Neural Fields in Robotics: A Survey
Muhammad Zubair Irshad, Mauro Comi, Yen-Chen Lin, Nick Heppert, Abhinav Valada, Rares Ambrus, Zsolt Kira, Jonathan Tremblay
2024-10-29
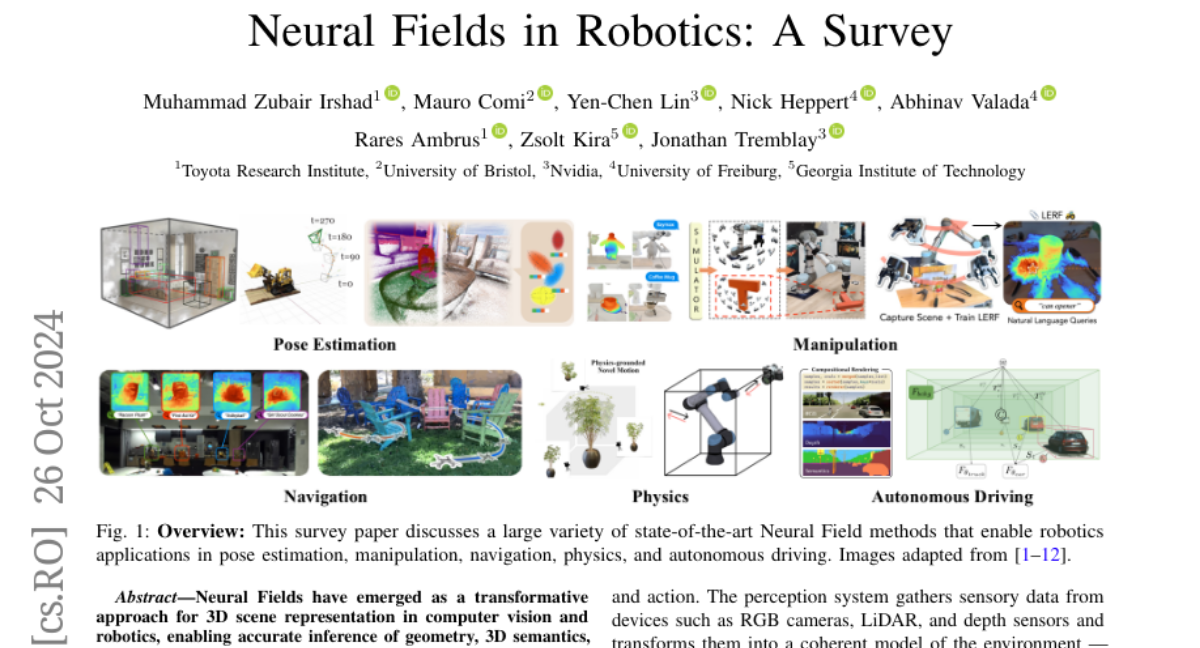
Summary
This paper surveys the use of Neural Fields in robotics, explaining how they can help robots understand and interact with 3D environments more effectively.
What's the problem?
Robots often need to understand complex 3D scenes to perform tasks like navigating or manipulating objects. Traditional methods for representing 3D scenes can be limited and may not capture all the necessary details. This makes it difficult for robots to accurately perceive their surroundings and make informed decisions.
What's the solution?
The authors discuss Neural Fields, a new approach that allows for better representation of 3D scenes by using advanced techniques to infer geometry, semantics, and dynamics from 2D data. They review different types of Neural Fields, such as Occupancy Networks and Neural Radiance Fields, and explain how these methods can improve various robotic applications like navigation and object manipulation. By integrating these models with existing technologies, robots can gain a clearer understanding of their environments.
Why it matters?
This research is important because it highlights how advanced AI techniques can enhance robotic capabilities. By improving how robots perceive and interact with the world, we can enable them to perform more complex tasks, leading to advancements in fields like autonomous driving, manufacturing, and service industries.
Abstract
Neural Fields have emerged as a transformative approach for 3D scene representation in computer vision and robotics, enabling accurate inference of geometry, 3D semantics, and dynamics from posed 2D data. Leveraging differentiable rendering, Neural Fields encompass both continuous implicit and explicit neural representations enabling high-fidelity 3D reconstruction, integration of multi-modal sensor data, and generation of novel viewpoints. This survey explores their applications in robotics, emphasizing their potential to enhance perception, planning, and control. Their compactness, memory efficiency, and differentiability, along with seamless integration with foundation and generative models, make them ideal for real-time applications, improving robot adaptability and decision-making. This paper provides a thorough review of Neural Fields in robotics, categorizing applications across various domains and evaluating their strengths and limitations, based on over 200 papers. First, we present four key Neural Fields frameworks: Occupancy Networks, Signed Distance Fields, Neural Radiance Fields, and Gaussian Splatting. Second, we detail Neural Fields' applications in five major robotics domains: pose estimation, manipulation, navigation, physics, and autonomous driving, highlighting key works and discussing takeaways and open challenges. Finally, we outline the current limitations of Neural Fields in robotics and propose promising directions for future research. Project page: https://robonerf.github.io