Not All Prompts Are Made Equal: Prompt-based Pruning of Text-to-Image Diffusion Models
Alireza Ganjdanesh, Reza Shirkavand, Shangqian Gao, Heng Huang
2024-06-19
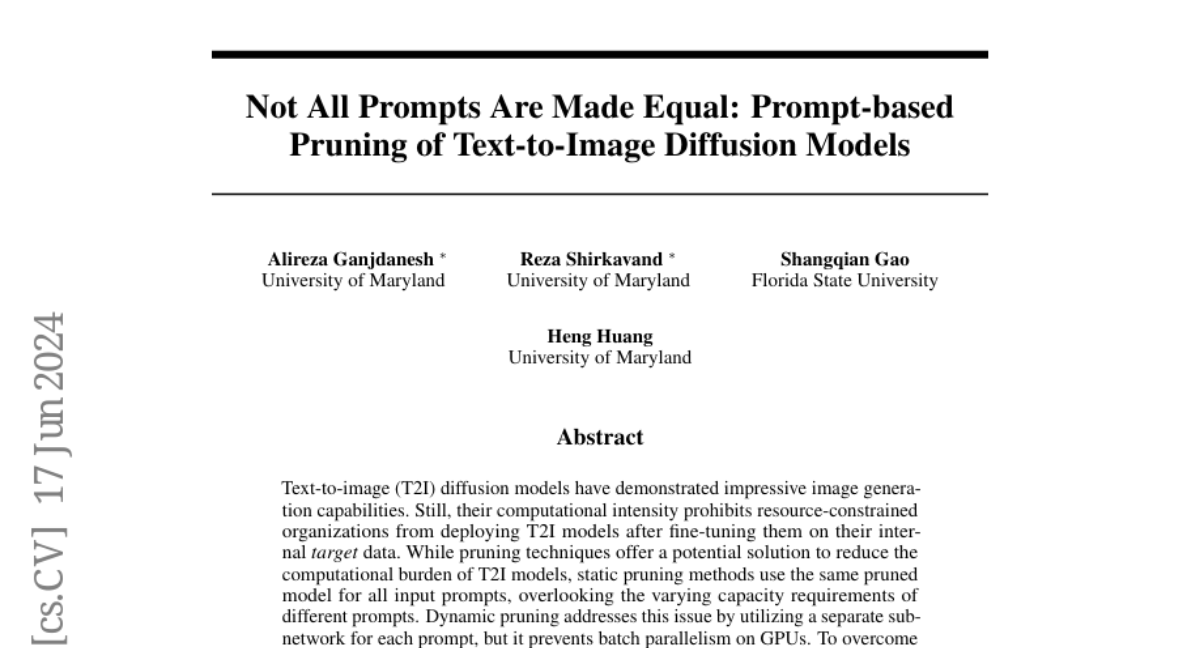
Summary
This paper discusses a new method called Adaptive Prompt-Tailored Pruning (APTP) designed to improve how text-to-image diffusion models generate images based on text prompts. The goal is to make these models more efficient and easier to use, especially for organizations with limited computing resources.
What's the problem?
Text-to-image diffusion models are powerful tools for creating images from text descriptions, but they require a lot of computational power, making them hard to use for organizations that don't have access to high-end hardware. Current methods for reducing the computational load, like pruning, often treat all prompts the same, which doesn't take into account that different prompts might need different amounts of processing power. This can lead to inefficiencies and wasted resources.
What's the solution?
To solve this problem, the authors developed APTP, which uses a system called a prompt router. This router learns how much processing power each text prompt needs and directs it to a specific model configuration that can handle that requirement. Instead of using a one-size-fits-all approach, APTP tailors the model's resources based on the complexity of the prompt. The authors trained this system using advanced techniques to ensure that similar prompts are grouped together effectively. They tested APTP with a popular model called Stable Diffusion and found that it performed better than existing methods in generating high-quality images.
Why it matters?
This research is important because it makes advanced image generation technology more accessible by improving its efficiency. By allowing models to adapt their processing power based on the specific needs of different prompts, APTP could help smaller organizations or individuals use powerful AI tools without needing expensive hardware. This could lead to more creative applications in fields like art, advertising, and education, where generating images from text descriptions is valuable.
Abstract
Text-to-image (T2I) diffusion models have demonstrated impressive image generation capabilities. Still, their computational intensity prohibits resource-constrained organizations from deploying T2I models after fine-tuning them on their internal target data. While pruning techniques offer a potential solution to reduce the computational burden of T2I models, static pruning methods use the same pruned model for all input prompts, overlooking the varying capacity requirements of different prompts. Dynamic pruning addresses this issue by utilizing a separate sub-network for each prompt, but it prevents batch parallelism on GPUs. To overcome these limitations, we introduce Adaptive Prompt-Tailored Pruning (APTP), a novel prompt-based pruning method designed for T2I diffusion models. Central to our approach is a prompt router model, which learns to determine the required capacity for an input text prompt and routes it to an architecture code, given a total desired compute budget for prompts. Each architecture code represents a specialized model tailored to the prompts assigned to it, and the number of codes is a hyperparameter. We train the prompt router and architecture codes using contrastive learning, ensuring that similar prompts are mapped to nearby codes. Further, we employ optimal transport to prevent the codes from collapsing into a single one. We demonstrate APTP's effectiveness by pruning Stable Diffusion (SD) V2.1 using CC3M and COCO as target datasets. APTP outperforms the single-model pruning baselines in terms of FID, CLIP, and CMMD scores. Our analysis of the clusters learned by APTP reveals they are semantically meaningful. We also show that APTP can automatically discover previously empirically found challenging prompts for SD, e.g., prompts for generating text images, assigning them to higher capacity codes.