Number it: Temporal Grounding Videos like Flipping Manga
Yongliang Wu, Xinting Hu, Yuyang Sun, Yizhou Zhou, Wenbo Zhu, Fengyun Rao, Bernt Schiele, Xu Yang
2024-11-18
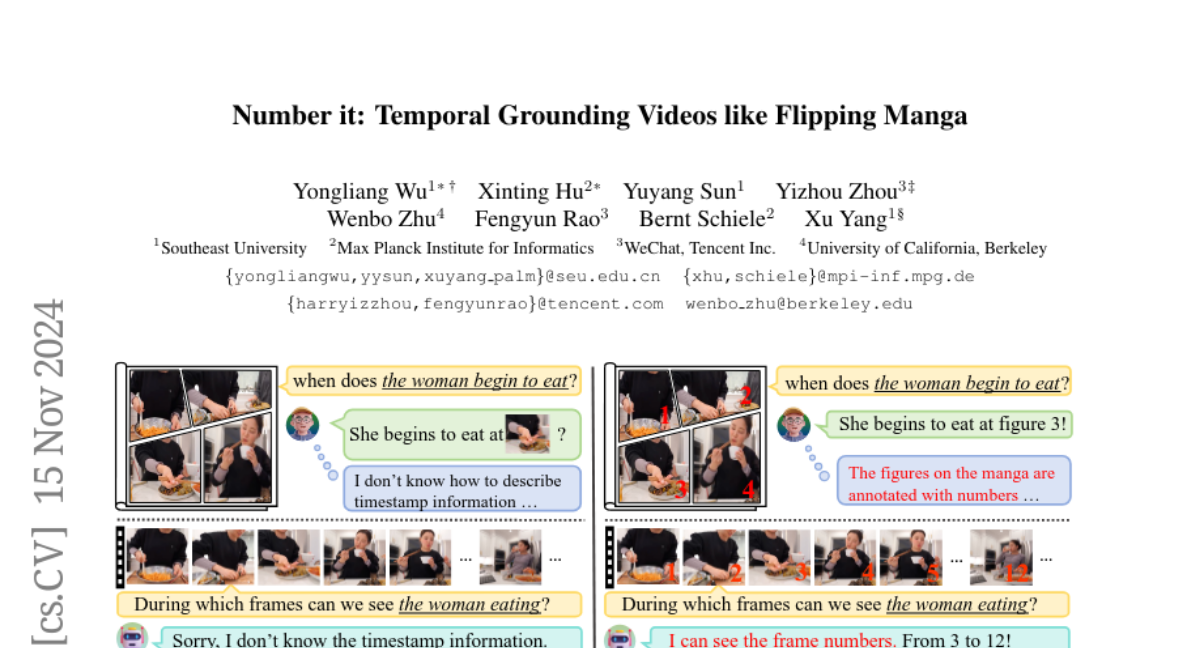
Summary
This paper introduces Xmodel-1.5, a new multilingual large language model (LLM) with 1 billion parameters, designed to perform well in various languages, including Thai, Arabic, and French.
What's the problem?
As language models grow larger and more complex, they often struggle to effectively understand and generate text in multiple languages. Existing models may not perform well on less common languages or specific tasks, which limits their usability in real-world applications.
What's the solution?
Xmodel-1.5 was trained on approximately 2 trillion tokens of text, which helps it understand and generate language more effectively. The model shows strong performance across several languages and includes a specially created evaluation dataset for Thai, developed with input from students to assess the model's capabilities. This dataset helps researchers understand how well the model performs in practical scenarios.
Why it matters?
This research is important because it contributes to the development of multilingual AI systems that can improve communication across different languages. By enhancing the performance of language models in less commonly spoken languages, Xmodel-1.5 aims to support better understanding and interaction in diverse linguistic contexts, which is crucial for global communication.
Abstract
Video Large Language Models (Vid-LLMs) have made remarkable advancements in comprehending video content for QA dialogue. However, they struggle to extend this visual understanding to tasks requiring precise temporal localization, known as Video Temporal Grounding (VTG). To address this gap, we introduce Number-Prompt (NumPro), a novel method that empowers Vid-LLMs to bridge visual comprehension with temporal grounding by adding unique numerical identifiers to each video frame. Treating a video as a sequence of numbered frame images, NumPro transforms VTG into an intuitive process: flipping through manga panels in sequence. This allows Vid-LLMs to "read" event timelines, accurately linking visual content with corresponding temporal information. Our experiments demonstrate that NumPro significantly boosts VTG performance of top-tier Vid-LLMs without additional computational cost. Furthermore, fine-tuning on a NumPro-enhanced dataset defines a new state-of-the-art for VTG, surpassing previous top-performing methods by up to 6.9\% in mIoU for moment retrieval and 8.5\% in mAP for highlight detection. The code will be available at https://github.com/yongliang-wu/NumPro.