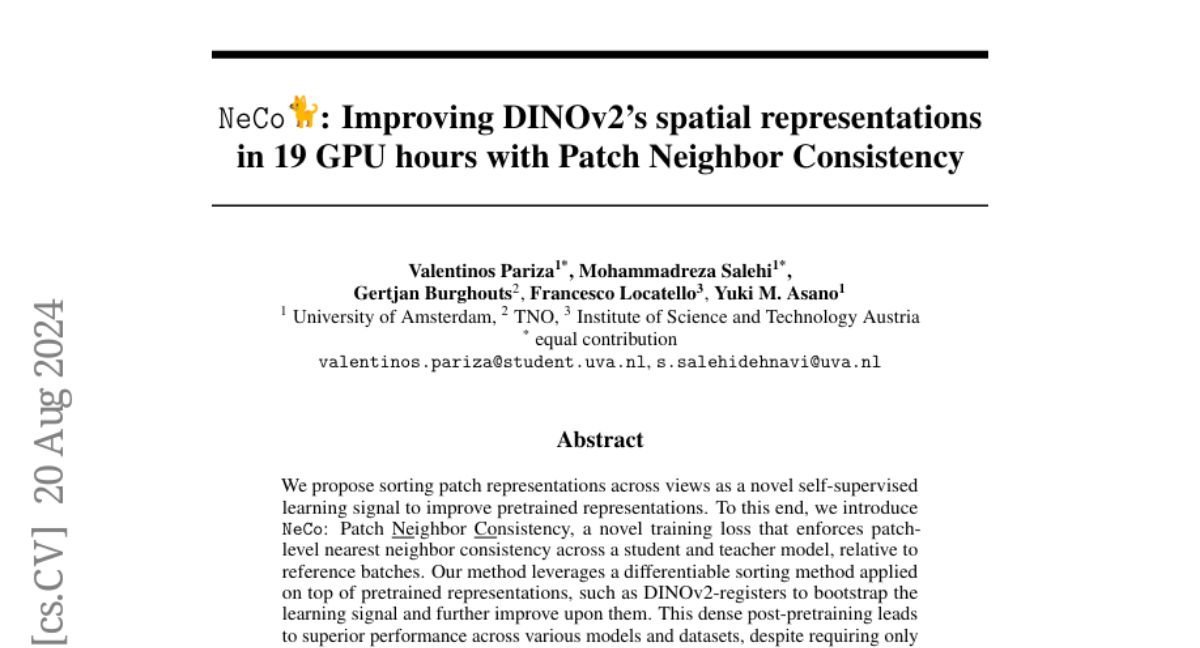
Summary
This paper discusses NeCo, a method that improves the performance of a model called DINOv2 by using a new training technique called Patch Neighbor Consistency.
What's the problem?
While models like DINOv2 have shown good results in understanding images, there is still room for improvement in how well they represent different parts of an image. Existing methods often require a lot of time and resources to enhance these representations effectively.
What's the solution?
NeCo introduces a new way to train models by sorting the representations of image patches (small sections of an image) across different views. This technique uses a special loss function that ensures similar patches are treated consistently between two models: a 'teacher' model that has already learned and a 'student' model that is learning. By doing this, NeCo can significantly improve the quality of the model's output in just 19 hours on a single GPU, leading to better performance in various tasks like semantic segmentation.
Why it matters?
This research is important because it shows how effective training methods can lead to better image understanding without requiring massive amounts of time or data. By improving how models learn from images, we can enhance applications in fields like computer vision, which is essential for technologies such as self-driving cars and medical imaging.
Abstract
We propose sorting patch representations across views as a novel self-supervised learning signal to improve pretrained representations. To this end, we introduce NeCo: Patch Neighbor Consistency, a novel training loss that enforces patch-level nearest neighbor consistency across a student and teacher model, relative to reference batches. Our method leverages a differentiable sorting method applied on top of pretrained representations, such as DINOv2-registers to bootstrap the learning signal and further improve upon them. This dense post-pretraining leads to superior performance across various models and datasets, despite requiring only 19 hours on a single GPU. We demonstrate that this method generates high-quality dense feature encoders and establish several new state-of-the-art results: +5.5% and + 6% for non-parametric in-context semantic segmentation on ADE20k and Pascal VOC, and +7.2% and +5.7% for linear segmentation evaluations on COCO-Things and -Stuff.