OmniGen: Unified Image Generation
Shitao Xiao, Yueze Wang, Junjie Zhou, Huaying Yuan, Xingrun Xing, Ruiran Yan, Shuting Wang, Tiejun Huang, Zheng Liu
2024-09-18
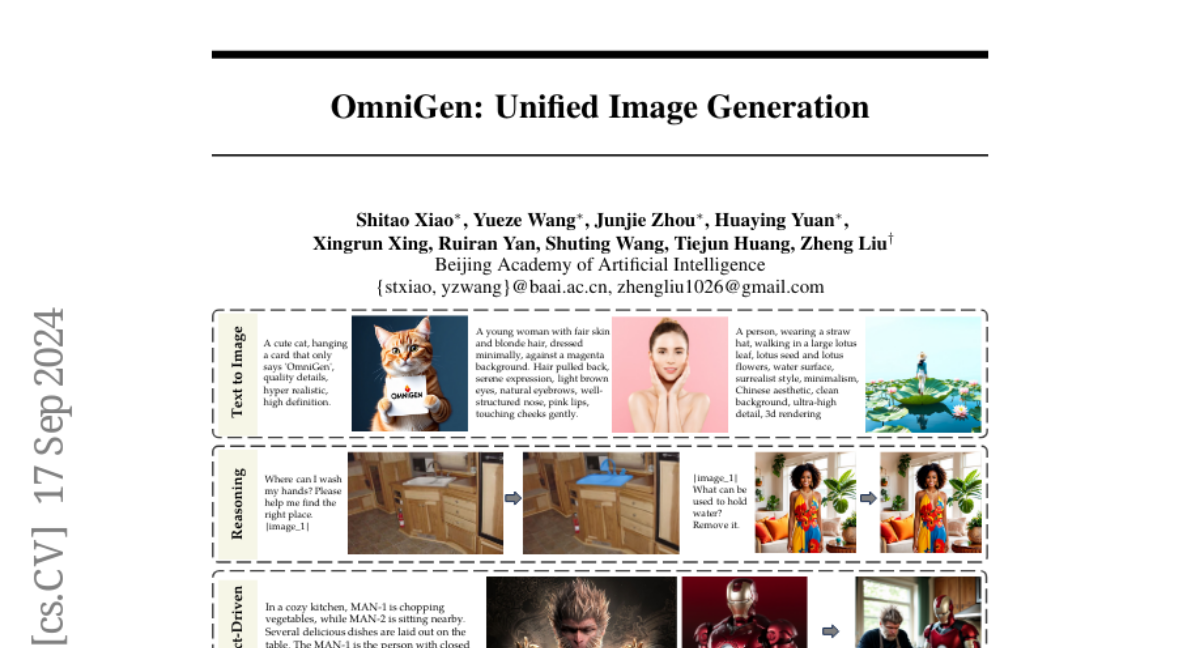
Summary
This paper introduces OmniGen, a new model for generating images that simplifies the process by combining various image generation tasks into one unified framework.
What's the problem?
Existing image generation models, like Stable Diffusion, often require multiple additional tools or modules to handle different tasks, such as generating images from text or editing images. This can make the process complicated and less user-friendly, especially for people who want to perform various tasks without needing to set up multiple systems.
What's the solution?
OmniGen solves this problem by integrating all these capabilities into a single model. It can generate images from text, edit images, and even perform traditional computer vision tasks like detecting edges or recognizing human poses. The model is designed to be simpler and more efficient, allowing users to complete complex tasks just by giving instructions without needing extra steps or tools. Additionally, it learns in a way that allows it to apply knowledge across different tasks, making it adaptable and powerful.
Why it matters?
This research is important because it represents a significant step towards creating a general-purpose image generation model that can handle various tasks effectively. By making image generation easier and more versatile, OmniGen has the potential to enhance applications in areas like graphic design, virtual reality, and content creation, making advanced image processing accessible to more users.
Abstract
In this work, we introduce OmniGen, a new diffusion model for unified image generation. Unlike popular diffusion models (e.g., Stable Diffusion), OmniGen no longer requires additional modules such as ControlNet or IP-Adapter to process diverse control conditions. OmniGenis characterized by the following features: 1) Unification: OmniGen not only demonstrates text-to-image generation capabilities but also inherently supports other downstream tasks, such as image editing, subject-driven generation, and visual-conditional generation. Additionally, OmniGen can handle classical computer vision tasks by transforming them into image generation tasks, such as edge detection and human pose recognition. 2) Simplicity: The architecture of OmniGen is highly simplified, eliminating the need for additional text encoders. Moreover, it is more user-friendly compared to existing diffusion models, enabling complex tasks to be accomplished through instructions without the need for extra preprocessing steps (e.g., human pose estimation), thereby significantly simplifying the workflow of image generation. 3) Knowledge Transfer: Through learning in a unified format, OmniGen effectively transfers knowledge across different tasks, manages unseen tasks and domains, and exhibits novel capabilities. We also explore the model's reasoning capabilities and potential applications of chain-of-thought mechanism. This work represents the first attempt at a general-purpose image generation model, and there remain several unresolved issues. We will open-source the related resources at https://github.com/VectorSpaceLab/OmniGen to foster advancements in this field.