NVLM: Open Frontier-Class Multimodal LLMs
Wenliang Dai, Nayeon Lee, Boxin Wang, Zhuoling Yang, Zihan Liu, Jon Barker, Tuomas Rintamaki, Mohammad Shoeybi, Bryan Catanzaro, Wei Ping
2024-09-18
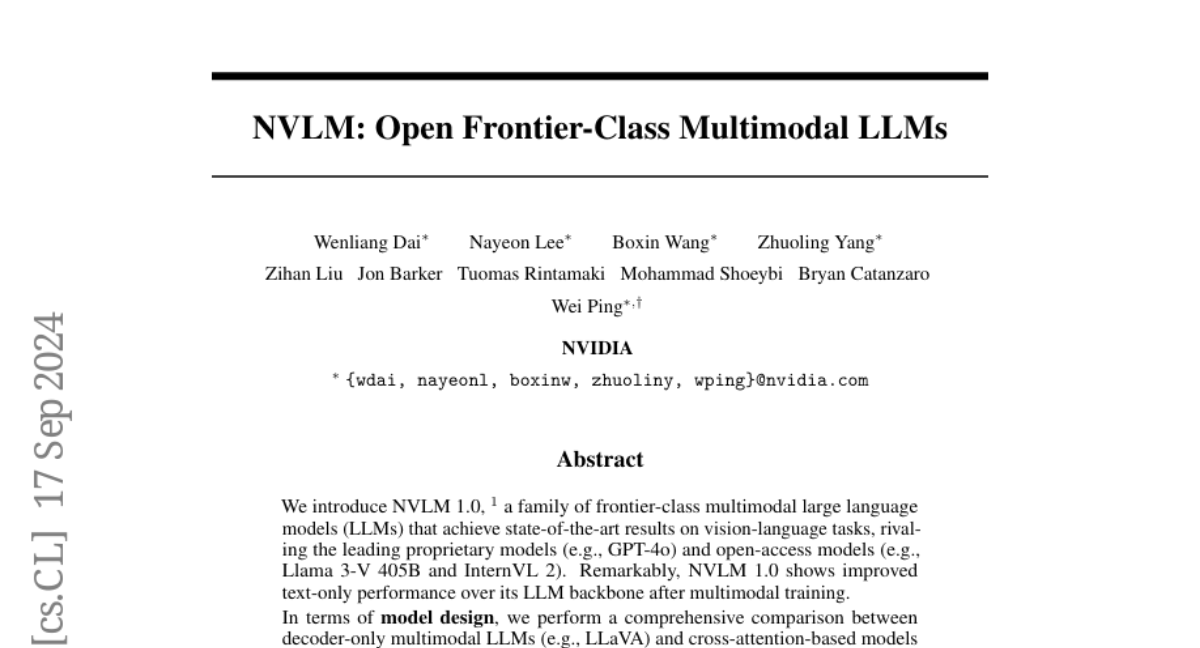
Summary
This paper introduces NVLM 1.0, a new type of multimodal large language model (LLM) that excels in both understanding text and images, achieving top performance in various tasks.
What's the problem?
Many existing models struggle to effectively combine text and image understanding, often losing performance in one area when trained on both. This makes it difficult for them to handle complex tasks that require a deep understanding of both types of data, especially when they need to maintain strong performance in text-only tasks.
What's the solution?
NVLM 1.0 addresses this issue by using a novel architecture that integrates the strengths of different model types. It can generate and understand content from both text and images without sacrificing performance in either area. The model has been trained with high-quality datasets that enhance its ability to perform tasks like mathematical reasoning and coding based on visual information. Additionally, it uses a unique tile-tagging design for processing high-resolution images, which improves its performance on tasks like optical character recognition (OCR).
Why it matters?
This research is significant because it represents a major advancement in creating models that can effectively understand and generate content across different modalities. By maintaining and even improving text-only performance while excelling in vision-language tasks, NVLM 1.0 has the potential to enhance applications in fields such as education, content creation, and AI-driven tools for various industries.
Abstract
We introduce NVLM 1.0, a family of frontier-class multimodal large language models (LLMs) that achieve state-of-the-art results on vision-language tasks, rivaling the leading proprietary models (e.g., GPT-4o) and open-access models (e.g., Llama 3-V 405B and InternVL 2). Remarkably, NVLM 1.0 shows improved text-only performance over its LLM backbone after multimodal training. In terms of model design, we perform a comprehensive comparison between decoder-only multimodal LLMs (e.g., LLaVA) and cross-attention-based models (e.g., Flamingo). Based on the strengths and weaknesses of both approaches, we propose a novel architecture that enhances both training efficiency and multimodal reasoning capabilities. Furthermore, we introduce a 1-D tile-tagging design for tile-based dynamic high-resolution images, which significantly boosts performance on multimodal reasoning and OCR-related tasks. Regarding training data, we meticulously curate and provide detailed information on our multimodal pretraining and supervised fine-tuning datasets. Our findings indicate that dataset quality and task diversity are more important than scale, even during the pretraining phase, across all architectures. Notably, we develop production-grade multimodality for the NVLM-1.0 models, enabling them to excel in vision-language tasks while maintaining and even improving text-only performance compared to their LLM backbones. To achieve this, we craft and integrate a high-quality text-only dataset into multimodal training, alongside a substantial amount of multimodal math and reasoning data, leading to enhanced math and coding capabilities across modalities. To advance research in the field, we are releasing the model weights and will open-source the code for the community: https://nvlm-project.github.io/.