Old Optimizer, New Norm: An Anthology
Jeremy Bernstein, Laker Newhouse
2024-10-03
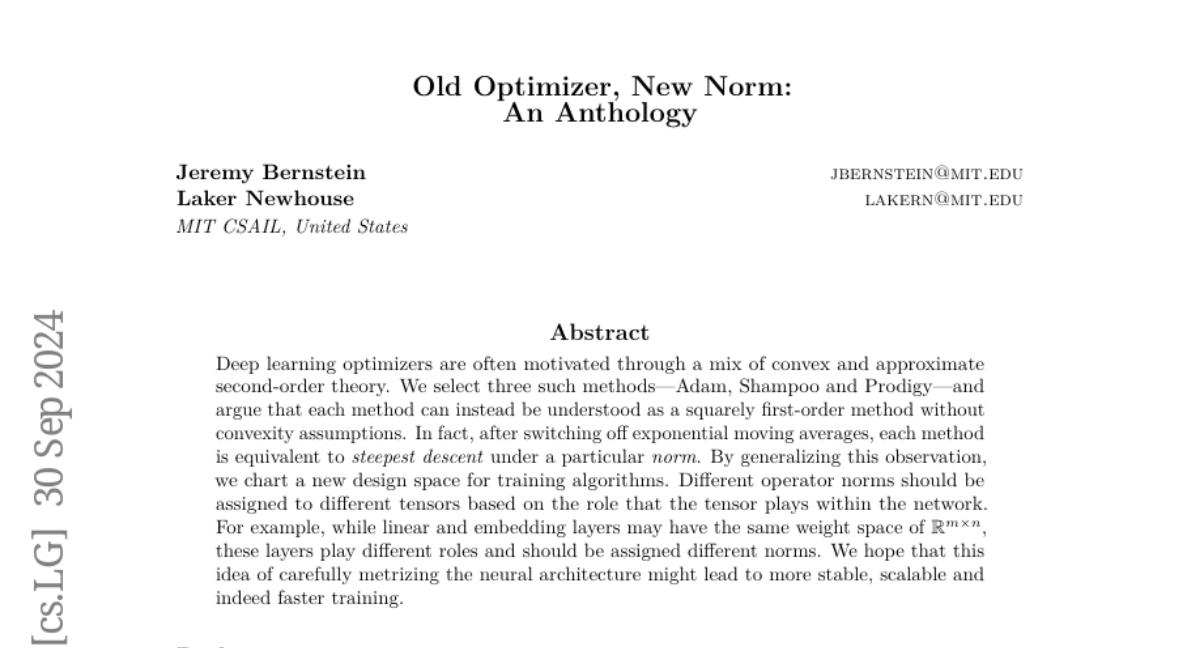
Summary
This paper discusses how to improve deep learning optimizers by understanding them better and proposing new ways to design them for more effective training of neural networks.
What's the problem?
Deep learning optimizers, which help train AI models by adjusting their parameters to minimize errors, often rely on complex theories that assume certain conditions (like convexity). However, many popular optimizers, such as Adam and Shampoo, can be seen as simpler methods that don't need these assumptions. This misunderstanding can lead to inefficiencies and suboptimal performance during training.
What's the solution?
The authors argue that by turning off certain features of these optimizers, they can be viewed as basic first-order methods that follow the steepest descent direction. They propose a new approach where different mathematical norms (ways of measuring distances and differences) are applied to different parts of the model based on their roles. This means that layers in the neural network that serve different functions will be treated differently during training, potentially leading to faster and more stable training processes.
Why it matters?
This research is important because it opens up new possibilities for designing more effective training algorithms for AI models. By better understanding how optimizers work and adapting them to the specific needs of different parts of a neural network, we can improve the efficiency and performance of deep learning systems, making them more capable in various applications such as image recognition, natural language processing, and more.
Abstract
Deep learning optimizers are often motivated through a mix of convex and approximate second-order theory. We select three such methods -- Adam, Shampoo and Prodigy -- and argue that each method can instead be understood as a squarely first-order method without convexity assumptions. In fact, after switching off exponential moving averages, each method is equivalent to steepest descent under a particular norm. By generalizing this observation, we chart a new design space for training algorithms. Different operator norms should be assigned to different tensors based on the role that the tensor plays within the network. For example, while linear and embedding layers may have the same weight space of R^{mtimes n}, these layers play different roles and should be assigned different norms. We hope that this idea of carefully metrizing the neural architecture might lead to more stable, scalable and indeed faster training.