NL-Eye: Abductive NLI for Images
Mor Ventura, Michael Toker, Nitay Calderon, Zorik Gekhman, Yonatan Bitton, Roi Reichart
2024-10-07
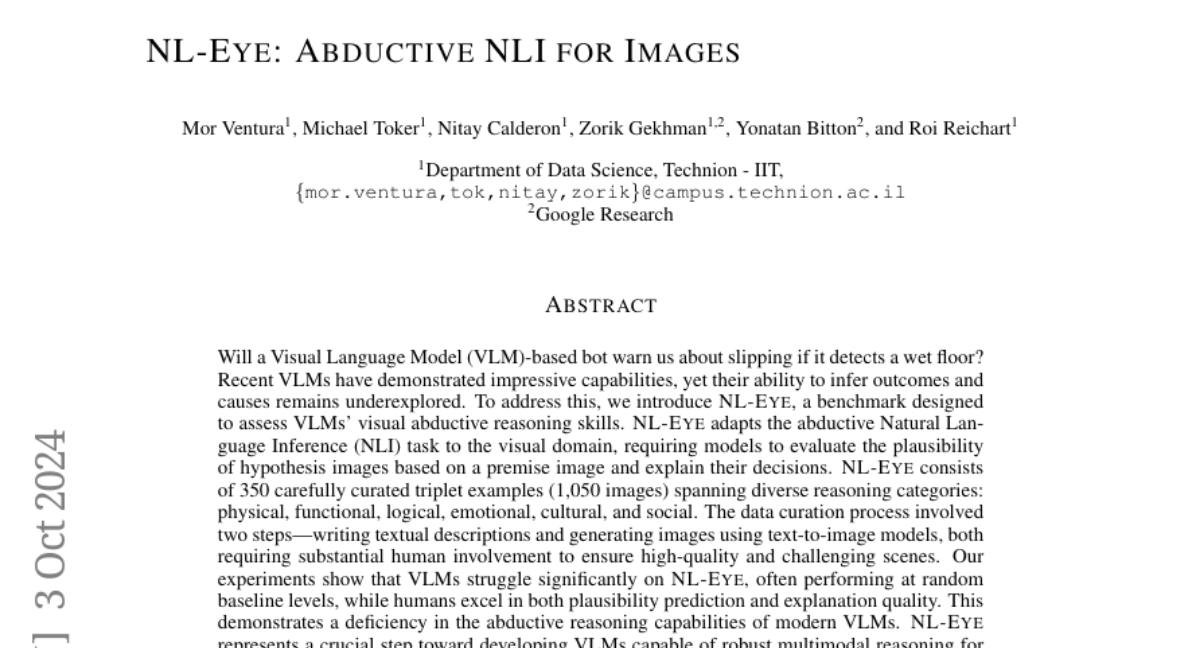
Summary
This paper presents NL-Eye, a new benchmark designed to evaluate how well visual language models (VLMs) can make inferences about images, specifically focusing on their ability to understand causes and effects.
What's the problem?
While recent VLMs have shown impressive capabilities in understanding images and text, they often struggle with reasoning about the relationships between different elements in an image. For example, if a VLM sees a wet floor, it may not effectively infer that someone might slip on it. This lack of reasoning ability limits their effectiveness in real-world applications where understanding context is crucial.
What's the solution?
To tackle this problem, the authors created NL-Eye, which adapts the concept of abductive reasoning (inferring the most likely explanation for an observation) to the visual domain. The benchmark consists of 350 examples with 1,050 images that require VLMs to evaluate the plausibility of different scenarios based on a given image. The data was carefully curated to include various reasoning categories such as physical and social contexts. The study found that VLMs performed poorly in these tasks, demonstrating that they often guess rather than reason correctly, while humans excelled at making plausible predictions and providing quality explanations.
Why it matters?
This research is important because it highlights the current limitations of VLMs in understanding complex visual scenarios. By establishing NL-Eye as a benchmark, the authors aim to push forward the development of VLMs that can better reason about images, which is essential for applications like safety alerts (e.g., warning about wet floors) and verifying video content. Improving these models could lead to more reliable AI systems that understand and respond appropriately to real-world situations.
Abstract
Will a Visual Language Model (VLM)-based bot warn us about slipping if it detects a wet floor? Recent VLMs have demonstrated impressive capabilities, yet their ability to infer outcomes and causes remains underexplored. To address this, we introduce NL-Eye, a benchmark designed to assess VLMs' visual abductive reasoning skills. NL-Eye adapts the abductive Natural Language Inference (NLI) task to the visual domain, requiring models to evaluate the plausibility of hypothesis images based on a premise image and explain their decisions. NL-Eye consists of 350 carefully curated triplet examples (1,050 images) spanning diverse reasoning categories: physical, functional, logical, emotional, cultural, and social. The data curation process involved two steps - writing textual descriptions and generating images using text-to-image models, both requiring substantial human involvement to ensure high-quality and challenging scenes. Our experiments show that VLMs struggle significantly on NL-Eye, often performing at random baseline levels, while humans excel in both plausibility prediction and explanation quality. This demonstrates a deficiency in the abductive reasoning capabilities of modern VLMs. NL-Eye represents a crucial step toward developing VLMs capable of robust multimodal reasoning for real-world applications, including accident-prevention bots and generated video verification.