NitroFusion: High-Fidelity Single-Step Diffusion through Dynamic Adversarial Training
Dar-Yen Chen, Hmrishav Bandyopadhyay, Kai Zou, Yi-Zhe Song
2024-12-05
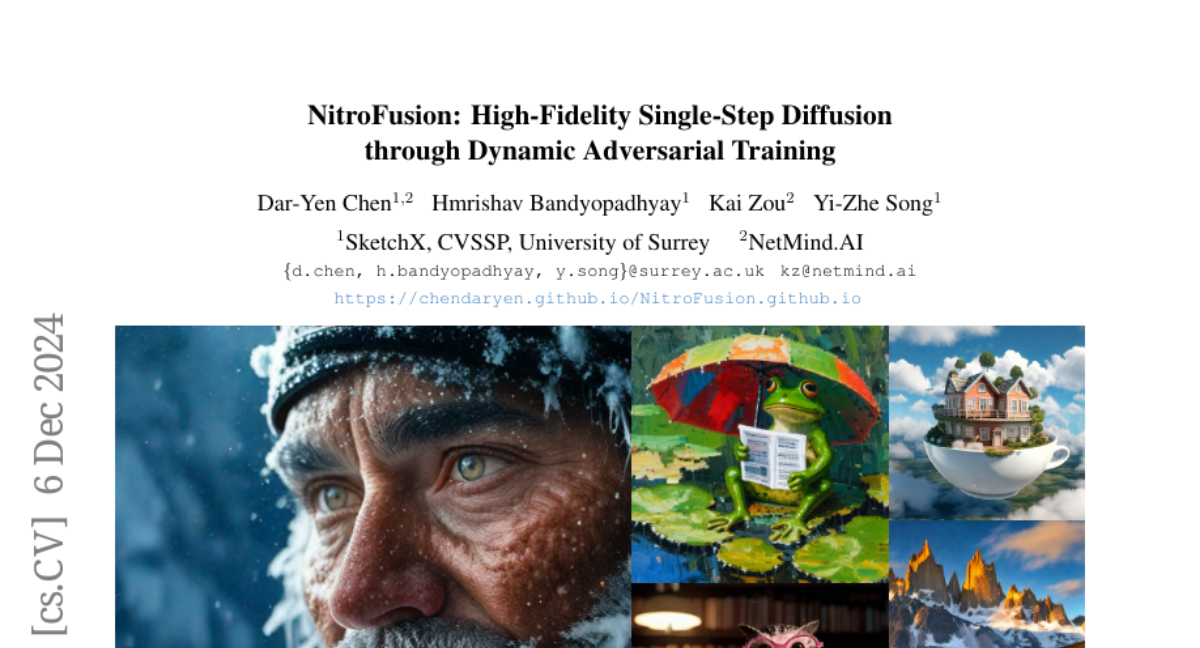
Summary
This paper introduces NitroFusion, a new method for generating high-quality images quickly using a single-step diffusion process through dynamic adversarial training.
What's the problem?
While one-step diffusion methods for generating images are much faster than traditional multi-step methods, they often produce lower quality images. This is a problem because users want both speed and high-quality results when creating images with AI. Existing methods struggle to balance these needs, leading to poor performance in generating detailed and coherent images.
What's the solution?
NitroFusion addresses this issue by using a dynamic adversarial framework that includes a large pool of specialized discriminators. These discriminators provide feedback on different aspects of the generated images, similar to how art critics focus on various elements like color and composition. The method employs techniques such as a dynamic pool of discriminators that refreshes periodically to avoid overfitting and uses global-local discriminator heads for assessing image quality at different scales. This allows NitroFusion to generate high-fidelity images in just one step while maintaining quality.
Why it matters?
This research is important because it significantly improves the efficiency of image generation technologies. By enabling high-quality image creation in a single step, NitroFusion can enhance applications in areas like video games, virtual reality, and digital art, where quick and visually appealing results are essential. This advancement could lead to broader use of AI in creative fields, making it easier for artists and developers to produce stunning visuals.
Abstract
We introduce NitroFusion, a fundamentally different approach to single-step diffusion that achieves high-quality generation through a dynamic adversarial framework. While one-step methods offer dramatic speed advantages, they typically suffer from quality degradation compared to their multi-step counterparts. Just as a panel of art critics provides comprehensive feedback by specializing in different aspects like composition, color, and technique, our approach maintains a large pool of specialized discriminator heads that collectively guide the generation process. Each discriminator group develops expertise in specific quality aspects at different noise levels, providing diverse feedback that enables high-fidelity one-step generation. Our framework combines: (i) a dynamic discriminator pool with specialized discriminator groups to improve generation quality, (ii) strategic refresh mechanisms to prevent discriminator overfitting, and (iii) global-local discriminator heads for multi-scale quality assessment, and unconditional/conditional training for balanced generation. Additionally, our framework uniquely supports flexible deployment through bottom-up refinement, allowing users to dynamically choose between 1-4 denoising steps with the same model for direct quality-speed trade-offs. Through comprehensive experiments, we demonstrate that NitroFusion significantly outperforms existing single-step methods across multiple evaluation metrics, particularly excelling in preserving fine details and global consistency.