NeKo: Toward Post Recognition Generative Correction Large Language Models with Task-Oriented Experts
Yen-Ting Lin, Chao-Han Huck Yang, Zhehuai Chen, Piotr Zelasko, Xuesong Yang, Zih-Ching Chen, Krishna C Puvvada, Szu-Wei Fu, Ke Hu, Jun Wei Chiu, Jagadeesh Balam, Boris Ginsburg, Yu-Chiang Frank Wang
2024-11-12
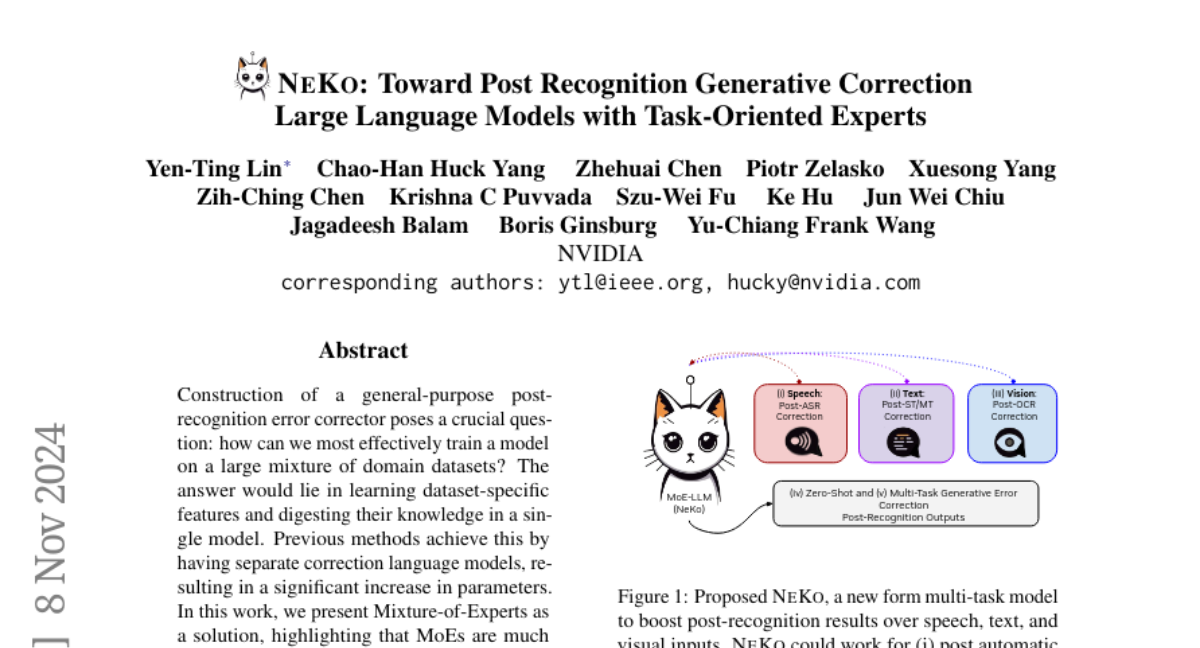
Summary
This paper introduces NeKo, a new model designed to correct errors in text generated from various recognition systems, using a method called Mixture-of-Experts (MoE) to improve accuracy across different tasks.
What's the problem?
When we use systems that convert speech or images to text, they can make mistakes. Existing models for correcting these errors often require a lot of resources and end up being too large and complicated. This makes it difficult to create a single model that can effectively handle different types of data, like speech, text, and images, without needing separate models for each task.
What's the solution?
NeKo uses a Mixture-of-Experts approach, where different 'experts' within the model specialize in correcting errors for specific types of data (like speech-to-text or image-to-text). This way, the model can learn from various datasets without becoming overly complex. The authors tested NeKo on different benchmarks and found that it significantly reduced error rates in generated text compared to previous models. For instance, it achieved better results in speech recognition and translation tasks, showing improvements in accuracy when correcting errors.
Why it matters?
This research is important because it provides a more efficient and effective way to correct errors in text generated from different sources. By using a single model that can adapt to various tasks, NeKo can help improve the reliability of automated systems in real-world applications, such as voice assistants and translation services. This could lead to better user experiences and more accurate communication across different languages and formats.
Abstract
Construction of a general-purpose post-recognition error corrector poses a crucial question: how can we most effectively train a model on a large mixture of domain datasets? The answer would lie in learning dataset-specific features and digesting their knowledge in a single model. Previous methods achieve this by having separate correction language models, resulting in a significant increase in parameters. In this work, we present Mixture-of-Experts as a solution, highlighting that MoEs are much more than a scalability tool. We propose a Multi-Task Correction MoE, where we train the experts to become an ``expert'' of speech-to-text, language-to-text and vision-to-text datasets by learning to route each dataset's tokens to its mapped expert. Experiments on the Open ASR Leaderboard show that we explore a new state-of-the-art performance by achieving an average relative 5.0% WER reduction and substantial improvements in BLEU scores for speech and translation tasks. On zero-shot evaluation, NeKo outperforms GPT-3.5 and Claude-Opus with 15.5% to 27.6% relative WER reduction in the Hyporadise benchmark. NeKo performs competitively on grammar and post-OCR correction as a multi-task model.