OlympicArena: Benchmarking Multi-discipline Cognitive Reasoning for Superintelligent AI
Zhen Huang, Zengzhi Wang, Shijie Xia, Xuefeng Li, Haoyang Zou, Ruijie Xu, Run-Ze Fan, Lyumanshan Ye, Ethan Chern, Yixin Ye, Yikai Zhang, Yuqing Yang, Ting Wu, Binjie Wang, Shichao Sun, Yang Xiao, Yiyuan Li, Fan Zhou, Steffi Chern, Yiwei Qin, Yan Ma, Jiadi Su
2024-06-19
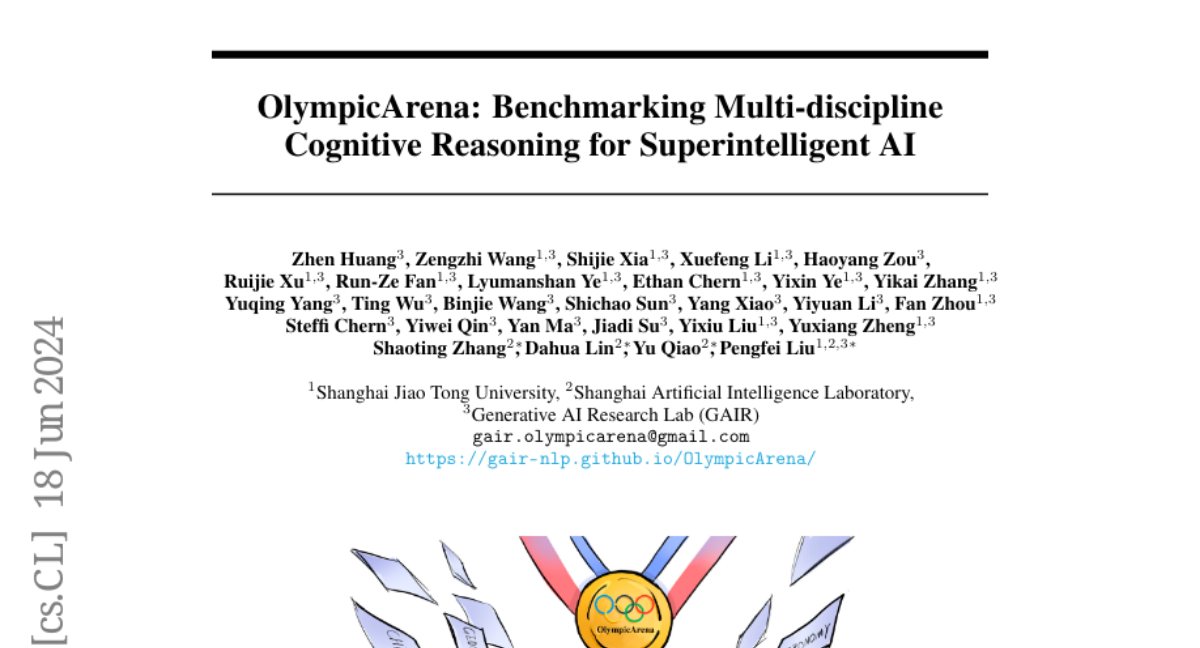
Summary
This paper introduces OlympicArena, a new benchmark designed to test how well artificial intelligence (AI) models can reason and solve problems across various disciplines. It includes a large set of problems that challenge these models in both text and images, aiming to evaluate their cognitive reasoning abilities.
What's the problem?
As AI technology has advanced, especially with large language models (LLMs) and multimodal models (LMMs), there is a growing need to assess how well these systems can think and solve complex problems. Current benchmarks often focus on narrow tasks or single types of input, which does not reflect the real-world challenges AI will face. This lack of comprehensive testing makes it hard to understand the true capabilities and limitations of these models.
What's the solution?
To address this issue, the authors created OlympicArena, which features 11,163 bilingual problems from seven different fields, including mathematics, physics, chemistry, biology, geography, astronomy, and computer science. These problems are designed to mimic the complexity of Olympic-level challenges and include both text-only and text-image formats. The benchmark not only measures how accurately the models can answer questions but also evaluates their reasoning processes. The authors found that even advanced models like GPT-4o struggled with these complex tasks, achieving only about 39.97% accuracy overall.
Why it matters?
This research is important because it provides a rigorous way to evaluate AI's cognitive reasoning skills across multiple disciplines. By using OlympicArena as a testing ground, researchers can better understand where AI models excel and where they need improvement. This could lead to the development of more capable AI systems that can tackle complex scientific problems and contribute to advancements in various fields.
Abstract
The evolution of Artificial Intelligence (AI) has been significantly accelerated by advancements in Large Language Models (LLMs) and Large Multimodal Models (LMMs), gradually showcasing potential cognitive reasoning abilities in problem-solving and scientific discovery (i.e., AI4Science) once exclusive to human intellect. To comprehensively evaluate current models' performance in cognitive reasoning abilities, we introduce OlympicArena, which includes 11,163 bilingual problems across both text-only and interleaved text-image modalities. These challenges encompass a wide range of disciplines spanning seven fields and 62 international Olympic competitions, rigorously examined for data leakage. We argue that the challenges in Olympic competition problems are ideal for evaluating AI's cognitive reasoning due to their complexity and interdisciplinary nature, which are essential for tackling complex scientific challenges and facilitating discoveries. Beyond evaluating performance across various disciplines using answer-only criteria, we conduct detailed experiments and analyses from multiple perspectives. We delve into the models' cognitive reasoning abilities, their performance across different modalities, and their outcomes in process-level evaluations, which are vital for tasks requiring complex reasoning with lengthy solutions. Our extensive evaluations reveal that even advanced models like GPT-4o only achieve a 39.97% overall accuracy, illustrating current AI limitations in complex reasoning and multimodal integration. Through the OlympicArena, we aim to advance AI towards superintelligence, equipping it to address more complex challenges in science and beyond. We also provide a comprehensive set of resources to support AI research, including a benchmark dataset, an open-source annotation platform, a detailed evaluation tool, and a leaderboard with automatic submission features.