OmniBind: Large-scale Omni Multimodal Representation via Binding Spaces
Zehan Wang, Ziang Zhang, Hang Zhang, Luping Liu, Rongjie Huang, Xize Cheng, Hengshuang Zhao, Zhou Zhao
2024-07-17
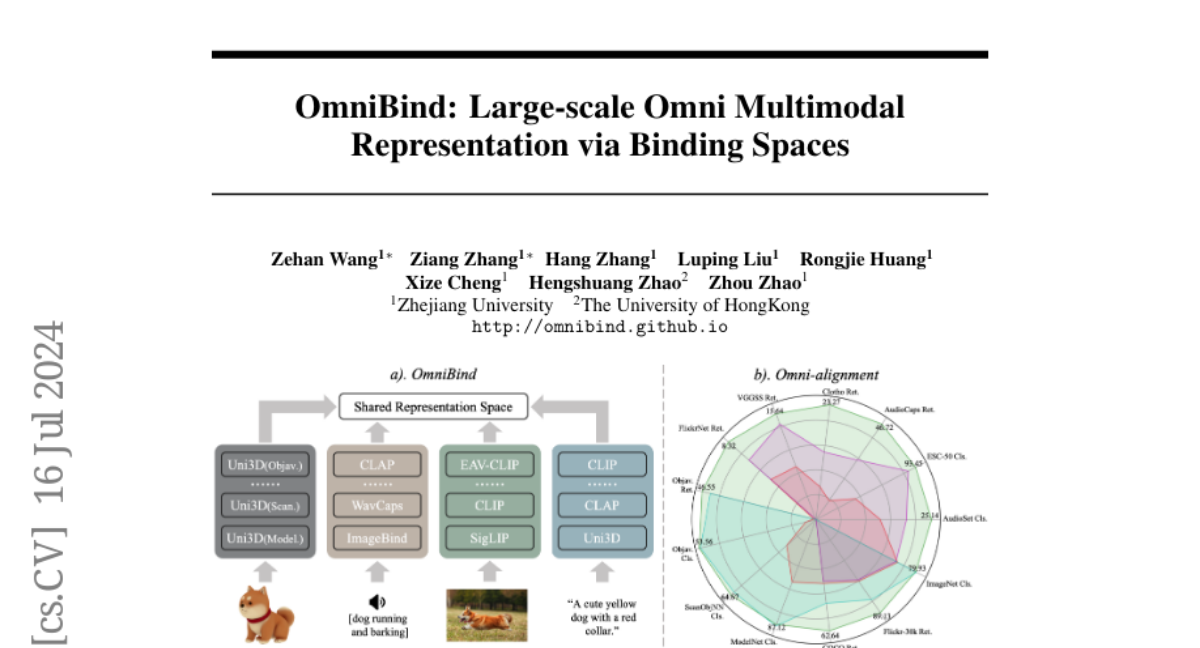
Summary
This paper introduces OmniBind, a new framework for creating a large-scale multimodal representation that can understand and generate information from various sources like text, images, audio, and 3D models.
What's the problem?
Combining different types of information (like text, images, and sounds) into one cohesive understanding is challenging. Existing methods often require a lot of data and can be inefficient, making it hard to process complex multimodal information effectively. This limits the ability of AI systems to interact with users in a meaningful way.
What's the solution?
OmniBind solves this problem by using a technique called 'binding spaces' to connect various pre-trained models that specialize in different types of data. Instead of starting from scratch, it remaps and combines these models to create a unified representation. The system dynamically assigns weights to different types of data to ensure they work well together. This approach allows OmniBind to learn efficiently using less data and less computing power while still achieving high performance across multiple tasks.
Why it matters?
This research is significant because it enables AI systems to better understand and generate responses based on diverse types of information. By improving how different modalities are integrated, OmniBind can enhance applications like virtual assistants, intelligent search engines, and any technology that requires understanding complex inputs from various sources. This could lead to more advanced human-computer interactions in the future.
Abstract
Recently, human-computer interaction with various modalities has shown promising applications, like GPT-4o and Gemini. Given the foundational role of multimodal joint representation in understanding and generation pipelines, high-quality omni joint representations would be a step toward co-processing more diverse multimodal information. In this work, we present OmniBind, large-scale multimodal joint representation models ranging in scale from 7 billion to 30 billion parameters, which support 3D, audio, image, and language inputs. Due to the scarcity of data pairs across all modalities, instead of training large models from scratch, we propose remapping and binding the spaces of various pre-trained specialist models together. This approach enables "scaling up" by indirectly increasing the model parameters and the amount of seen data. To effectively integrate various spaces, we dynamically assign weights to different spaces by learning routers with two objectives: cross-modal overall alignment and language representation decoupling. Notably, since binding and routing spaces both only require lightweight networks, OmniBind is extremely training-efficient. Learning the largest 30B model requires merely unpaired unimodal data and approximately 3 days on a single 8-4090 node. Extensive experiments demonstrate the versatility and superiority of OmniBind as an omni representation model, highlighting its great potential for diverse applications, such as any-query and composable multimodal understanding.