OmniNOCS: A unified NOCS dataset and model for 3D lifting of 2D objects
Akshay Krishnan, Abhijit Kundu, Kevis-Kokitsi Maninis, James Hays, Matthew Brown
2024-07-13
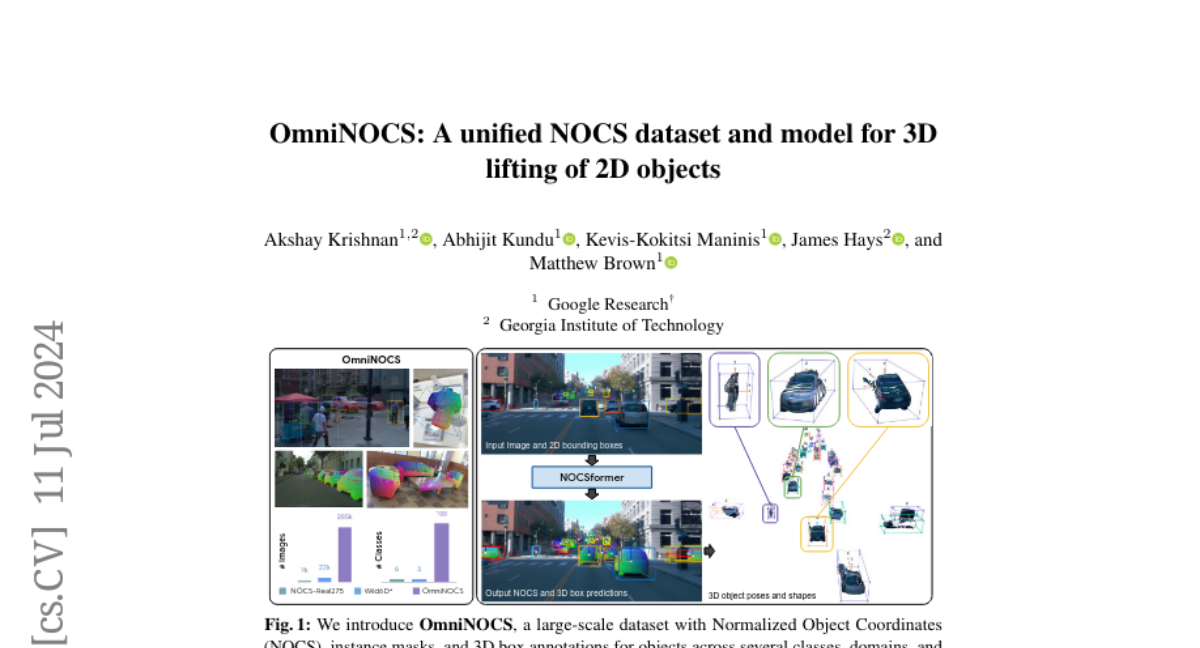
Summary
This paper introduces OmniNOCS, a new and extensive dataset designed to improve how computers understand and predict the 3D shapes and positions of objects from 2D images. It includes detailed information like object masks and 3D bounding boxes for a wide variety of indoor and outdoor scenes.
What's the problem?
Existing datasets for understanding 3D objects from 2D images are limited in size and variety, making it hard for models to learn effectively. They often contain fewer object classes and instances, which restricts the ability of AI systems to generalize their learning to new situations or different types of objects. This can lead to poor performance when trying to recognize or manipulate objects in real-world scenarios.
What's the solution?
OmniNOCS addresses these issues by providing a large-scale dataset that includes 20 times more object classes and 200 times more instances than previous datasets. The authors also developed a new model called NOCSformer, which uses advanced transformer technology to predict the 3D shapes, masks, and poses of objects based on 2D detections. This model is unique because it can work with a wide range of object categories and generalize well to new datasets.
Why it matters?
This research is important because it enhances the ability of AI systems to accurately interpret and interact with the physical world by improving how they understand 3D objects from images. By providing a comprehensive dataset and an effective model, OmniNOCS can help advance fields like robotics, augmented reality, and autonomous vehicles, where understanding the shape and position of objects is crucial.
Abstract
We propose OmniNOCS, a large-scale monocular dataset with 3D Normalized Object Coordinate Space (NOCS) maps, object masks, and 3D bounding box annotations for indoor and outdoor scenes. OmniNOCS has 20 times more object classes and 200 times more instances than existing NOCS datasets (NOCS-Real275, Wild6D). We use OmniNOCS to train a novel, transformer-based monocular NOCS prediction model (NOCSformer) that can predict accurate NOCS, instance masks and poses from 2D object detections across diverse classes. It is the first NOCS model that can generalize to a broad range of classes when prompted with 2D boxes. We evaluate our model on the task of 3D oriented bounding box prediction, where it achieves comparable results to state-of-the-art 3D detection methods such as Cube R-CNN. Unlike other 3D detection methods, our model also provides detailed and accurate 3D object shape and segmentation. We propose a novel benchmark for the task of NOCS prediction based on OmniNOCS, which we hope will serve as a useful baseline for future work in this area. Our dataset and code will be at the project website: https://omninocs.github.io.