OD-VAE: An Omni-dimensional Video Compressor for Improving Latent Video Diffusion Model
Liuhan Chen, Zongjian Li, Bin Lin, Bin Zhu, Qian Wang, Shenghai Yuan, Xing Zhou, Xinghua Cheng, Li Yuan
2024-09-04
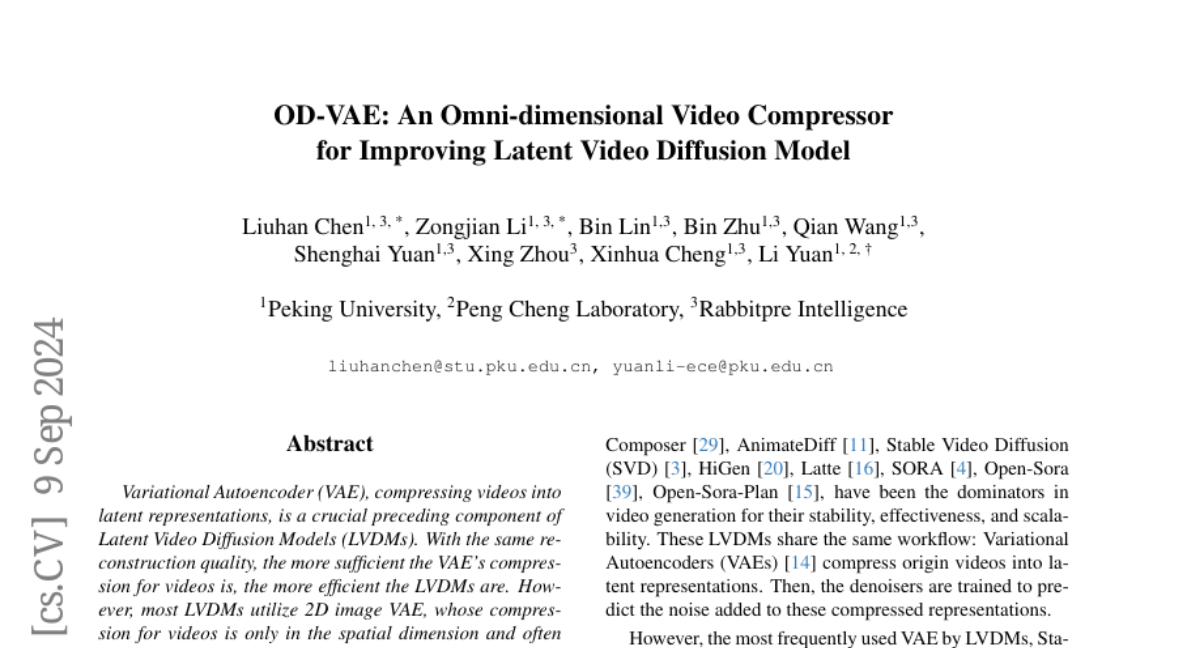
Summary
This paper talks about OD-VAE, a new type of video compressor that improves how videos are processed for better quality and efficiency in video generation models.
What's the problem?
Current methods for compressing videos often only focus on the visual aspects (like what you see) and ignore how the video changes over time (like motion). This limitation makes it difficult to create high-quality videos from compressed data, especially when using models designed to generate videos.
What's the solution?
OD-VAE introduces a new way to compress videos by considering both the visual and temporal aspects. It compresses videos in two dimensions: spatial (the picture itself) and temporal (the changes over time). The researchers designed four different versions of OD-VAE to find the best balance between video quality and how quickly it can process the data. They also created new strategies for training the model efficiently so it can handle long videos without needing too much computer memory.
Why it matters?
This research is important because it allows for better video generation by making the compression process more effective. By improving how videos are compressed and reconstructed, OD-VAE can enhance applications in entertainment, virtual reality, and other fields where high-quality video is essential.
Abstract
Variational Autoencoder (VAE), compressing videos into latent representations, is a crucial preceding component of Latent Video Diffusion Models (LVDMs). With the same reconstruction quality, the more sufficient the VAE's compression for videos is, the more efficient the LVDMs are. However, most LVDMs utilize 2D image VAE, whose compression for videos is only in the spatial dimension and often ignored in the temporal dimension. How to conduct temporal compression for videos in a VAE to obtain more concise latent representations while promising accurate reconstruction is seldom explored. To fill this gap, we propose an omni-dimension compression VAE, named OD-VAE, which can temporally and spatially compress videos. Although OD-VAE's more sufficient compression brings a great challenge to video reconstruction, it can still achieve high reconstructed accuracy by our fine design. To obtain a better trade-off between video reconstruction quality and compression speed, four variants of OD-VAE are introduced and analyzed. In addition, a novel tail initialization is designed to train OD-VAE more efficiently, and a novel inference strategy is proposed to enable OD-VAE to handle videos of arbitrary length with limited GPU memory. Comprehensive experiments on video reconstruction and LVDM-based video generation demonstrate the effectiveness and efficiency of our proposed methods.