ObjectMate: A Recurrence Prior for Object Insertion and Subject-Driven Generation
Daniel Winter, Asaf Shul, Matan Cohen, Dana Berman, Yael Pritch, Alex Rav-Acha, Yedid Hoshen
2024-12-16
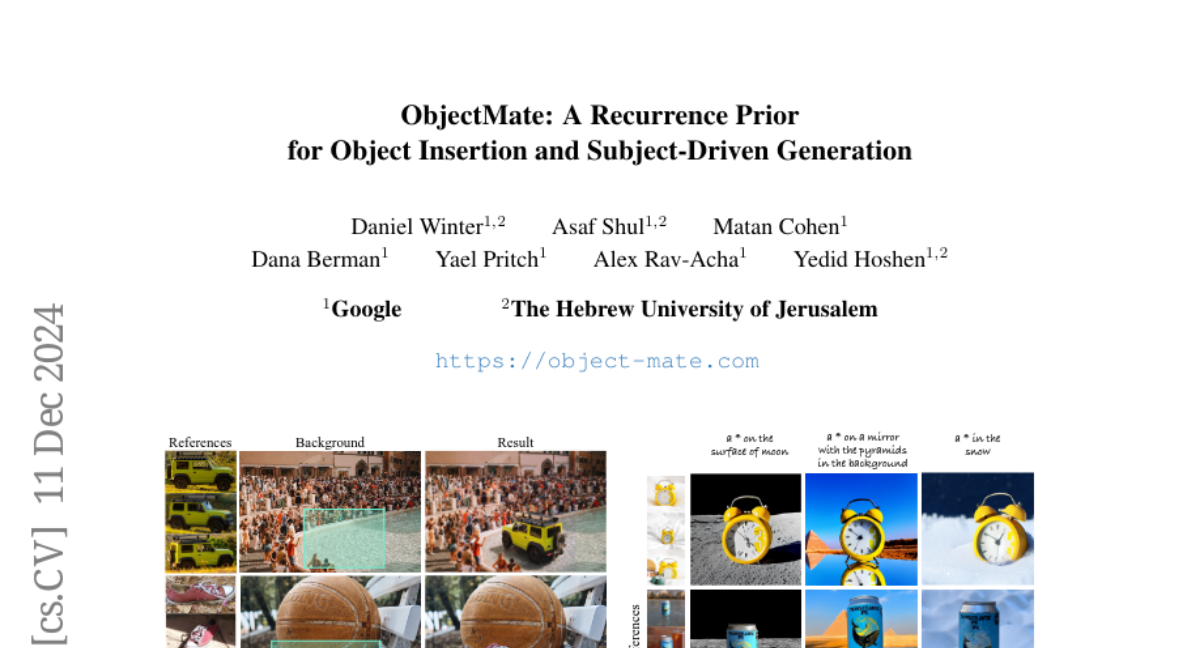
Summary
This paper talks about ObjectMate, a new method that helps computers insert objects into scenes and generate images based on specific subjects more accurately and realistically.
What's the problem?
Current methods for inserting objects into images often struggle to make the objects look natural in terms of lighting and position. They also have difficulty keeping the identity of the object consistent across different views. This can lead to unrealistic images that don’t match the original scene well.
What's the solution?
ObjectMate solves these problems by using a unique approach that takes advantage of the fact that many common objects appear in various images with different poses and lighting. It gathers diverse views of these objects from large datasets, allowing it to create a rich dataset for training. This dataset helps the model learn how to accurately compose objects into scenes while preserving their identity. ObjectMate uses a straightforward architecture to map descriptions of the scene and object to create high-quality composite images without needing complex adjustments during use.
Why it matters?
This research is significant because it improves how AI can create realistic images by seamlessly integrating objects into scenes. This has practical applications in fields like graphic design, video game development, and virtual reality, where creating believable visual content is essential.
Abstract
This paper introduces a tuning-free method for both object insertion and subject-driven generation. The task involves composing an object, given multiple views, into a scene specified by either an image or text. Existing methods struggle to fully meet the task's challenging objectives: (i) seamlessly composing the object into the scene with photorealistic pose and lighting, and (ii) preserving the object's identity. We hypothesize that achieving these goals requires large scale supervision, but manually collecting sufficient data is simply too expensive. The key observation in this paper is that many mass-produced objects recur across multiple images of large unlabeled datasets, in different scenes, poses, and lighting conditions. We use this observation to create massive supervision by retrieving sets of diverse views of the same object. This powerful paired dataset enables us to train a straightforward text-to-image diffusion architecture to map the object and scene descriptions to the composited image. We compare our method, ObjectMate, with state-of-the-art methods for object insertion and subject-driven generation, using a single or multiple references. Empirically, ObjectMate achieves superior identity preservation and more photorealistic composition. Differently from many other multi-reference methods, ObjectMate does not require slow test-time tuning.