ObjCtrl-2.5D: Training-free Object Control with Camera Poses
Zhouxia Wang, Yushi Lan, Shangchen Zhou, Chen Change Loy
2024-12-11
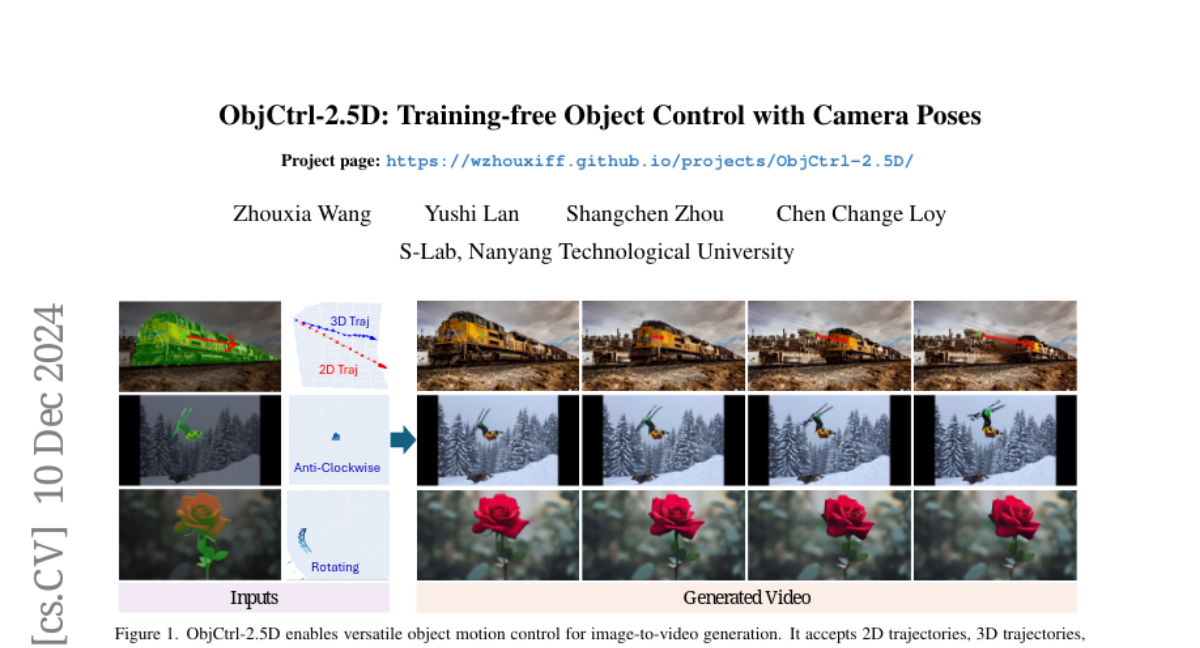
Summary
This paper talks about ObjCtrl-2.5D, a new method that allows for better control of objects in videos by using 3D trajectories instead of the traditional 2D ones.
What's the problem?
Current methods for controlling how objects move in videos often rely on 2D paths, which can lead to unnatural movements and don’t always reflect what the user wants. These methods struggle to accurately capture the depth and positioning of objects, making it hard to create realistic video content.
What's the solution?
The authors introduce ObjCtrl-2.5D, which uses a training-free approach to control object movements through 3D trajectories that include depth information. By treating object movement like camera movement, they represent these 3D paths as sequences of camera poses. This allows them to use an existing model for camera motion control without needing additional training. They also added a module to separate the target object from the background, enabling more precise control over how the object moves independently.
Why it matters?
This research is important because it significantly improves how objects can be controlled in video generation, leading to more realistic and customizable animations. By allowing for complex movements like rotation and better alignment with user intentions, ObjCtrl-2.5D enhances creative possibilities in fields like animation, gaming, and visual effects.
Abstract
This study aims to achieve more precise and versatile object control in image-to-video (I2V) generation. Current methods typically represent the spatial movement of target objects with 2D trajectories, which often fail to capture user intention and frequently produce unnatural results. To enhance control, we present ObjCtrl-2.5D, a training-free object control approach that uses a 3D trajectory, extended from a 2D trajectory with depth information, as a control signal. By modeling object movement as camera movement, ObjCtrl-2.5D represents the 3D trajectory as a sequence of camera poses, enabling object motion control using an existing camera motion control I2V generation model (CMC-I2V) without training. To adapt the CMC-I2V model originally designed for global motion control to handle local object motion, we introduce a module to isolate the target object from the background, enabling independent local control. In addition, we devise an effective way to achieve more accurate object control by sharing low-frequency warped latent within the object's region across frames. Extensive experiments demonstrate that ObjCtrl-2.5D significantly improves object control accuracy compared to training-free methods and offers more diverse control capabilities than training-based approaches using 2D trajectories, enabling complex effects like object rotation. Code and results are available at https://wzhouxiff.github.io/projects/ObjCtrl-2.5D/.