OmniBooth: Learning Latent Control for Image Synthesis with Multi-modal Instruction
Leheng Li, Weichao Qiu, Xu Yan, Jing He, Kaiqiang Zhou, Yingjie Cai, Qing Lian, Bingbing Liu, Ying-Cong Chen
2024-10-08
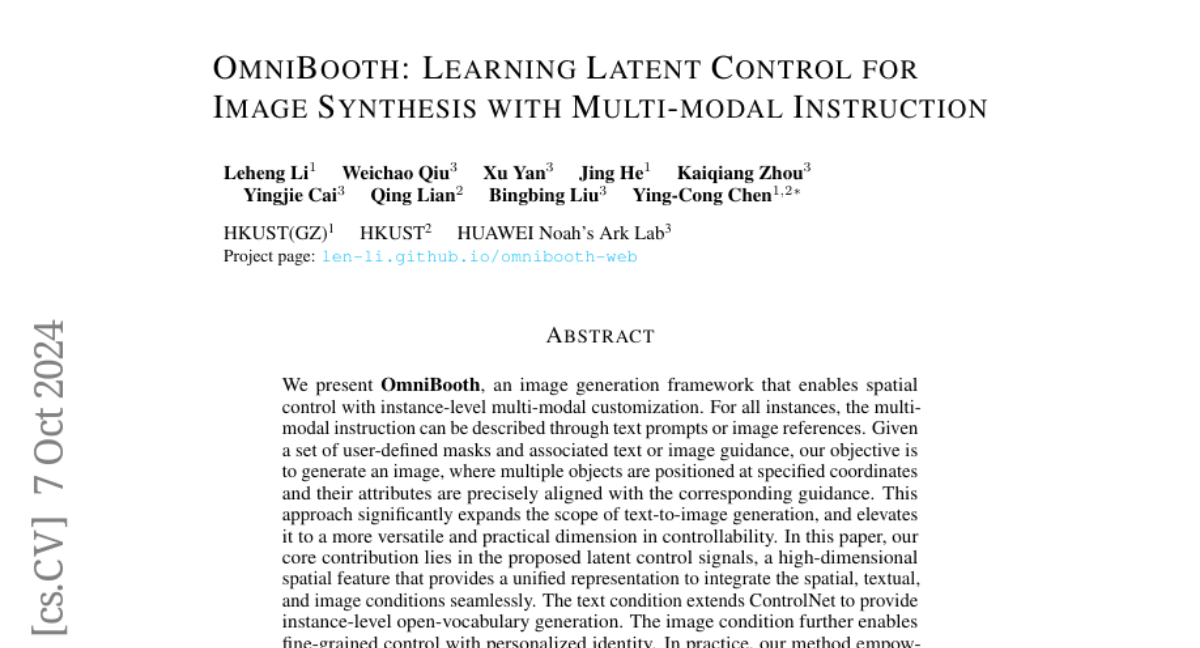
Summary
This paper presents OmniBooth, a new framework for generating images that allows users to control the placement and appearance of multiple objects based on text prompts or image references.
What's the problem?
In traditional image generation methods, it can be difficult to create images with precise control over where objects are placed and how they look. Existing models often struggle to integrate different types of input (like text and images) effectively, leading to less accurate or desirable results in the generated images.
What's the solution?
OmniBooth addresses this problem by using a technique called latent control signals, which helps the model understand and combine spatial, textual, and visual information more effectively. Users can provide specific instructions about where to place objects and how they should appear using either text or images. The framework allows for greater flexibility and control in generating images, making it easier for users to achieve their desired outcomes. The authors conducted experiments that showed OmniBooth performs better in creating high-quality images that match user specifications compared to previous models.
Why it matters?
This research is important because it enhances the capabilities of image generation technologies, making them more user-friendly and versatile. By allowing for precise control over image creation, OmniBooth can be useful in various fields such as graphic design, video game development, and virtual reality, where accurate representation of objects is crucial.
Abstract
We present OmniBooth, an image generation framework that enables spatial control with instance-level multi-modal customization. For all instances, the multimodal instruction can be described through text prompts or image references. Given a set of user-defined masks and associated text or image guidance, our objective is to generate an image, where multiple objects are positioned at specified coordinates and their attributes are precisely aligned with the corresponding guidance. This approach significantly expands the scope of text-to-image generation, and elevates it to a more versatile and practical dimension in controllability. In this paper, our core contribution lies in the proposed latent control signals, a high-dimensional spatial feature that provides a unified representation to integrate the spatial, textual, and image conditions seamlessly. The text condition extends ControlNet to provide instance-level open-vocabulary generation. The image condition further enables fine-grained control with personalized identity. In practice, our method empowers users with more flexibility in controllable generation, as users can choose multi-modal conditions from text or images as needed. Furthermore, thorough experiments demonstrate our enhanced performance in image synthesis fidelity and alignment across different tasks and datasets. Project page: https://len-li.github.io/omnibooth-web/