OmniEdit: Building Image Editing Generalist Models Through Specialist Supervision
Cong Wei, Zheyang Xiong, Weiming Ren, Xinrun Du, Ge Zhang, Wenhu Chen
2024-11-12
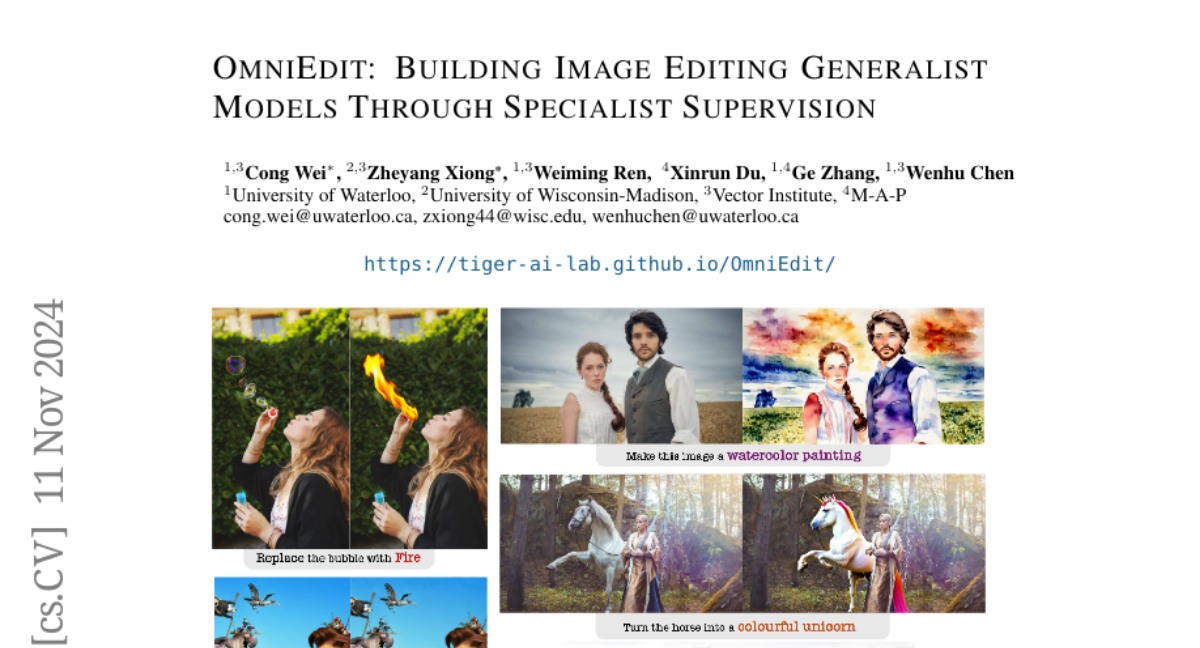
Summary
This paper presents OmniEdit, a new image editing model that can handle multiple editing tasks using guidance from various specialized models, improving the quality and versatility of image editing.
What's the problem?
Current image editing methods often face challenges that limit their effectiveness in real-world applications. These include having limited editing skills due to biased training data, dealing with noisy datasets filled with artifacts, and being restricted to low-resolution images with fixed sizes. This makes it hard for models to perform well when faced with diverse and complex editing tasks.
What's the solution?
OmniEdit addresses these issues by using a combination of seven specialized models that provide guidance for different editing tasks. It employs a new method called importance sampling to improve the quality of the training data, moving away from simpler filtering techniques that don't work as well. Additionally, it introduces a new architecture called EditNet that enhances the model's ability to edit images successfully. OmniEdit is designed to work with images of any size and aspect ratio, making it more adaptable for real-world use. The authors tested OmniEdit against existing models and found that it significantly outperformed them in both automated and human evaluations.
Why it matters?
This research is important because it creates a more powerful and flexible tool for image editing that can be used in various applications, from graphic design to photography. By improving how models learn from specialized tasks and handle different image formats, OmniEdit can lead to higher quality edits and better user experiences in creative fields.
Abstract
Instruction-guided image editing methods have demonstrated significant potential by training diffusion models on automatically synthesized or manually annotated image editing pairs. However, these methods remain far from practical, real-life applications. We identify three primary challenges contributing to this gap. Firstly, existing models have limited editing skills due to the biased synthesis process. Secondly, these methods are trained with datasets with a high volume of noise and artifacts. This is due to the application of simple filtering methods like CLIP-score. Thirdly, all these datasets are restricted to a single low resolution and fixed aspect ratio, limiting the versatility to handle real-world use cases. In this paper, we present \omniedit, which is an omnipotent editor to handle seven different image editing tasks with any aspect ratio seamlessly. Our contribution is in four folds: (1) \omniedit is trained by utilizing the supervision from seven different specialist models to ensure task coverage. (2) we utilize importance sampling based on the scores provided by large multimodal models (like GPT-4o) instead of CLIP-score to improve the data quality. (3) we propose a new editing architecture called EditNet to greatly boost the editing success rate, (4) we provide images with different aspect ratios to ensure that our model can handle any image in the wild. We have curated a test set containing images of different aspect ratios, accompanied by diverse instructions to cover different tasks. Both automatic evaluation and human evaluations demonstrate that \omniedit can significantly outperform all the existing models. Our code, dataset and model will be available at https://tiger-ai-lab.github.io/OmniEdit/